Article • Neuro-oncology
Challenges in brain tumour segmentation
Neuroradiologist Dr Sofie Van Cauter described the challenges to brain tumour image segmentation during the European Society of Medical Imaging Informatics (EuSoMII) annual meeting in Valencia. She also outlined how, when clinically validated, AI could help tackle such problems.
Report: Mélisande Rouger
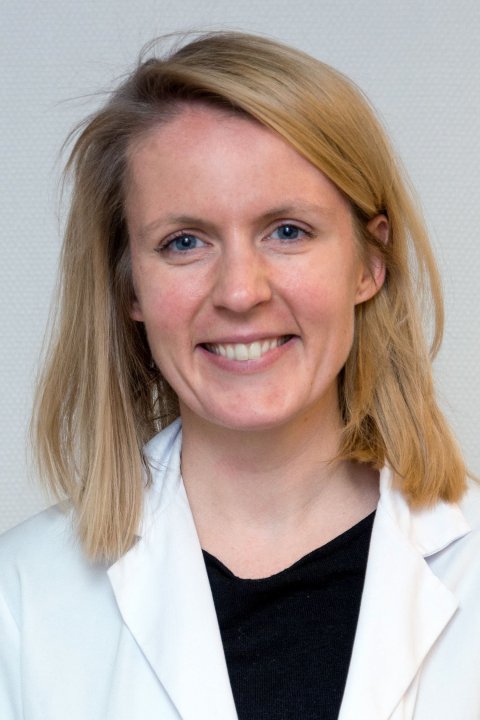
The WHO classification of brain tumours has come a long way since first introduced in 1979. The 2016 classification was something quite revolutionary, neuroradiologist Dr Sofie Van Cauter explained during the EuSoMII annual meeting. ‘For the first time, molecular diagnostics were taken into account in the diagnosis of brain tumours, leading to more detailed diagnoses and better reflecting the aggressiveness of the lesion and the patient’s prognosis and response to therapy,’ she said. ‘This has been made possible with the evolution of next generation sequencing.’
In her talk, Van Cauter focused on primary brain tumours and especially gliomas, which are the most common type of brain tumours and come in different histological and molecular subtypes. In a first step, the pathologist determines the histological subtype and a grade of aggressiveness – the classic, and still quite subjective, way to diagnosis. In the second step, as proposed by the WHO classification, several genetic mutations can be determined, leading to a more detailed final diagnosis.
Molecular diagnostics
‘We know, since we applied molecular diagnostics, that we are much more able to predict the patient’s prognosis and response to therapy. For example, in certain lesions a more benign histological subtype can initially be considered. However, if the lesion has a certain gene mutation, we know the lesion will have an aggressive course and the patient’s prognosis is not good,’ Van Cauter explained.
When dealing with a brain tumour, radiologists look at multiple MRI sequences performed in multimodal imaging protocol. In recent years, neuro-oncology research has focused on advanced MRI techniques, which try to relate histological features in radiological phenotypes, such as cellularity or vascularity. In current clinical practice, the basic imaging protocol consists of T1-weighted sequences, in which anatomy can be demonstrated, T2-weighted sequences, which relate to oedema and can be a measure of cellularity. There are also FLAIR sequences, to distinguish more subtle oedema, and T1 contrast-enhanced sequences, which distinguish areas with disrupted blood-brain barrier from regions of non-disrupted blood-brain barrier. Diffusion and perfusion-weighted sequences provide additional measures, reflecting vascular proliferation and cell density.
Challenges for brain tumour diagnosis and follow-up
When a glioma is suspected, and when dealing with a brain lesion on MRI, radiologists still try to make a diagnosis according to the histological subtype. However, this diagnosis is quite subjective, which is a major issue. ‘Accurate, quantitative thresholds are not determined yet, we just base the diagnosis on qualitative, subjective features like ”low-to-moderate oedema is probably reflecting this or that type of tumour”. We still have a problem with the diagnostic accuracy to predict the histological subtype of a glioma – it’s currently moderate to low. We hardly got started with the correlation between molecular profiling and imaging,’ she pointed out.
Glioma therapy is a combination of surgery, chemo- and radiotherapy. There have been many efforts in more advanced therapy, but until now clinicians have stayed with the cornerstone of therapies established since 2005.
An even more important issue in glioma is follow-up, currently done according to the RANO criteria, which try to classify patients in a certain category, from complete response to partial response to stable disease in progression. ‘This is done, again, in a very basic way. Just to classify into four categories, you need to sum the perpendicular diameters of all enhancing lesions and compare them to the smallest measurable tumour volumes. The classification only takes into account T1-weighted sequences with contrast administration and, to a minor extent, features on T2 and FLAIR images,’ Van Cauter explained.
Thus, radiologists face many challenges in adequate diagnosis and follow-up of gliomas. Evaluation of gliomas is subjective; does not take into account robust quantitative parameters and is not adapted to the emerging evolutions in molecular diagnostics. ‘Molecular diagnosis is better suited, but is still a new and emerging field,’ she pointed out.
AI will help, but needs clinical validation
AI entered neuro-oncology a few years ago. A Chinese group notably developed an AI algorithm that determined the subtype of brain tumours much faster and more accurately compared to a group of neurologists. In radiology in general, the tasks in AI algorithms fall into three categories: detection, segmentation and classification. Segmentation algorithms consist of multiple steps. Lesions, or other tissue of interest, have to be diagnosed, delineated and finally, different tissue types must be determined. In the case of a brain tumour, solid tumour, necrotic core and oedema have to be separated from healthy tissue. The development of automatic segmentation algorithms that focus on these tasks is an enlarging field and in the last ten years, the number of publications has risen steeply.
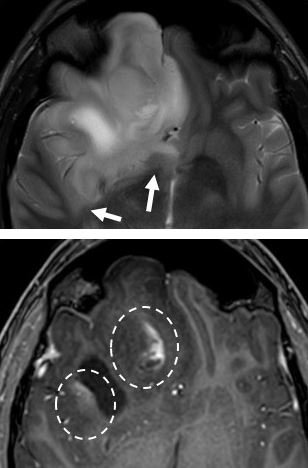
For segmentation, convolutional neural networks are used, which consist of several steps and multiple layers in a computer algorithm, using features related to symmetry, intensity gradients, etc. These characteristics are combined to classify different regions as enhancing tumour, non-enhancing tumour, necrosis or oedema. Until now, this work has stayed mainly in the field of engineering, signal and image processing. Using this kind of AI on a daily basis still requires multiple validation steps, from technical to biological and finally clinical validation. ‘Today, even starting from the beginning of the validation steps, we still have some limitations,’ she said.
The first, very important technical limitation is scarcity of data. The first general public studies on AI used data sets of 60,000 images or more, whereas in the medical field we have to work with smaller data sets – mostly between 100 and 1,000. Then, brain tumours are very heterogeneous – each is different in size, shape and location. Tumour boundaries are often unclear or irregular, with discontinuities. In addition, hospitals all have different scanners and image protocols, which complicates standardisation and data quality.
Nonetheless, there has been some progress, especially with the Brain Tumour Segmentation Challenge (BraTS), an objective evaluation of the different state-of-the-art methods that offers publicly available data sets and a community benchmark. BraTS is a data set of more than 500 cases, from different scanners, centres and imaging protocols, and also provides additional clinical data on overall survival as well as an extra assessment of uncertainty in brain tumour segmentation. ‘This has been a tremendous evolution in the field of engineering to face the challenges of the technical validation, the first step of getting brain tumour segmentation into a validated clinical product,’ Van Cauter said.
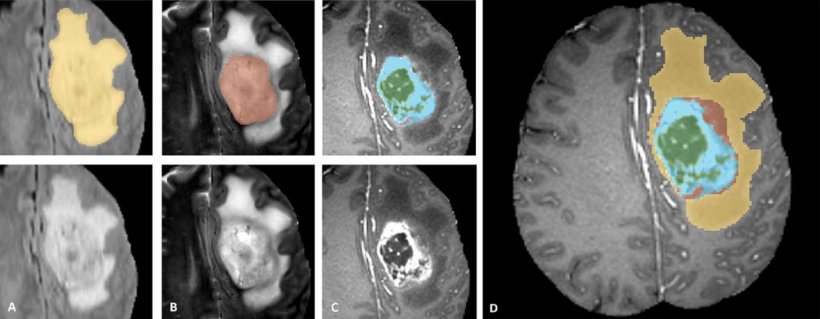
Adapted from Menze et al. IEEE 2015
Investing in interoperability, trust and safety
The next step is biological validation. ‘We still have a problem there and it mainly relates to the way engineers and medical doctors communicate and, of course, how brain tumours biologically behave. Images are not biological entities. With glioblastomas, neurosurgeons can remove everything and two months later we have a new tumour lesion. Microscopic tumour invasion cannot be captured either by imaging or by AI. When we segment tumours, an active tumour, in the language of engineers, is regarded as an enhancing tumour - and that is just not true. Lower grade tumour parts in glioblastomas are often non-enhancing,’ she added.
Clinical validation goes beyond the pure act of segmentation. The final aim is to adapt these algorithms to answer the current clinical and radiological challenges as stated, more reliably than current clinical practice. ‘If we think about segmentation,’ she said, ‘I think the targets are: stepping up to molecular diagnostics, detailed treatment planning, volumetric therapy follow-up and more detailed evaluation and early prediction of overall survival.’
AI in medical data analysis and neuro-oncology is here to stay and from the technical point of view, results are getting quite reliable. But to be introduced in the clinical setting, future investment should be in interpretability, trust and safety. ‘Some algorithms are able to make extremely accurate predictions but, from a clinical point of view, how can we trust predictions of features that we cannot understand? We need to invest more in the biological and clinical validation of these techniques,’ Van Cauter concluded.
Profile:
Dr Sofie Van Cauter is a neuroradiologist at Ziekenhuis Oost Limburg in Genk and University Hospitals Leuven, Belgium. To gain her PhD, she researched advanced MRI techniques in gliomas with an emphasis on diffusion kurtosis imaging, MR spectroscopy and perfusion techniques. This was done in collaboration with the department of Electrical Engineering of the Catholic University Leuven, where she researched deep learning techniques in diagnosis and follow-up of gliomas.
19.08.2020