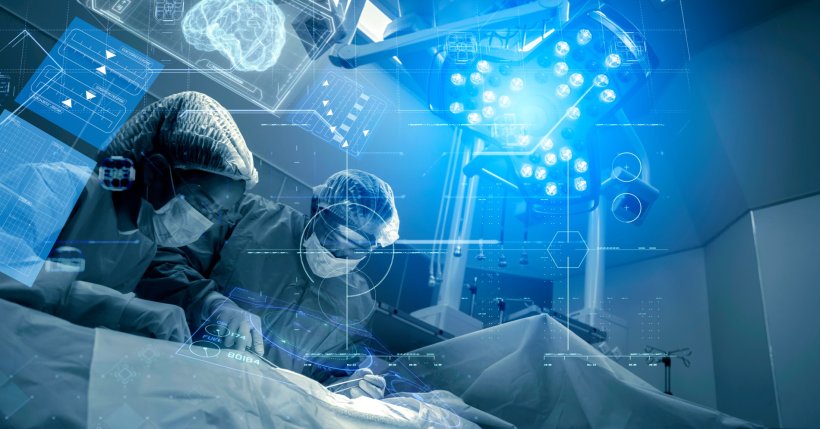
Image source: Adobe Stock/whyframeshot
Article • Assistance and decision-making systems
Artificial intelligence for preoperative planning
In surgery, artificial intelligence (AI) is applied mostly in imaging, navigation, and robotic intervention. However, AI can also play a major role in preoperative planning. Objective decisions-making, optimal utilisation of operating theatres and less overtime are additional advantages that are achieved with the use of AI in surgery.
Report: Sascha Keutel
Surgeons make complex, high-risk decisions with significant impact on patients’ lives, usually under time pressure. The multitude and variability of diagnostic data and the potential dangers of a surgical intervention pose tough challenges for the staff. Therefore, thorough pre-operative planning, in which surgeons prepare the operation based on medical records, historical methods and data, is essential for the success of a surgery.
Decision-making systems
Surgeons decide based on their training, experience, and knowledge, so their judgement or bias can influence them. Decision-making systems based on AI have the potential to overcome these limitations. These systems enable the processing of many patient data sets, which can be transferred into mathematical models and enriched with background knowledge to recommend or advise against a certain therapy.
Artificial intelligence is used as a decision-support tool with two tasks: to compensate for the different levels of knowledge and experience of surgeons by objectively and individually analysing patient data; and highlighting clinically relevant correlations that the physicians may not recognise.
For example, scientists at Heidelberg University Hospital are developing a “Cognitive Medical Assistant” (KoMed).1 The aim of the project is to recognise patterns from laboratory results, image data, diagnoses, and therapies and to identify correlations that can create individual risk profiles. The algorithm should identify a patient's surgical risk before an operation, facilitate therapy decisions and prevent complications. Specifically, the algorithm is designed to predict the likelihood that patients will need a blood transfusion.
Recommended article
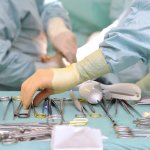
News • "Kognitiver Assistent"
KI soll zukünftig OP-Risiken mindern
Wissenschaftler des Universitätsklinikum Heidelberg entwickeln einen „Kognitiven medizinischen Assistenten“. Der Algorithmus soll das individuelle Operationsrisiko des Patienten im Vorfeld erkennen, Therapieentscheidungen erleichtern und Komplikationen vorbeugen. Das Projekt wird vom Ministerium für Wissenschaft, Forschung und Kunst Baden-Württemberg mit 2 Millionen Euro gefördert.
In another study, researchers used deep learning to predict kidney failure, mortality and post-operative bleeding after heart surgery. With their approach, the scientists achieved superior results compared to standard clinical reference tools.2
Clinical trials in AI-assisted surgery with evidence are scarce, and AI-assisted decision support has yet to realise its potential. Currently, decision support applications are limited to risk stratification of surgical treatment or its complications.3 In surgical oncology, AI studies are mainly limited to the feasibility of predicting oncological outcomes4, postoperative complications5 and predicting mortality and survival6.
For now, only a few comparative studies focus on improving treatment decision-making. Commercial attempts to address this problem have struggled with various issues. IBM Watson for Oncology Cognitive Computing System drew on data, test cases and experts at Memorial Sloan Kettering Cancer Center to generate treatment recommendations. However, studies have so far shown little consistency between Watson’s treatment recommendations and actual surgical treatments. Google’s DeepMind, on the other hand, was criticised for its lack of transparency7 and problems with data protection8.
OR planning
Scheduling operations, assigning patients, surgeons, nursing staff and anaesthetists – surgery planning in hospitals is a dynamic, complex, and time-consuming undertaking. Emergencies, staff absences or unforeseen events during a procedure are common short-term events that can quickly upset the schedule. Even for experienced OR managers, exact planning or possible manual adjustments are therefore difficult. The results are not only suboptimal utilisation of operating theatres and thus of resources but also inflict additional strain on staff.
Assistance management systems that schedule staff, treatments, times, resources as well as upstream and downstream processes around the operating theatre with the assistance of AI could provide a remedy. Based on the collection and analysis of data, these systems create a scheduling plan according to definable criteria, evaluate what is happening and adjust the plan in real time if unexpected events occur.
A stable OR plan not only relieves staff in the long term by eliminating shifts and overtime, but also increases treatment quality because the staff has more time to care for the patients. Optimised capacity also pays off for a hospital's economic situation by enabling better scheduling of staff and bed occupancy.
In the “KIOM” project, researchers are developing an interactive AI-supported assistance system for efficient planning of operations.9 It is intended to interactively support OR managers in scheduling surgeries. The combination of mathematical optimisation approaches with AI methods generates stable and transparent OR plans.
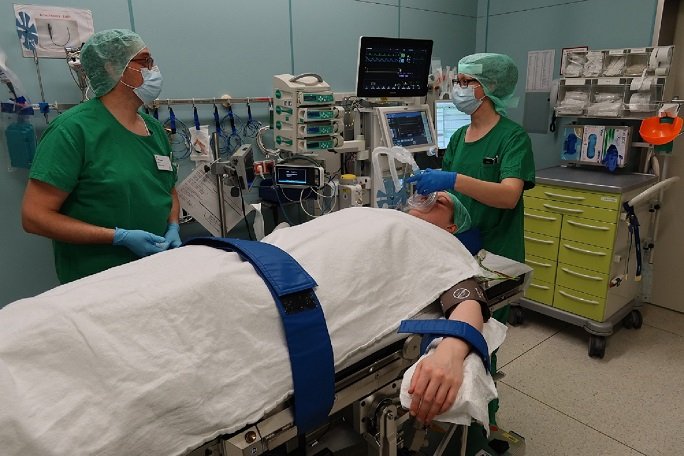
Image source: CASSANDRA project
Anaesthesiology
Many studies test AI applications for anaesthesiology in clinical settings. The aim is to predict potential complications with a personalised perioperative therapy and to sustainably improve the postoperative outcome of patients.
In the Cassandra project, researchers are developing and evaluating a virtual “AI team member” to support anaesthesiologists in the treatment and care of patients.10 Also, they aim to reduce the time and mental burden on staff and improve documentation quality through automatic documentation. In addition, researchers are exploring the potential for AI to control the mechanical ventilation of anaesthetised patients. Other trials study how the AI can automate weaning from ventilation.11
Further areas of application involve AI-based estimation of the correct opioid dosage and the identification of patients who could benefit from pre-operative counselling by a hospital's acute pain service.12
Concluding thoughts
Many AI software developments and studies on their applications show improvements over existing methods. But the hype around AI can lead to unrealistic expectations and disappoint clinicians and patients. Assistance and decision-making systems can give hospitals greater planning certainty and improve surgical outcomes, but operators should use them in the right situation to solve a specific problem. The examples of IBM and Alphabet show how complex the integration of AI systems into the clinical workflow can be.
References:
- "KoMeD" project of Heidelberg University Hospital (in German)
- Meyer et al., Machine learning for real-time prediction of complications in critical care: a retrospective study; Lancet Respiratory Medicine 2018
- Hasimoto et al., Artificial Intelligence in Surgery: Promises and Perils; Annals of Surgery 2018
- Kudo et al., Artificial Intelligence System to Determine Risk of T1 Colorectal Cancer Metastasis to Lymph Node; Gastroenterology 2021
- Mai et al., Artificial neural network model for preoperative prediction of severe liver failure after hemihepatectomy in patients with hepatocellular carcinoma; Surgery 2020
- Que et al., Application of preoperative artificial neural network based on blood biomarkers and clinicopathological parameters for predicting long-term survival of patients with gastric cancer; World Journal of Gastroenterology 2019
- CNBC: Google and DeepMind face lawsuit over deal with Britain’s National Health Service
- Powls, Hodson, Google DeepMind and healthcare in an age of algorithms; Health and Technology 2017
- "KIOM" project at the German Federal Ministry of Education and Research (in German)
- "Cassandra" project at the German Federal Ministry of Education and Research (in German)
- Martinoni et al., Model-based control of mechanical ventilation: design and clinical validation; British Journal of Anaesthesia 2004
- Tighe et al., Use of Machine-learning Classifiers to Predict Requests for Preoperative Acute Pain Service Consultation; Pain Medicine 2012
26.11.2022