Article • Diagnostic assistant systems
AI in endoscopy: helper, trainer – influencer?
Article: Wolfgang Behrends
Artificial intelligence (AI) is increasing its foothold in endoscopy. Although the algorithms often detect pathologies faster than humans, their use also generates new problems. PD Dr Alexander Hann (@Alexsworking) attests to the great potential of AI helpers. However, the deputy head of gastroenterology at the University Hospital Würzburg points out that their use can affect not only the reporting of findings – but also the person making the findings.
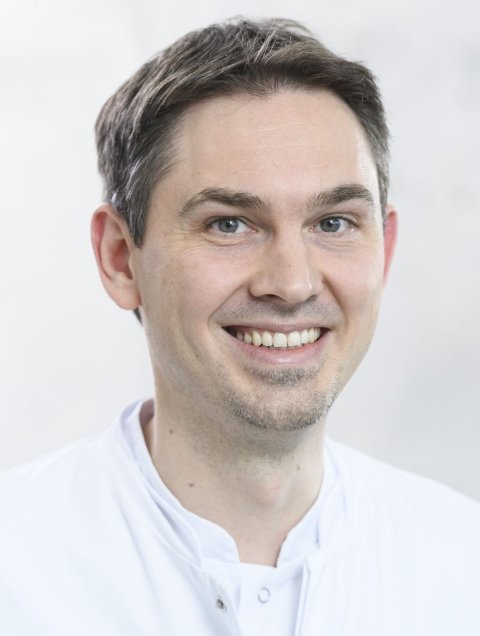
Image source: University Hospital Würzburg
‘On paper, artificial intelligence has learned from the best. We only provide it with the best data for training, so that in the end it should deliver above-average performance.’ The AI works deterministically, so it is not affected by daily form like humans but delivers results at the same level at all times. Some studies suggest decreasing performance over the course of the day in some endoscopic areas1,2 – ‘This human factor is taken out of the equation by AI.’
On the other hand, an algorithm needs a large amount of training data to reliably recognise pathological changes. In the case of rare diseases, humans therefore often have an advantage: ‘We tend to remember uncommon cases better and recognise special tumour precursors more easily once we have had negative experiences with them. That's not the case with AI.’
A scribe with structure and a stopwatch
One area where the technology is already established is colon cancer screening. ‘The first application that is already on the market is polyp detection software. Here, the AI looks at the endoscopy image at 30 frames per second like a second pair of eyes and marks the precancerous lesions it detects.’ Another application, which is currently in the wings, can interpret these findings and make a prediction as to whether a polyp remains harmless or can actually lead to cancer. ‘What's interesting is that this software doesn't give a probability as a number, but it commits: a finding is either classified as a harmless precursor or as an adenoma that can potentially become cancer.’ In cases that the AI cannot assess, the software will of course make no such prediction.
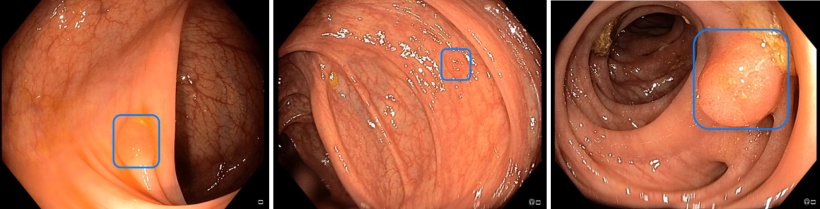
An intestinal polyp, highlighted by an AI for polyp detection, developed in the InExEn working group at the University Hospital Würzburg
Image source: Alexander Hann
Hann sees great potential for AI support in the creation of reports of findings – not a very demanding task, but one that takes a lot of time. In endoscopic examinations, determining the so-called withdrawal time is a particular challenge here: ‘Theoretically, we would even have to use a stopwatch to subtract the times we need for polyp removal from the total withdrawal time in order to measure how long the mucosa has been assessed,’ the expert explains. ‘Of course, this is not really realistic. So, we think artificial intelligence could help in determining these timespans and writing at least the rough draft of a standardised reporting.’
However, common standards are hard to establish due to the fact that almost all major manufacturers have their own AI solutions, which in turn are designed to fit the different endoscope models, Hann explains: ‘The devices work with different light sources and image sensors, and the image section is also always a little different – some have round corners, others do not. This always results in a different image. This makes it difficult to compare the AIs with each other.’
Moving images need context to make sense
Old data are a poor fit for training an AI for polyp detection
Alexander Hann
For AI training, however, it is not enough to simply feed the algorithm photos of previous finds, Hann points out – because still images from old archives do not show polyps in their original state: ‘When a polyp is found, it is usually covered by a mucus cap. For the photo, however, this cap is removed and the polyp is positioned in the middle of the picture – but this is not how it looked when it was discovered. This means that old data are a poor fit for training an AI for polyp detection.’
While “teaching” the AI using video sequences has the advantage of showing polyps as they would be seen during an actual endoscopic examination, the moving images also bring about new challenges: ‘Many of the current AI systems reinterpret the 30-50 images per second they process each time’. Lacking context, they can't tell if a polyp they detect is new – or the same one they saw just a few seconds ago. ‘This is still the subject of research activity at the moment,’ Hann reports. ‘Commercially available AIs with memory capacity don't exist yet.’ Currently, the classification is rule-based to avoid such repetitive findings. But typical disturbances in a video such as blurring caused by liquids on the lens and light reflections also pose challenges for the AI. ‘It's not straightforward, because such effects can sometimes fool even human viewers. Therefore, a key task is to keep refining the underlying rules.’
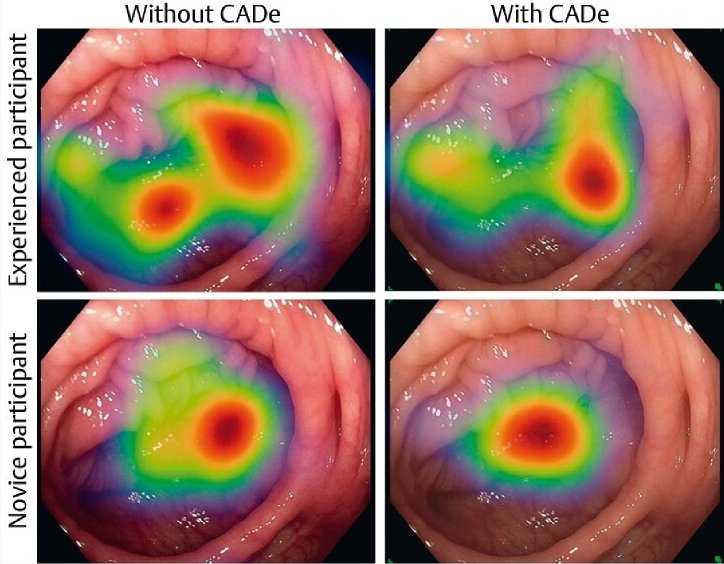
Can AI boxes lead to laziness?
Hann, however, does not consider the use of AI helpers in endoscopy to be a one-way street: the research group for interventional and experimental endoscopy (InExEn), which he leads, is currently investigating whether the use of AI tools has an effect on the behaviour of the medical examiner.3 ‘This influence has hardly been researched. There are industry-made products on the market, and we use them. But in doing so, we have little understanding of what they are actually doing to us.’
For example, the InExEn group studied the behaviour of medical professionals who were shown videos of endoscopic examinations in two variants – with and without AI-generated detection boxes. ‘We found that the eye movements changed when the AI markers were switched on: The diagnosticians did not search the monitor as thoroughly for polyps. We deduce from this that the examiner relies – at least to some extent – on the AI.’
Hann therefore advocates letting inexperienced endoscopists work without assistance systems at first, so that they can gather their own expertise. ‘It's a bit like the navigation system in a car,’ he points out: if you are used to following the instructions of the navigation system, you run the risk of paying less attention to the signs along the route. ‘In my opinion, this is a danger that we should at least be aware of. So far, there is hardly any research on the influence of AI on humans. We should change that before we deploy these systems across the board.’
Farewell to the black box
Nevertheless, Hann is convinced that the technology can also be used to improve endoscopy training: ‘If the AI itself shows us what it is looking for and gives examples, this could be used to better train inexperienced colleagues.’ At the same time, this would be a step towards greater AI transparency, the expert points out – because so far, the algorithm's diagnostic criteria can hardly be reproduced.
References:
- Brennan et al.: Does time of day affect polyp detection rates from colonoscopy?; Gastrointestinal Endoscopy 2011
- Lee et al., Endoscopist fatigue estimates and colonoscopic adenoma detection in a large community-based setting; Gastrointestinal Endoscopy 2017
- Troya et al.: The influence of computer-aided polyp detection systems on reaction time for polyp detection and eye gaze; Endoscopy 2022
20.10.2022