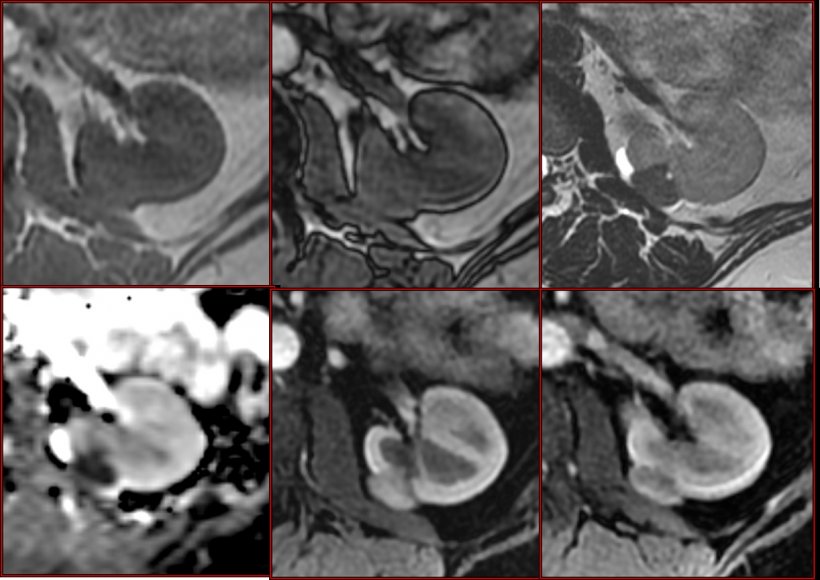
Article • Morphology, texture, function, metabolism
Radiomics will transform tumour characterisation
Tumours change over time – and not only in size. They also evolve genetically, mutate and spread through equally diverse metastases. Each is unique and present with a more or less complex structure, but rarely as a unified entity.
Report: Melisande Rouger
Characterising them from A to Z and from detection to neutralisation remains a challenge for modern medicine. Radiomics could be a powerful ally to assess tumour heterogeneity and replace the invasive and insufficient methods that are currently used, according to prominent French radiologist Nicolas Grenier who spoke during the last meeting of the European Society of Urogenital Radiology.
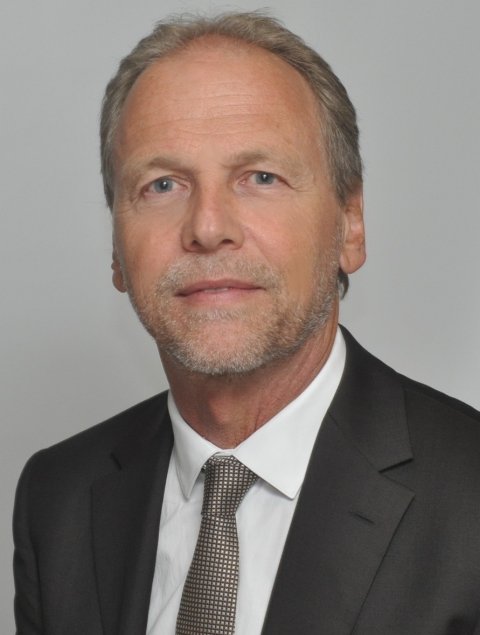
Physicians are still limited when it comes to assessing tumour heterogeneity in terms of phenotypes or genotypes. The methods that are currently used in reference centres to that purpose are mainly to perform biopsies at 5 or 6 different sites of the tumour. Not only are these methods invasive, they are also limited over time, according to Nicolas Grenier, head of urogenital and vascular radiology at Pellegrin University Hospital in Bordeaux. ‘These methods just provide useful information for tumour mapping, but they do not enable assessment of tumour evolution over time with gene expression modifications, or to spot new localisations, i.e. metastases,’ Grenier said.
Tumours do not have a permanent state and there may be more than one story within the same lesion. ‘Within the same tumour you may have different fragments that do not have the same severity, or the same genes or expression factors. You can have mutations in all the cells or just in a few regions,’ Grenier said. Over time, the tumour may spread through metastases, which may also not have the same phenotypes. Depending on their location in the body, these new lesions may present very distinct phenotype expression.
Radiomics could help solve tumour heterogeneity problems with the passing of time. The whole idea behind radiomics is to extract parameters on morphology, texture, function or metabolism, which enable characterisation of the entire tumour and its corresponding metastases. These parameters, or descriptors, once correlated with genotypes, or clinical or treatment response data, can give a full picture of both spatial and temporal tumour heterogeneity and provide prognosis prediction. However, to implement this game-changing approach, we need to review the way we perform scientific research completely, Grenier stressed. ‘We are still following Descartes’ principle and start with a hypothesis to build an entire protocol, which is supposed to confirm or infirm that hypothesis. To work with radiomics, we must do the exact opposite, i.e. screen an entire population to extract descriptors, to formulate a hypothesis, for instance which population may be more receptive to a given treatment,’ he said.
The imager’s eye is important in quality control, and also to ensure that the data remains pertinent within the context
Nicolas Grenier
In the end, radiomics works a little like genomics, in which genes are extracted from a population cohort to determine which groups have which genome, information that can help assess who will better respond to a particular treatment.
Artificial intelligence (AI) will provide the horsepower that is needed to mine and select meaningful data generated by algorithms. These algorithms extract thousands and thousands of data, and AI can help sort out the most interesting and pertinent of these, which, once correlated to genetic or clinical information, will help advance our research. ‘AI will also save time and expedite work by taking care of simple but repetitive tasks, enabling physicians to focus on more interesting parts of their jobs,’ Grenier added. Nonetheless, radiologists must keep an eye on the quality of data extracted by machines, to ensure images are free of artefacts, noise and movement, and simply good enough to work with, he insisted. ‘The imager’s eye is important in quality control, and also to ensure that the data remains pertinent within the context.’
Recommended article
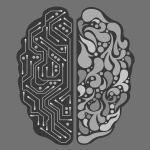
Article • AI in radiology
Augmented intelligence rather than artificial
Artificial intelligence (AI) will increase efficiency and improve quality as well as clinical outcomes – and thus strengthen rather than weaken the role of radiologists, said Dr Joon Beom Seo at ECR 2018. A spectre is haunting radiologists – the spectre of artificial intelligence. Is AI about to replace radiologists? Wrong question,’ declared radiologist Dr Joon Beom Seo, professor at the…
Research with radiomics in kidney tumour is just beginning, with less than 10 publications so far, each focusing on a particular aspect of the field; for instance using CT to extract purely morphological parameters for treatment response, collecting functional parameters based on MR PET to correlate with tumour grade information, etc. ‘All these studies are experimental and carried out at a single centre. Besides, most studies have a commercial purpose and aim to determine which is the most appropriate algorithm for which context in order to sell it. We have nothing really practical so far. We’ll have to sort out the results and compare experience,’ Grenier pointed out.
Pellegrin Hospital has a few initiatives underway using AI, notably in cardiac arrhythmia and stroke, and has just started a project to develop radiomics in oncology, in cooperation with Sophia Genetics and local radiology teams including the Bergonié Cancer Institute. Grenier and his colleagues are working with what he called pre-radiomics, i.e. multiparametric MRI in kidney tumour, with encouraging results. ‘We can now describe the tumour histological type based on MRI sequences, something that was only possible through biopsy before.’ Radiomics will enable things to advance further, notably by classifying tumour types and grades, determine which part of a tumour has the highest grade, and assessing early treatment response for instance in metastatic cancer using anti-angiogenic therapy, he concluded.
Profile:
Nicolas Grenier is a professor of radiology and head of urogenital and vascular radiology at Pellegrin University Hospital in Bordeaux, France, where he also coordinates the radiology and nuclear medicine departments.
21.03.2019