Article • AI in radiology
Augmented intelligence rather than artificial
Artificial intelligence (AI) will increase efficiency and improve quality as well as clinical outcomes – and thus strengthen rather than weaken the role of radiologists, said Dr Joon Beom Seo at ECR 2018.
Report: Michael Krassnitzer
Source: Pixabay/Seanbatty
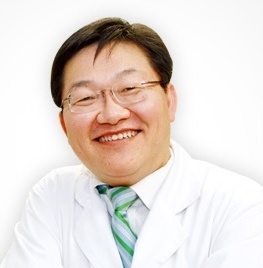
A spectre is haunting radiologists – the spectre of artificial intelligence. Is AI about to replace radiologists? Wrong question,’ declared radiologist Dr Joon Beom Seo, professor at the Artificial Intelligence for Medical Imaging Research & Development Centre, University of Ulsan in Seoul, South Korea, during his presentation at ECR 2018. He responded with an alternative question: ‘How can we improve and reposition radiology by integrating AI technologies?’
The term artificial intelligence in the context of radiology usually describes applications based on deep learning: an artificial system learns from examples by autonomously recognising patterns and structures. To date, such applications are already available for all areas of image analysis, be it image conversion and segmentation of lesions and organs or the detection and classification of lesions.
Seo cited some examples of image conversion applications: images acquired with a certain filter can be converted in a way that they seem to have been acquired with a different filter. While in low-dose CT deep learning applications can reduce noise; in Diffusion Tensor Imaging (DTI) they can increase image quality such as spatial resolution from 2.5 mm to 1.25 mm and the number of gradients from 15 to 90. ‘Image conversion using deep learning methods is a promising area,’ Seo pointed out, because it might, inter alia, solve the issue of heterogeneous data in multi-centre studies.
Introducing this technology into clinical routine will be the dawn of a new era
Joon Beom Seo
‘AI-assisted segmentation of lesions and organs can already be carried out in a fully automatic way,’ the South Korean expert suggested. For example, the segmentation of organs in abdominal CT, or the detailed visualisation of the respiratory tract in thoracic CT. ‘Deep learning methods display the organs as well as manual post-processing. However, the computer will do in two minutes what the radiologist does in two hours.’ Thus, AI offers a significant reduction in time and effort. ‘Introducing this technology into clinical routine will be the dawn of a new era,’ Seo said, with enthusiasm. The same, he believes, holds true for detection and classification of lesions. AI already delivers great results in the case of CT detection of lung nodules. This could also be introduced to clinical applications, said Seo: the computer might check the scans for suspicious areas and report them for review before the physician looks at the images.
Nevertheless, at this point AI still operates in a rather restrictive environment, Seo emphasised, pointing at political and legal concerns regarding privacy and commercial use, but also at technological issues. Most importantly, however, many radiologists are worried about the fact that deep learning systems are black boxes: ‘We do not know how a system arrives at its results,’ Seo explained.
Who teaches the machine?
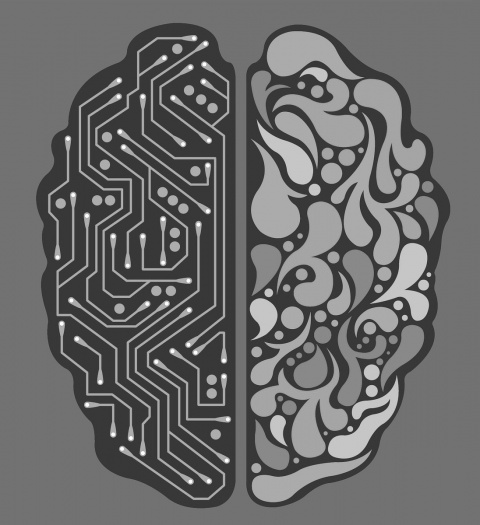
Currently, AI applications in medical imaging are still based on supervised learning processes, i.e. human specialist input is necessary. This raises the issue of who should or may train the systems. ‘If you look for example at the detection of diabetic retinopathy you will see that there are large interobserver differences,’ he said. Which goes to show that even a deep learning system depends on the quality of the data it uses to learn. ‘Artificial intelligence won’t replace radiologists, but rather strengthen their role by improving quality and clinical outcomes and increasing efficiency,’ Seo said, returning to the initial question. AI thus is a tool not a replacement of the radiologist: ‘We should,’ he concluded, ‘call it augmented rather than artificial intelligence.’
Session: Joon Beom Seo: Artificial intelligence applications in radiology, ECR 2018
Profile:
Joon Beom Seo MD PhD, is professor at the University of Ulsan College of Medicine in Seoul, South Korea, and attending physician in radiology at the Asan Medical Centre in Seoul.
28.11.2018