Article • Deep Learning vs. Machine Learning
Radiomics strengthens breast imaging
The field of AI-enhanced imaging provides radiologists with an unprecedented opportunity to shape patient care, a leading Austrian radiologist explained at ECR 2020.
Report: Mélisande Rouger
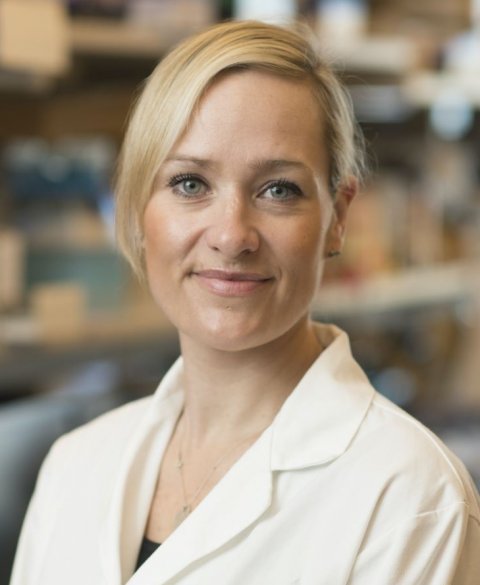
Workflow with radiomics starts with image acquisition and segmentation. When the region of interest is defined, radiomics analysis enables extraction of a large quantity of imaging features, and then to select, reduce, classify and model the data to answer a specific question. ‘Machine learning or deep learning may be used at any step of the process and help in various aspects of daily practice,’ said Katja Pinker-Domenig, a breast radiologist at the Memorial Sloan Kettering Cancer Centre, USA, and the Medical University of Vienna in Austria.
When it comes to mammography, the most common use case for machine learning (ML) or deep learning (DL) is screening accuracy. Studies have shown that deep convolutional neural networks (CNN) achieve area under curves (AUC) of about 0.9, regardless of the framework. Researchers now want to take it one step further and are looking at performance of radiologists on mammograms with or without the help of artificial intelligence. AI support has been shown to increase sensitivity and there is a trend towards increasing specificity. ‘When the radiologist is provided with a decision support system, performance improves, not reading time,’ Pinker-Domenig said.
Recommended article
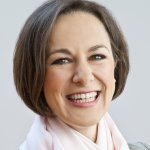
Article • Radiology + data + AI = ?
Today and future radiomics
Radiomics is one of the most exciting topics in radiology. It involves data and artificial intelligence (AI) but very few people know or understand the details. In her lecture ‘How does Radiomics work?’, presented at the German Radiology Congress in Leipzig, Professor Ulrike Attenberger outlined how radiomics will advance radiology but also the obstacles faced along the way.
With ultrasound, the main application has been classification, to differentiate between benign and malignant masses. Ultrasound is recommended when a suspicious mass has already been spotted. However, to date, there are no guidelines that recommend using AI with this modality in clinical practice. ‘More studies are needed to explore advanced DL methods and prove their usefulness,’ Pinker-Domenig underlined. ‘It’s an area of interest.’ A study by Barinov et al (Journal of Digital Imaging, June 2019) has shown that an FDA approved AI-decision support system incorporated in image analysis improved accuracy in breast cancer diagnosis for all radiologists, regardless of their level of experience. AI-enhanced MRI has by far been the most explored area, particularly in lesion detection. ‘Research has shown that fully automated detection of breast cancer using CNN is possible and this is very encouraging, in particular with the trend of abbreviated MR protocols in breast cancer screening,’ she added.
With CNN, the next step is classification. Authors of another study applied AI based breast lesion detection classification imaged to multiparametric breast MR protocol with ultrafast DCE-MRI, Tw2 and DWI. The researchers could show that the final AI system that incorporates information from all sequences showed significantly higher performance than DEC-MRI alone. When operating at the same sensitivity level as radiologists it was compared to, AI-enhanced MRI improved sensitivity and detected less false positives than humans, potentially sparing biopsy. However, in another study, radiologists did perform better than ML and DL, which performed better than ML (AUC: 0.88 vs. 0.81) for lesion classification. Radiologists should thus strive to use DL rather than ML algorithms, especially for complicated cases such as BRCA mutation carriers, Pinker-Domenig explained.
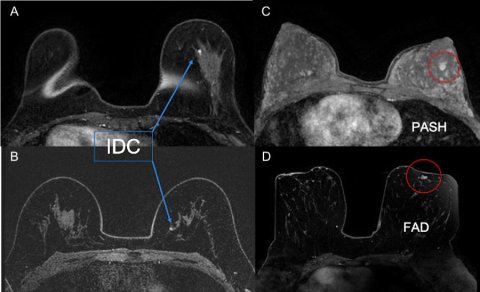
‘We should identify cases in which we struggle, for example small enhancing masses in BRCA mutation carriers. These patients often undergo high-risk MRI screening and present with small tiny lesions that probably have a benign appearance but can be cancer. Our awareness level in this patient collective is already altered compared with an average risk of breast cancer,’ she said. According to a recent study, the BI-RADS descriptors are not very useful to classify lesions, with a 53% diagnostic accuracy, 73% sensitivity and 42% specificity.
By contrast, radiomics diagnostic accuracy is almost 80%, and sensitivity and specificity are also higher (Figure 1). ‘This shows potential for AI to be used as an adjunct tool, to spare unnecessary biopsies for benign appearing small breast masses. This can be very useful in these patients, who undergo repeated screening and biopsies,’ she said.
Prediction and prognosis at hand
With AI enhanced MRI, radiologists can also try themselves at prediction and prognosis. Molecular subtyping with DCE-MRI has been studied extensively. Specific molecular subtypes seem to carry radiomics signatures that can be used to accurately classify those lesions with respect to receptors – the molecular subtype – with good diagnostic accuracy. These signatures have the potential to provide prognostic indicators that are derived from the entire tumour and that may be used to monitor tumour biology changes during treatment. This function can be extended to contrast-enhanced mammography, which has the same underlying psychological mechanisms as MRI and can be used as an alternative when MRI is not available.
DWI has also shown ability to detect lesions and DWI radiomics signature might have the potential to provide a non-invasive, contrast agent-free method to assess tumour biology before, during and after treatment, Pinker believes. ‘Luminal B and HER-2 enriched cancers seem to carry distinct radiomics features and may be used in this context.’
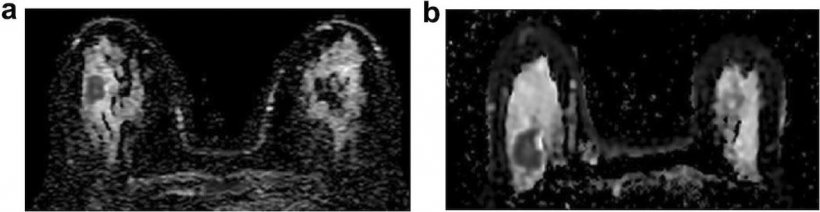
Image source: Leithner et al.: Radiomic Signatures Derived from Diffusion-Weighted Imaging for the Assessment of Breast Cancer Receptor Status and Molecular Subtypes; Molecular Imaging and Biology 2019 (CC BY 4.0)
Another potential application is prediction of recurrence scores, which are currently assessed by genetic testing, a costly and often unavailable solution for many countries around the world. Imaging, when it is available, could offer an alternative with non-invasive testing. ‘CNN achieved good class accuracies for prediction of low, intermediate and high-risk patients. CNN architecture can be trained to predict oncotype recurrent scores and offered to patients when genetic testing is not available,’ Pinker suggested. Prediction of treatment response is also at hand with ML combined to multiparametric MRI, as the combination allows prediction of pCR and survival outcomes with high accuracy.
Our greatest challenge will be to make our clinical partners adapt our AI enhanced imaging methods in their clinical workflow
Katja Pinker-Domenig
Allying radiomics with other data appears as a promising option. ‘When we combine radiomics and other features, such as pathological stage or genomic type, we achieve the best performance. MRI can add in patient assessment for patient outcomes,’ Pinker said. Researchers have also found that an image-based DL model to predict the five-year risk of breast cancer based on a single breast MR image from a single examination improves individual risk discrimination when compared with a state-of-the-art risk assessment model.
AI power is up for grabs but many challenges lie ahead, including the need for continuous study and the lack of a standard method for segmentation, feature extraction and selection and classification. ‘We have small samples size and need further studies with large datasets and with subgroup analysis by patient group and/or tumour type. Independent testing is necessary for meaningful clinical implementation,’ Pinker pointed out. With ML and DL tools in breast imaging, radiologists have an unprecedented opportunity to better derive clinical value from imaging data and reshape the way they care for patients. ‘Our greatest challenge will be to make our clinical partners adapt our AI enhanced imaging methods in their clinical workflow,’ Pinker concluded. ‘But I’m pretty confident think we will get there.’
Profile:
Katja Pinker-Domenig MD PhD is a radiologist and associate professor of radiology at the Department of Radiology, Breast Imaging Service, Memorial Sloan Kettering Cancer Centre in New York, and Department of Radiology, Molecular and Gender Imaging Service, Medical University of Vienna.
21.10.2020