Image: Shutterstock/Bimbim
Article • Radiology + data + AI = ?
Today and future radiomics
Radiomics is one of the most exciting topics in radiology. It involves data and artificial intelligence (AI) but very few people know or understand the details.
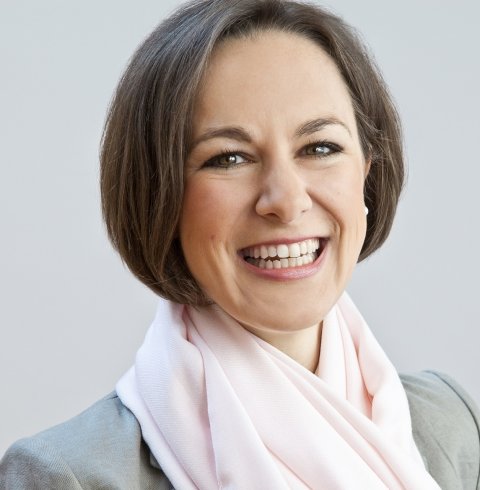
In her lecture ‘How does Radiomics work?’, presented at the German Radiology Congress in Leipzig, Professor Ulrike Attenberger, Vice Chair and Medical Director from the Institute of Clinical Radiology and Nuclear Medicine in Manheim University Medical Centre, Germany, outlined how radiomics will advance radiology but also the obstacles faced along the way.
Images become numbers – The central idea behind radiomics is the extraction of standardised, quantitative, multidimensional information from CT or MRI image data sets using artificial intelligence procedures.
Medical image features are extracted, analysed and modelled to predict treatment-relevant targets – such as progression – and survival rates, or the response of a tumour to certain types of therapy
Ulrike Attenberger
Obtaining standardised, quantitative information from images is currently a key issue. ‘The evaluation of radiological images still depends on the individual experience of the radiologist.’ In the case of liver lesions, for instance, criteria that are not particularly tangible, such as the uptake behaviour of a contrast agent, are being used. Laboratory values, on the other hand, can be quantified as numbers, and thresholds clearly distinguish between normal and pathological values. ‘In radiology, we only really have this to a very small extent,’ Attenberger points out.
This is where Radiomics comes in. With the help of AI procedures, quantitative information is extracted from image features which can then be integrated and evaluated with clinical, molecular and genetic data. ‘It is hoped that this will allow a more precise prediction of clinical endpoints than is currently possible with individual analysis of image features.’ The ability to quantify image features in a standardised way is a basic prerequisite of personalised medicine. This is needed to develop image-based biomarkers.
This happens with Radiomics Workflow: ‘Medical image features are extracted, analysed and modelled to predict treatment-relevant targets – such as progression – and survival rates, or the response of a tumour to certain types of therapy.’ Researchers have discovered the potential of this technology not only for oncology but also for analysis in cardiac imaging. Radiomics applications already improve the differentiation between calcified and non-calcified plaques and enable better identification of patients with acute myocarditis.
Recommended article
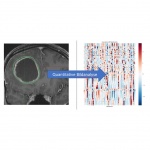
Article • Therapy monitoring
Liquid biopsy versus radiomics – the race is on
The development of new procedures to monitor cancer treatments is gathering momentum. One such innovation is liquid biopsy. This new lab technique allows non-invasive identification, characterisation and monitoring of circulating tumour DNA. Thus, liquid biopsy can potentially revolutionise oncological diagnostics – and put a spoke in the wheel of radiology. High time to act, says Professor Dr…
From diagnostician to machine trainer
‘This is a revolution comparable to the industrialisation in the 18th and 19th centuries,’ Attenberger says. ‘We need to completely rethink and change our diagnostic routines.’ The image itself is no longer at the centre, but the training of a machine, or an algorithm respectively, which extracts information from image features that is not apparent to the human eye.
The computer can create heat maps and correlate the information generated from the image with clinical data such as outcome, disease progress or genetic data and laboratory values. ‘This is a very complex, multistage process where statistical procedures play an important part. Although it may seem paradoxical, many features become reduced during this process, but this is necessary to avoid overfitting, i.e. over-adaptation due to accounting for too many variables, sometimes barely relevant to the issue. The results of this “training” are then validated using new sets of data.’
The Holy Grail and the black box
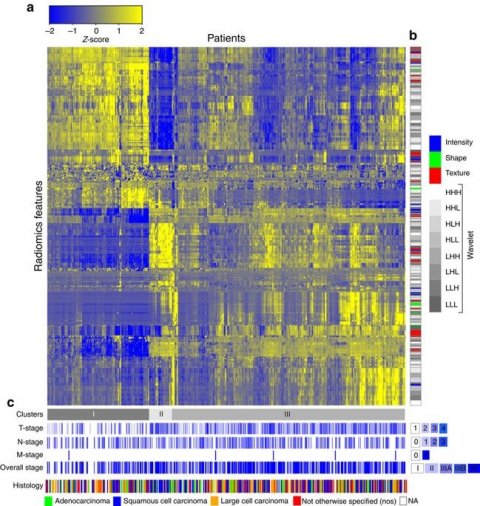
Radiomics heat map: (a) Unsupervised clustering of lung cancer patients (Lung1 set, n=422) on the y axis and radiomic feature expression (n=440) on the x axis, revealed clusters of patients with similar radiomic expression patterns. (b) Clinical patient parameters for showing significant association of the radiomic expression patterns with primary tumour stage (T-stage; P<1 × 10−20, χ2 test), overall stage (P=3.4 × 10−3, χ2 test) and histology (P=0.019, χ2 test). (c) Correspondence of radiomic feature groups with the clustered expression patterns.
Source: Hugo J. W. L. Aerts, Emmanuel Rios Velazquez, Ralph T. H. Leijenaar, Chintan Parmar, Patrick Grossmann et al.: Decoding tumour phenotype by noninvasive imaging using a quantitative radiomics approach; Nature Communications; © 2014, Springer Nature
Consequently, at the end of this calculation there are figures that describe how precise the trained model is, to deliver conclusions as to how aggressive a tumour is, for instance, or how well it will respond to a certain treatment. The latter is not easy to determine, the expert explains: ‘This is still a bit of a Holy Grail. Initially, we have to model how the tumour is likely to change during treatment. The details of quantifiable changes before and after therapy must then be correlated with other variables, such as genetic or clinical data. We can then, in theory, derive a predictive model which can potentially deliver predictions on effectiveness.’
However, as yet this approach is nowhere near ready for clinical application. First, unified standards must be determined that can be used to edit new data. ‘We’ve actually seen some progress here, not least due to projects such as the Image Biomarker Standardisation Initiative (IBSI) or the Radiomics Quality Score Initiative from Professor Lambin in Maastricht.’
AI could be another way to balance the heterogeneity of the protocols, which is currently making a universal use of analysis procedures impossible. Before any approaches to this become established one of the most prominent demands from experts is to reveal the source codes of the algorithms in large databases, so that the procedures can be made repeatable.
Profile:
Professor Ulrike Attenberger is Vice Chair and Medical Director at the Institute of Clinical Radiology and Nuclear Medicine at the Manheim University Medical Centre. After gaining her medical degree in Munich 2006, her doctorate followed on the ‘Importance of MRI in the diagnosis of pulmonary hypertonia’. Her research focus is now on MRI, for example its use in tumour diagnosis, and the evaluation of therapy response. The professor received a Fellow Award from the RSNA for her work on the optimisation of dose reduction for contrast-enhanced MR angiographies. She also received the Walter-Friedrich Prize from the DRG (German Society of Radiology) for her habilitation thesis on the implementation and evaluation of new acquisition and processing procedures to expand MRI applications in routine clinical diagnostics.
03.12.2019