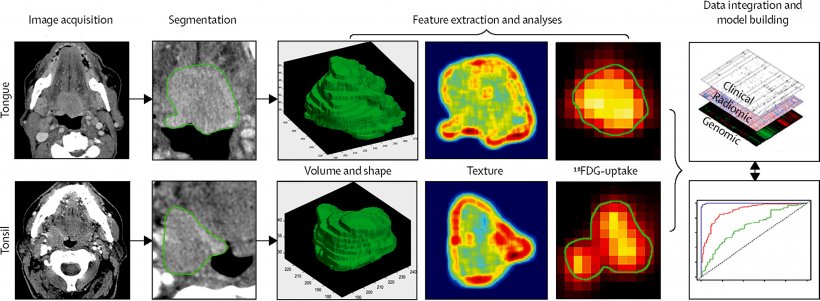
Article • Distributed learning
Radiomics on tap in 5-10 years
Keeping data within the hospital by sending the learning modules to each hospital database might prove a game-changer in radiomics, a leading Dutch researcher will show at ECR 2019.
Report: Mélisande Rouger
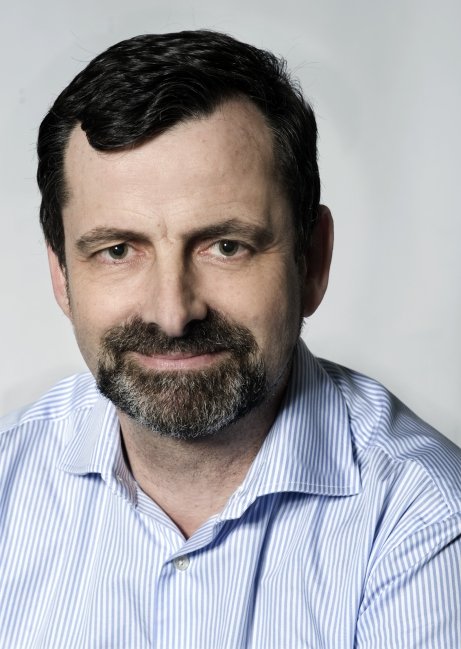
Radiomics, a field that aims to extract large amounts of quantitative features from medical images using data-characterisation algorithms, is a major advance for healthcare, according to Philippe Lambin, a radiation oncologist from Maastricht University. ‘The information delivered by radiology, and more generally healthcare, is still based on a qualitative and semi-subjective assessment. There aren’t that many quantitative data in the radiology report,’ he pointed out. Radiomics will help to remedy this situation by enabling the extraction of quantitative and measurable information on intensity, shape and texture of tumours, plus wavelengths, and complex semantic features that can represent frictions, for instance contact between a tumour and the bone.
15,000 to 20,000 quantitative image characteristics can be generated with radiomics to show gene mutations, differentiate aggressive from non-aggressive tumours, and assess treatment response. The histological type of the tumour can also be determined by mining biological information from a CT scan. Within five to ten years, every hospital will use a radiomics solution, Lambin believes. ‘A genomic signature means taking and sending a tissue sample to a lab. Extracting information from an already existing image is cheaper and much more attractive. Also, a biopsy may not represent a tumour’s evolution over time. All these issues disappear with radiomics,’ he said. In the meantime, there are still major obstacles to its implementation in clinical practice, and radiomics algorithms must be rigorously validated and fulfil an unmet clinical need to pay off. ‘Radiomics, today, is in a chasm – the valley of death,’ Lambin said to describe the gap between scientific validation and applications in clinical routine.
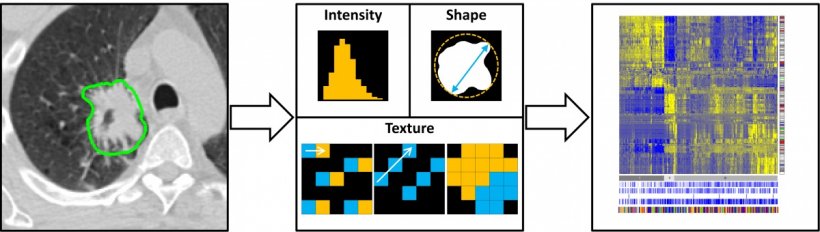
Overcoming data confidentiality legislation
To be adequately trained, algorithms must be fed huge amounts of data from millions of images from different countries. Collecting these images and sharing the data is an issue, with all the existing data confidentiality legislation. Sending the learning modules to each hospital database, instead of having the hospital send their data into a centralised system, would enable that difficulty to be overcome, Lambin believes. He and his team at Oncoradiomics, a Maastricht University spinoff, have developed such a solution, which they have called ‘distributed learning from federated databases’. ‘The benefit is that hospitals keep their databanks within their systems, which are protected by firewalls, rather than centralising the multiple databases from different countries,’ he said. The researchers already tested distributed learning at various locations across Europe and the USA, with results as good as software centralising data.
If we could have a million data, I bet that deep learning would be better than traditional radiomics. But we would still have the issue of an uninterpretable algorithm
Philippe Lambin
Another condition for radiomics to be implemented in clinical practice is to use quality research. A quality score to assess studies is available on the radiomics.world website, which guarantees enough patients are involved without external validation. Lambin recommended using the TRIPOD classification to assess quality of the biomarkers used in a trial. ‘Radiomics signatures must meet the TRIPOD level 4 to be deemed worthy. Prospective studies may also be required, with a predetermined signature that needs to be validated in the study,’ he said. Software must also receive CE mark or FDA approval. Integration into the hospital workflow is key for the solution to bring value. Last but not least, reimbursement can be problematic when financial incentives go in the wrong direction. ‘Using our radiomics signature, we can reduce the number of useless interventions in kidney cysts, for example, but in some countries hospital managers argue that they are paid per intervention,’ he said.
Combining deep learning with radiomics
An interesting path to explore in the future is the alliance of radiomics and deep learning (DL), notably to develop non-invasive imaging based biomarkers for radiomics. ‘Current research combines the best of DL, in particular automated segmentation, with the best of radiomics, by enforcing robust image characteristics into DL,’ Lambin pointed out. ‘This looks like a promising approach.’ DL uses a radically different method than radiomics, in which image characteristics with an already known image signature, for instance heterogeneity, are selected. So far no one can explain how DL works exactly. ‘It’s a black box; we don’t really know how it works, which is annoying because doctors like to understand the process,’ he said.
DL also requires more horsepower to train its algorithms to distinguish between a malignant and a benign tumour. To feed the beast, DL needs at least 10,000 images and, sometimes, synthetic data. ‘If we could have a million data, I bet that DL would be better than traditional radiomics. But,’ he concluded, ‘we would still have the issue of an uninterpretable algorithm.’
Profile:
Philippe Lambin is a clinician, radiation oncologist and pioneer in translational research, with a focus on hypoxia and Decision Support Systems. He has a PhD in Radiation Biology. In 2016 and 2018 he was an ‘ERC advanced & ERC PoC grant laureate’. He is also co-author of more 450 peer reviewed scientific papers (Hirsch Index) Google scholar: 86. A co-inventor of more than 18 patents, of which five are in the (pre) commercialisation phase, he has also co-promoted over 50 completed PhD’s. Lambin is among the creators of ‘Radiomics’ and ‘Distributed learning’ a revolutionary Big Data approach for healthcare.
26.02.2019