Article • Post-hypothesis analysis
The mechanics of radiomics
Radiomics have the potential to generate new hypotheses and patient profiles, and probably to discover new genes, prominent French researcher Prof. Laure Fournier explained in a dedicated New Horizons session at ECR.
Report: Mélisande Rouger
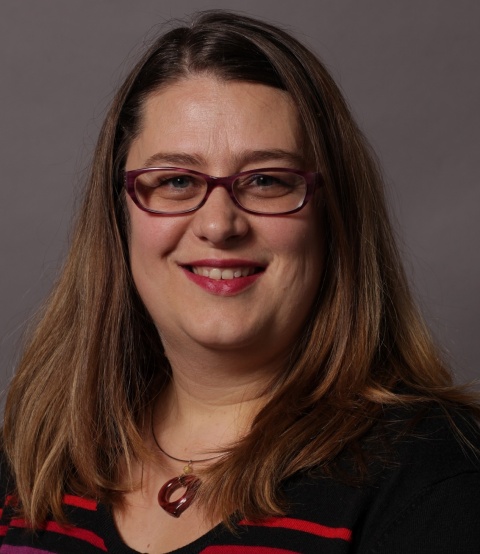
Confirming or infirming hypotheses has long driven scientific research; however, this traditional and costly approach is giving way to data-driven initiatives, according to Prof. Laure Fournier, a leading radiologist at Georges Pompidou European Hospital in Paris. “Usually we formulate the hypothesis first, then take an image and analyze it. We like that in France, it comes from Descartes. The problem is that it’s very expensive and time consuming, because each time you have an idea, you have to put together a research project,” she said. There has been a paradigm shift and researchers are now first looking at the data to try to extract meaning out of it before they formulate a hypothesis. “This is discovery research. We’re not validating something; we’re trying to discover new things that we hadn’t thought of,” Fournier said. This is the same principle behind radiomics, which consists of extracting large sets of complex descriptors from clinical images, without any prior hypothesis.
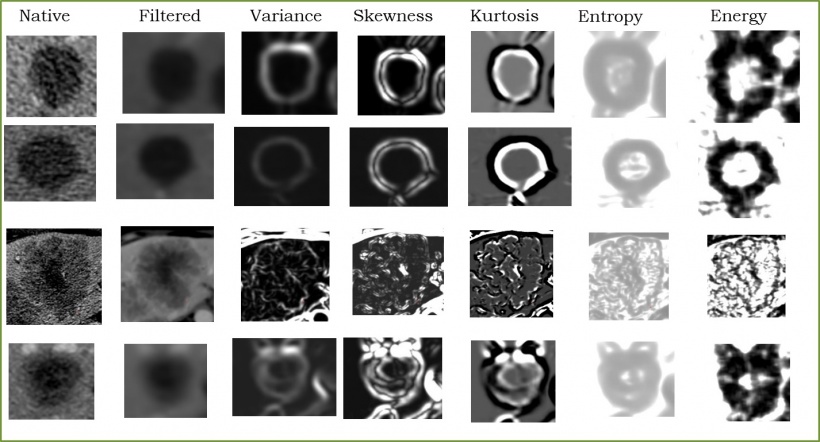
These techniques are interesting to quantify heterogeneity on the pixel scale; they give more information than the human eye
Laure Fournier
Images offer much more than just looking at size or morphology, Fournier highlighted. “Images are phenotypes. What we want to stress and tell our clinicians is that an image is a reflection of all the biology that’s going on underneath. There is a lot of information in our images besides measuring size in cancer, so we should try to get a lot more information from them,” she said. With radiomics, a wide variety of parameters – on tumor geometry, size, contours, anfractuosity, irregularity and directionality, as well as pixel composition – can be extracted from features from CT, MRI and PET studies. These data are then converted into high-dimensional data, and their mining is used to detect correlations with genomic patterns; this process is known as radiogenomics. To use radiomics properly, one must first collect and extract all the data contained in an image. Then comes the process of data depuration, i.e. cleaning the data and making it uniform, and finally processing this data to select appropriate parameters. “The most common way to process these parameters is by using a two-step approach: first we reduce the number of features based on their technical quality and then we test them for their clinical relevance,” Fournier said.
To process all the data contained in an image, dedicated software and AI tools are available in open source. Nonetheless Fournier’s team has developed their own software, which is now able to collect over 1,700 parameters per image, a capacity that tremendously improves the chance of not missing any relevant finding. “These techniques are interesting to quantify heterogeneity on the pixel scale; they give more information than the human eye. They’re more precise and subtle,” she said. Only a few parameters will be technically relevant though. To reduce the number of features to those that truly matter, Fournier performs quality selection based on reproducibility. “What we want is to select features that are reproducible. Another problem is that a lot of these parameters are very close to each other and may give you the same information. Once you get rid of useless parameters, you end up with a small number of features.”
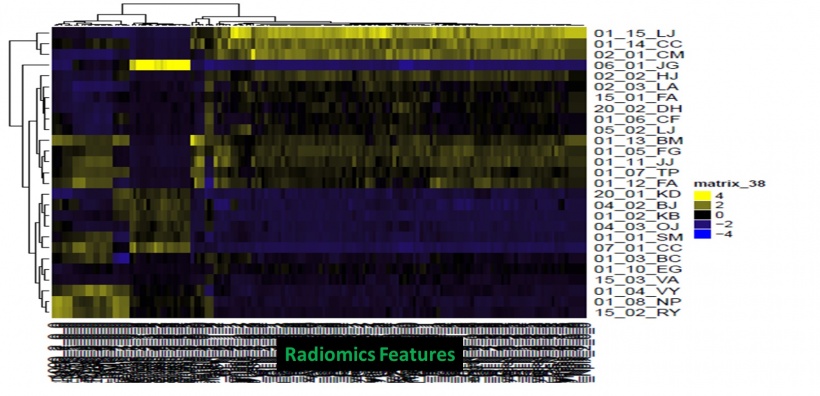
As for clinical relevance, Fournier’s team focused on identifying predictive radiomics signature to predict prognosis survival prediction, with good results. “We obtained a PSS of almost 3 vs. 18 months, so this clearly separates two very different populations,” she said. Although software and AI tools are easy to use, radiologists need a lot of help from bio statisticians, engineers, data scientists and computer scientists to operate radiomics properly. “You will need a true multidisciplinary approach if you want to delve deep into radiomics,” she said.
One of the main challenges remains acquisition variability. “We should work with data acquired from multicenter studies, because if parameters aren’t reproducible in that setting, they’re dumped out in the garbage. So if we use multicenter data to start with, we can avoid that challenge,” Fournier said. Integrating all the data, not just images, and having big cohorts are also paramount for the development of radiomics. There is no doubt that radiomics will significantly improve research, by allowing testing simultaneously a very large number of new parameters that are extracted from images, Fournier believes. “The idea is that new biomarkers will merge, and to pan all these biomarkers and generate new hypotheses. Radiomics might lead us to new patient profiles and find new genes and proteins that might explain disease.”
Profile:
Laure Fournier, MD, PhD, is radiologist at Georges Pompidou European Hospital in Paris and Professor of Radiology at Paris Descartes University, Sorbonne Paris Cité.
10.07.2018