News • Detecting depression, psychosis
Machine learning could aid mental health diagnoses
A way of using machine learning to more accurately identify patients with a mix of psychotic and depressive symptoms has been developed by researchers at the University of Birmingham.
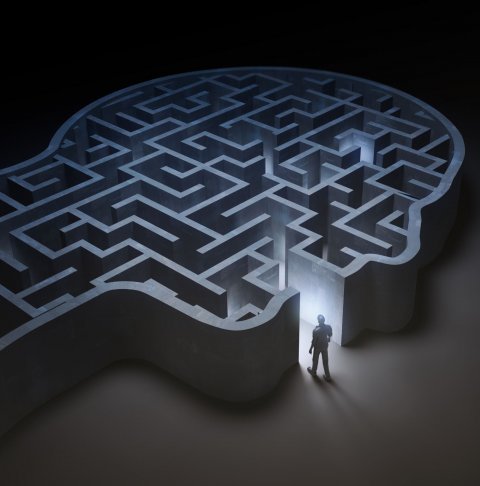
Image source: Shutterstock/Mopic
Patients with depression or psychosis rarely experience symptoms of purely one or the other illness. Historically, this has meant that mental health clinicians give a diagnosis of a ‘primary’ illness, but with secondary symptoms. Making an accurate diagnosis is a big challenge for clinicians and diagnoses often do not accurately reflect the complexity of individual experience or indeed neurobiology. Clinicians diagnosing psychosis, for example, would frequently regard depression as a secondary illness, with implications for treatment decisions which focus more on psychosis symptoms (e.g. hallucinations or delusions).
A team at the University of Birmingham’s Institute for Mental Health and Centre for Human Brain Health, working with researchers from the PRONIA consortium wanted to explore the possibility of using machine learning to create highly accurate models of ‘pure’ forms of both illnesses and to use these to investigate the diagnostic accuracy of a cohort of patients with mixed symptoms. Their results are published in Schizophrenia Bulletin. “The majority of patients have co-morbidities, so people with psychosis also have depressive symptoms and vice versa”, explains lead author Paris Alexandros Lalousis. “That presents a big challenge for clinicians in terms of diagnosing and then delivering treatments that are designed for patients without co-morbidity. It’s not that patients are misdiagnosed, but the current diagnostic categories we have do not accurately reflect the clinical and neurobiological reality”.
Our study highlights the need for clinicians to understand better the complex neurobiology of these conditions, and the role of ‘co-morbid’ symptoms; in particular considering carefully the role that depression is playing in the illness
Alexandros Lalousis
The researchers examined questionnaire responses, detailed clinical interviews and data from structural magnetic resonance imaging from a cohort of 300 patients taking part in the PRONIA study, a European Union-funded cohort study taking place across seven European research centres (www.pronia.eu). Within this cohort, the researchers identified small subgroups of patients who could be classified as suffering either from psychosis without any symptoms of depression, or from depression without any psychotic symptoms. Using this data, the team identified machine learning models of ‘pure’ depression, and ‘pure’ psychosis. They were then able to use machine learning methods to apply these models to patients with symptoms of both illnesses. The aim was to build a highly accurate disease profile for each patient and test that against their diagnosis to see how accurate it was.
The team found that, while patients with depression as a primary illness were more likely to be diagnosed accurately, patients with psychosis with depression had symptoms which most frequently tended towards the depression dimension. This may indicate that depression plays a greater part in the illness than had previously been thought. Mr Lalousis added: “There is a pressing need for better treatments for psychosis and depression, conditions which constitute a major mental health challenge worldwide. Our study highlights the need for clinicians to understand better the complex neurobiology of these conditions, and the role of ‘co-morbid’ symptoms; in particular considering carefully the role that depression is playing in the illness. In this study we have shown how using sophisticated machine learning algorithms which take into account clinical, neurocognitive, and neurobiological factors can aid our understanding of the complexity of mental illness. In the future, we think machine learning could become a critical tool for accurate diagnosis. We have a real opportunity to develop data-driven diagnostic methods – this is an area in which mental health is keeping pace with physical health and it’s really important that we keep up that momentum.”
Source: University of Birmingham
09.02.2021