News • From physical to computational staining
Deep learning accurately stains digital biopsy H&E slides
Tissue biopsy slides stained using hematoxylin and eosin (H&E) dyes are a cornerstone of histopathology, especially for pathologists needing to diagnose and determine the stage of cancers.
A research team led by scientists at the Massachusetts Institute of Technology (MIT) Media Lab, in collaboration with clinicians at Stanford University School of Medicine and Harvard Medical School, now shows that digital scans of these biopsy slides can be stained computationally, using deep learning algorithms trained on data from physically dyed slides.
The researchers published their study in JAMA Network.
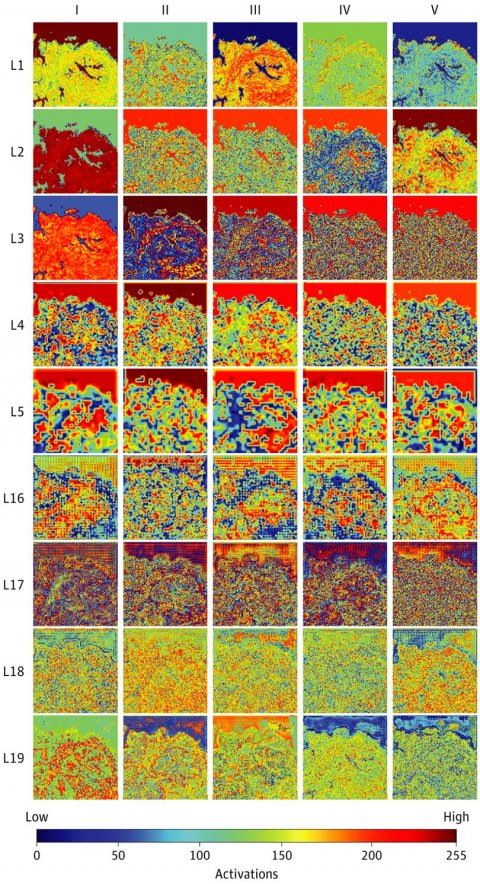
Pathologists who examined the computationally stained H&E slide images in a blind study could not tell them apart from traditionally stained slides while using them to accurately identify and grade prostate cancers. What’s more, the slides could also be computationally “de-stained” in a way that resets them to an original state for use in future studies, the researchers conclude.
This process of computational digital staining and de-staining preserves small amounts of tissue biopsied from cancer patients and allows researchers and clinicians to analyze slides for multiple kinds of diagnostic and prognostic tests, without needing to extract additional tissue sections. “Our development of a de-staining tool may allow us to vastly expand our capacity to perform research on millions of archived slides with known clinical outcome data,” says Alarice Lowe, an associate professor of pathology and director of the Circulating Tumor Cell Lab at Stanford University, who was a co-author on the paper. “The possibilities of applying this work and rigorously validating the findings are really limitless.”
The researchers also analyzed the steps by which the deep learning neural networks stained the slides, which is key for clinical translation of these deep learning systems, says Pratik Shah, MIT principal research scientist and the study’s senior author. “The problem is tissue, the solution is an algorithm, but we also need ratification of the results generated by these learning systems,” he says. “This provides explanation and validation of randomized clinical trials of deep learning models and their findings for clinical applications.”
Other MIT contributors are joint first author and technical associate Aman Rana (now at Amazon) and MIT postdoc Akram Bayat in Shah’s lab. Pathologists at Harvard Medical School, Brigham and Women's Hospital, Boston University School of Medicine, and Veterans Affairs Boston Healthcare provided clinical validation of the findings.
We’re not really just solving a staining problem, we’re also solving a save-the-tissue problem
Pratik Shah
To create computationally dyed slides, Shah and colleagues have been training deep neural networks, which learn by comparing digital image pairs of biopsy slides before and after H&E staining. It’s a task well-suited for neural networks, Shah said, “since they are quite powerful at learning a distribution and mapping of data in a manner that humans cannot learn well.” Shah calls the pairs “siblings,” noting that the process trains the network by showing them thousands of sibling pairs. After training, he said, the network only needs the “low-cost, and widely available easy-to-manage sibling,”— non-stained biopsy images—to generate new computationally H&E stained images, or the reverse where an H&E dye stained image is virtually de-stained.
In the current study, the researchers trained the network using 87,000 image patches (small sections of the entire digital images) scanned from biopsied prostate tissue from 38 men treated at Brigham and Women’s Hospital between 2014 and 2017. The tissues and the patients’ electronic health records were de-identified as part of the study.
When Shah and colleagues compared regular dye-stained and computationally stained images pixel by pixel, they found that the neural networks performed accurate virtual H&E staining, creating images that were 90-96 percent similar to the dyed versions. The deep learning algorithms could also reverse the process, de-staining computationally colored slides back to their original state with a similar degree of accuracy. “This work has shown that computer algorithms are able to reliably take unstained tissue and perform histochemical staining using H&E,” says Lowe, who said the process also “lays the groundwork” for using other stains and analytical methods that pathologists use regularly.
Computationally stained slides could help automate the time-consuming process of slide staining, but Shah said the ability to de-stain and preserve images for future use is the real advantage of the deep learning techniques. “We’re not really just solving a staining problem, we’re also solving a save-the-tissue problem,” he said.
As part of the study, four board-certified and trained expert pathologists labeled 13 sets of computationally stained and traditionally stained slides to identify and grade potential tumors. In the first round, two randomly selected pathologists were provided computationally stained images while H&E dye-stained images were given to the other two pathologists. After a period of four weeks, the image sets were swapped between the pathologists, and another round of annotations were conducted. There was a 95 percent overlap in the annotations made by the pathologists on the two sets of slides. “Human readers could not tell them apart,” says Shah.
The pathologists’ assessments from the computationally stained slides also agreed with majority of the initial clinical diagnoses included in the patient’s electronic health records. In two cases, the computationally stained images overturned the original diagnoses, the researchers found. “The fact that diagnoses with higher accuracy were able to be rendered on digitally stained images speaks to the high fidelity of the image quality,” Lowe says.
Another important part of the study involved using novel methods to visualize and explain how the neural networks assembled computationally stained and de-stained images. This was done by creating a pixel-by-pixel visualization and explanation of the process using activation maps of neural network models corresponding to tumors and other features used by clinicians for differential diagnoses. This type of analysis helps to create a verification process that is needed when evaluating “software as a medical device,” says Shah, who is working with the U.S. Food and Drug Administration on ways to regulate and translate computational medicine for clinical applications. “The question has been, how do we get this technology out to clinical settings for maximizing benefit to patients and physicians?” Shah says. “The process of getting this technology out involves all these steps: high quality data, computer science, model explanation and benchmarking performance, image visualization, and collaborating with clinicians for multiple rounds of evaluations.”
Source: Massachusetts Institute of Technology (MIT)
26.05.2020