News • mhealth
App accurately detects COVID-19 infection in people’s voices
Artificial intelligence (AI) can be used to detect COVID-19 infection in people’s voices by means of a mobile phone app, according to research to be presented at the European Respiratory Society International Congress in Barcelona, Spain.
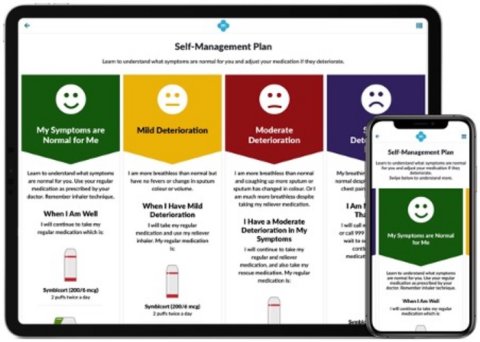
The AI model used in this research is more accurate than lateral flow/rapid antigen tests and is cheap, quick and easy to use, which means it can be used in low-income countries where PCR tests are expensive and/or difficult to distribute.
Ms Wafaa Aljbawi, a researcher at the Institute of Data Science, Maastricht University, The Netherlands, told the congress that the AI model was accurate 89% of the time, whereas the accuracy of lateral flow tests varied widely depending on the brand. Also, lateral flow tests were considerably less accurate at detecting COVID infection in people who showed no symptoms.
“These promising results suggest that simple voice recordings and fine-tuned AI algorithms can potentially achieve high precision in determining which patients have COVID-19 infection,” she said. “Such tests can be provided at no cost and are simple to interpret. Moreover, they enable remote, virtual testing and have a turnaround time of less than a minute. They could be used, for example, at the entry points for large gatherings, enabling rapid screening of the population.”
COVID-19 infection usually affects the upper respiratory track and vocal cords, leading to changes in a person’s voice. Ms Aljbawi and her supervisors, Dr Sami Simons, pulmonologist at Maastricht University Medical Centre, and Dr Visara Urovi, also from the Institute of Data Science, decided to investigate if it was possible to use AI to analyse voices in order to detect COVID-19.
They used data from the University of Cambridge’s crowd-sourcing COVID-19 Sounds App that contains 893 audio samples from 4,352 healthy and non-healthy participants, 308 of whom had tested positive for COVID-19. The app is installed on the user’s mobile phone, the participants report some basic information about demographics, medical history and smoking status, and then are asked to record some respiratory sounds. These include coughing three times, breathing deeply through their mouth three to five times, and reading a short sentence on the screen three times.
The researchers used a voice analysis technique called Mel-spectrogram analysis, which identifies different voice features such as loudness, power and variation over time.
“In this way we can decompose the many properties of the participants’ voices,” said Ms Aljbawi. “In order to distinguish the voice of COVID-19 patients from those who did not have the disease, we built different artificial intelligence models and evaluated which one worked best at classifying the COVID-19 cases.”
They found that one model called Long-Short Term Memory (LSTM) out-performed the other models. LSTM is based on neural networks, which mimic the way the human brain operates and recognises the underlying relationships in data. It works with sequences, which makes it suitable for modelling signals collected over time, such as from the voice, because of its ability to store data in its memory.
Its overall accuracy was 89%, its ability to correctly detect positive cases (the true positive rate or “sensitivity”) was 89%, and its ability to correctly identify negative cases (the true negative rate or “specificity”) was 83%.
“These results show a significant improvement in the accuracy of diagnosing COVID-19 compared to state-of-the-art tests such as the lateral flow test,” said Ms Aljbawi. “The lateral flow test has a sensitivity of only 56%, but a higher specificity rate of 99.5%. This is important as it signifies that the lateral flow test is misclassifying infected people as COVID-19 negative more often than our test. In other words, with the AI LSTM model, we could miss 11 out 100 cases who would go on to spread the infection, while the lateral flow test would miss 44 out of 100 cases.
“The high specificity of the lateral flow test means that only one in 100 people would be wrongly told they were COVID-19 positive when, in fact, they were not infected, while the LSTM test would wrongly diagnose 17 in 100 non-infected people as positive. However, since this test is virtually free, it is possible to invite people for PCR tests if the LSTM tests show they are positive.”
The researchers say that their results need to be validated with large numbers. Since the start of this project, 53,449 audio samples from 36,116 participants have now been collected and can be used to improve and validate the accuracy of the model. They are also carrying out further analysis to understand which parameters in the voice are influencing the AI model.
AI & COPD
In a second study, Mr Henry Glyde, a PhD student in the faculty of engineering at the University of Bristol, showed that AI could be harnessed via an app called myCOPD to predict when patients with chronic obstructive pulmonary disease (COPD) might suffer a flare-up of their disease, sometimes called acute exacerbation. COPD exacerbations can be very serious and are associated with increased risk of hospitalisation. Symptoms include shortness of breath, coughing and producing more phlegm (mucus).
“Acute exacerbations of COPD have poor outcomes. We know that early identification and treatment of exacerbations can improve these outcomes and so we wanted to determine the predictive ability of a widely used COPD app,” he said.
The myCOPD app is a cloud-based interactive app, developed by patients and clinicians and is available to use in the UK’s National Health Service. It was established in 2016 and, so far, has over 15,000 COPD patients using it to help them manage their disease.
The researchers collected 45,636 records for 183 patients between August 2017 and December 2021. Of these, 45,007 were records of stable disease and 629 were exacerbations. Exacerbation predictions were generated one to eight days before a self-reported exacerbation event. Mr Glyde and colleagues used these data to train AI models on 70% of the data and test it on 30%.
The patients were “high engagers”, who had been using the app weekly over months or even years to record their symptoms and other health information, record medication, set reminders, and have access to up-to-date health and lifestyle information. Doctors can assess the data via a clinician dashboard, enabling them to provide oversight, co-management and remote monitoring.
“The most recent AI model we developed has a sensitivity of 32% and a specificity of 95%. This means that the model is very good at telling patients when they are not about to experience an exacerbation, which may help them to avoid unnecessary treatment. It is less good at telling them when they are about to experience one. Improving this will be the focus of the next phase of our research,” said Mr Glyde.
Speaking before the congress, Dr James Dodd, Associate Professor in respiratory medicine at the University of Bristol and project lead, said: “To our knowledge, this study is the first of its kind to model real world data from COPD patients, extracted from a widely deployed therapeutic app. As a result, exacerbation predictive models generated from this study have the potential to be deployed to thousands more COPD patients after further safety and efficacy testing. It would empower patients to have more autonomy and control over their health. This is also a significant benefit for their doctors as such a system would likely reduce patient reliance on primary care. In addition, better-managed exacerbations could prevent hospitalisation and alleviate the burden on the healthcare system. Further study is required into patient engagement to determine what level of accuracy is acceptable and how an exacerbation alert system would work in practice. The introduction of sensing technologies may further enhance monitoring and improve the predictive performance of models.”
One of the limitations of the study is the small number of frequent users of the app. The current model requires a patient to input a COPD assessment test score, fill out their medication diary and then report they are having an exacerbation accurately days later. Usually, only patients who are highly engaged with the app, using it daily or weekly, can provide the amount of data needed for the AI modelling. In addition, because there are significantly more days the users are stable than when they are having an exacerbation, there is a significant imbalance between the exacerbation and non-exacerbation data available. This results in even further difficulty in the models correctly predicting events after training on this imbalanced data.
“A recent partnership between patients, clinicians and carers to set research priorities in COPD found that the highest-rated question was how to identify better ways to prevent exacerbations. We have focused on this question ,and we will be working closely with patients to design and implement the system,” concluded Mr Glyde.
Chair of the ERS Science Council, Professor Chris Brightling, is the National Institute for Health and Care Research (NIHR) Senior Investigator at the University of Leicester, UK, and was not involved with the research. He commented: “These two studies show the potential of artificial intelligence and apps on mobile phones and other digital devices to make a difference in how diseases are managed. Having more data available for training these artificial intelligence models, including appropriate control groups, as well as validation in multiple studies, will improve their accuracy and reliability. Digital health using AI models presents an exciting opportunity and is likely to impact future health care.”
Source: European Lung Foundation
06.09.2022