Article • A challenger arrives
AI – just a tool or the future of healthcare?
Neuroscientist Lynda Chin MD, Founder and CEO of Real-world Education Detection and Intervention, has little doubt: ‘Artificial intelligence to the rescue,’ she proclaimed in her keynote address at the Artificial Intelligence and Machine Learning Summit, held in Las Vegas this spring.
What is the equivalent of a residency programme for an AI programme?
Lynda Chin
‘We need a system and analytics to interpret data!’ she urged, despite being well aware that building a suitable algorithm, as well as training it to discover patterns and identify relationships, is a tedious task. ‘We train an artificial intelligence (AI) system just as we would train a doctor’, she explained and pondered, ‘What is the equivalent of a residency programme for an AI programme?’
Global interest in AI
Work to derive actionable insights from ever-increasing data is the next logical step. Hence, self-learning algorithms and their underlying neuronal networks have become a major focus in software development.
As the development of AI is highly correlated with the availability of data, healthcare attracts a lot of AI activities. According to a study by Roland Berger of the strategy consultants and human venture capital firm Asgard, ‘Artificial Intelligence – A strategy for European startups’, the healthcare and FinTech industries together, after B2B services, attract most AI start-ups on a European and global level. Nonetheless, have those undertakings in AI, machine learning (ML) and deep learning effected improvements in healthcare on a larger scale?
Solving real problems
Although AI has become a buzz word in the healthcare world, its use is still in early days. AI is accredited with revealing clinical and operational insights and contributing to spare patients from unnecessary procedures and worry. Some examples on how AI enhances diagnostics and improves disease management include:
Picking up illnesses earlier
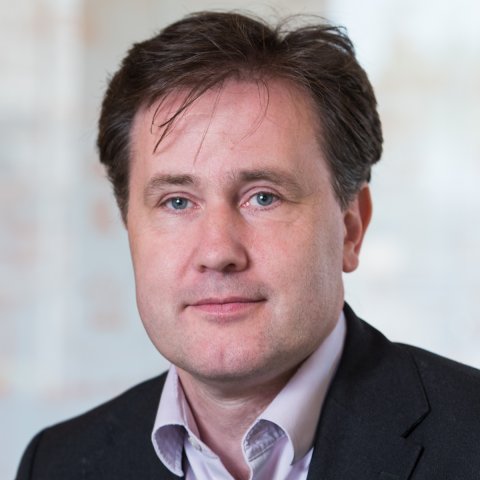
Ultromics, a new AI-based solution developed by Paul Leeson, Professor for Cardiovascular Medicine at the University of Oxford, diagnoses cardiac scans to determine a patient’s risk of an imminent cardiological problem. Currently, cardiologists can defer from the timing of the heartbeat in scans if there is an impending cardiological problem. However, they are wrong in one in five cases, leading to patients being discharged and suffering a heart attack at home or having unnecessary surgery.
To train Ultromics, Leeson used the scans of 1,000 patients he had treated over the past seven years, along with information about whether those patients continued having heart problems. The solution picks up details in the images doctors cannot see and gives recommendations as to whether a patient should be treated further. Ultromics has been tested in clinical trials in six cardiology units and, according to Leeson, the data indicates that the solution has greatly out-performed its fellow cardiologists. The trial results suggest further that Ultromics could save lives and spare patients unnecessary surgery, which Leeson reckons could save England’s NHS more than £300 million per year.
Another example is an AI/ML-based system by the British start-up Optellium, which addresses the management of patients with large clumps of cells – nodules – in the lungs. According to Optellium, up to 30% of all patients scanned have such small nodules of which the majority are harmless. However, radiologists often struggle to determine if a nodule is cancerous, leading to an indeterminate diagnosis that needs up to two years follow-up imaging to monitor potential growth and, in some cases, the performance of biopsies and surgeries.
The solution, the firm reports, ‘provides an objective risk score of nodule malignancy learned from a database of thousands of examples with known ground-truth diagnoses’, enabling clinicians to ‘confidently stratify lung nodule patients earlier, potentially on the basis of only one or two scans’. Optellium’s chief science and technology officer, Dr Timor Kadir, reports that trials of the system in Manchester imply that more than 4,000 lung cancer patients a year, in the UK alone, could be diagnosed much earlier and hence have a much greater chance of survival.
Radiomics on the radar
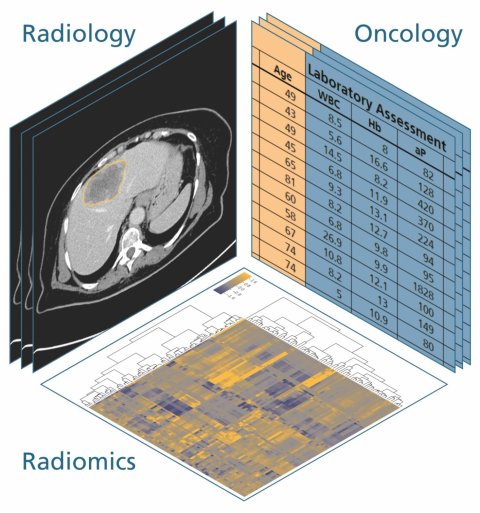
The Fraunhofer Institute for Medical Image Computing (MEVIS) and partners are deploying radiomics to discover relationships between image features and clinical data within the Patient-Oriented Oncological Therapy Assistance (PANTHER) project. The aim is to develop a clinical decision support system for physicians to help determine the best course of therapy (medication, radiation, or a mix of both) for patients suffering from cancer of the colon or lymphoma. This will allow benefits to be maximised and curtail the side effects of a chosen therapy in a hitherto uncharted way by adjusting the course of prescribed therapy early in the treatment.
Jan Hendrik Moltz PhD, Research Scientist at Fraunhofer MEVIS, explained: ‘So far, the size development of a tumour is the most important criterion in evaluating CT images. However, there is much more information hidden in those scans that has hardly been used.’ He refers to information on changes in the shape and texture of a tumour that occur during therapy, but that cannot be detected by physicians with their bare eyes. By deploying AI and deep learning on historical data from the Medical Centre of the University of Munich, it is hoped to reveal insights into the effectiveness of the therapy of bowel cancer and lymphoma.
Mid-term into the three-year-long project, Moltz reports good progress on homogenising the data, as well as segmenting computed tomography scans – segmenting refers to marking the structures to be analysed on the scans. ‘Analysing the spleen, for example, manually, is very time-consuming,’ Moltz explained. We have managed to develop a segmentation algorithm based on deep learning that is already so effective that it almost needs no further manual corrections. Automating the segmentation enables us to obtain outcomes much faster.’
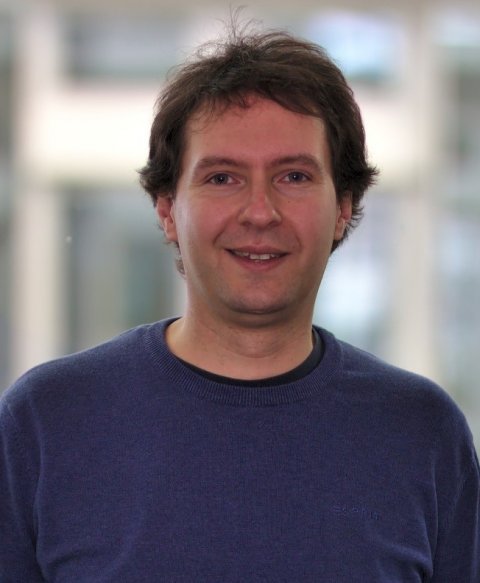
Mid-term into the three-year-long project, Moltz reports good progress on homogenising the data, as well as segmenting computed tomography scans – segmenting refers to marking the structures to be analysed on the scans. ‘Analysing the spleen, for example, manually, is very time-consuming,’ Moltz explained. We have managed to develop a segmentation algorithm based on deep learning that is already so effective that it almost needs no further manual corrections. Automating the segmentation enables us to obtain outcomes much faster.’
Last November, ‘Xiaoyi’, meaning ‘little doctor’, an AI-fed robot, successfully passed the medical approval test in China, even with above average results. Will this be the future? Chin shook her head: ‘Data analytics helps healthcare to be more efficient, but AI is just a tool. It needs clinical experts to understand the problem. Although computers will make therapy decisions, they will support clinicians, not replace them.’
01.09.2018