Courtesy of Professor M Shriver, Pennsylvania State University, and Dr Peter Claes, KU Leuven. Reprinted from Paul Suetens, Fundamentals of Medical Imaging 3rd edition, Cambridge University Press, 2017
Article • The revolution escalates
AI image analysis: Opportunity or threat?
New procedures in medical image analysis based on artificial intelligence (AI) offer numerous opportunities but still have their limitations.
Report: Michael Krassnitzer
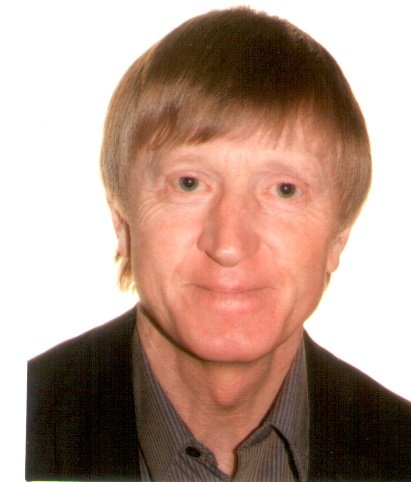
‘Is image computing an opportunity or a threat?’ asked Dr Paul Suetens, professor of Medical Imaging and Image Processing at University Hospital Leuven. During the recent European Radiology Congress 2018 held in Vienna he also provided his own answer: ‘It’s an opportunity if the radiologist takes advantage of this supporting technology. It’s a threat if it is discarded by the radiologist – “I am too busy now” are words I often hear; then it’s other specialists who are gratefully adopting this technology. Image Computing, including image analysis, artificial intelligence, artificial neural networks und deep learning, is starting a revolution,’ Suetens is convinced. Artificial Intelligence (AI) is not new – research in this field was carried out as far back as the 1950s – but, whilst in the early days AI learnt from image descriptions, it now learns directly from the images, such as photometric image characteristics.
Suetens was involved in a project that used MRI images with BOLD contrast – with the image signal depending on the oxygen content in red blood cells – for a detailed investigation into which areas of the brain are active during hearing and processing of language. Other types of image computing are based on geometric image characteristics, such as segmentation of thoracic images.
Detecting mutations via facial analysis
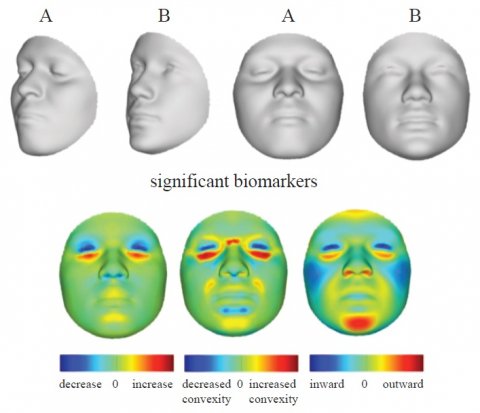
Image computing also includes the exciting field of image genetics, in which Suetens is also involved. As a member of an international research team, he linked a database containing 3-D facial images with genetic information. One of the results was that biomarkers, which point towards genetic mutations, were found in the image data. Certain characteristics of human faces suggest a mutation of the SLC35D1 gene, which is associated with chondrodysplasia with snail-like pelvis, a very rare, lethal form of skeletal dysplasia.
A further use of this link between facial images and genetic information is the reconstruction of faces from human DNA, making it possible for instance to reconstruct the features of well-known persons of whom, long after they have died, only artistic representations have been available. Respective reconstructions based on saliva samples from living test subjects achieve astonishing resemblances.
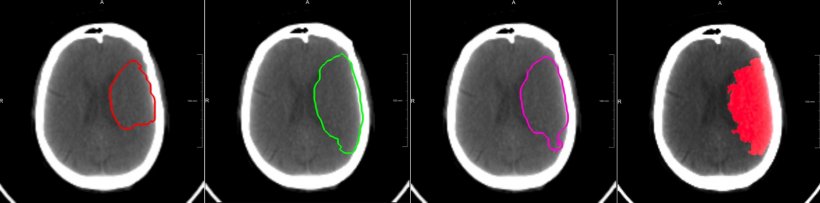
- 180 CT perfusion images in the acute phase.
- Y/N followed by an intra-arterial thrombectomy. About 50% of the training set received an endovascular treatment.
- The time between imaging and the end of the thrombectomy.
- Occlusion present Y/N.
- A follow-up CT scan after 5 days with a delineation of the final lesion.
Left three images: the prognosis showing three cases: (left) a complete reperfusion except for the core; (middle) no treatment, hence, the final lesion consists of core and penumbra; (right) predicted lesion after thrombectomy 3h after imaging with a presumed mTICI grade 2a.
Right: Follow-up scan after five days with the final lesion. Thrombectomy was performed 3h after imaging with mTICI grade 2a.
Courtesy of Dr David Robben, KU Leuven, and MR CLEAN Investigators
Deep Learning is still in its infancy
When we increase the number of nodes the results become worse – and we don’t yet know why this is
Paul Suetens
All these applications are based on deep learning. This entails an artificial system learning from examples and then recognising inherent patterns and regularities by itself. The basis of this are so-called artificial neural networks that are modelled on the workings of the human brain. ‘Deep learning is a new paradigm with a strong impact on medical image analysis. It is sufficiently accurate and fast to compete with the human expert for specific narrowly defined tasks,’ says Suetens.
However, deep learning still has its limitations. ‘Deep learning is still in its infancy,’ admits Suetens. If only a limited amount of data is available, or where the issue is around complex forms and deformations, neural networks do not function very well. ‘A neural network is nothing other than a large number of individual data processors which are linked with one another – comparable to neurons in the human brain,’ explains Suetens.
However, the human brain has around 86 billion nerve cells, whilst an artificial neural network only has 20 million nodes. ‘When we increase the number of nodes the results become worse – and we don’t yet know why this is,’ admits Suetens.
Profile:
Professor Paul Suetens heads the Division Image and Speech Processing in the Department of Electrical Engineering at Katholieke Universiteit (KU) Leuven, Belgium. He is also chairman of the Medical Imaging Research Centre at University Hospital Leuven. His research focuses on medical imaging and medical image computing, which methodologically lies in the domains of computational science and machine learning. He has authored more than 500 peer-reviewed papers in international journals and conference proceedings and is author of the book ‘Fundamentals of Medical Imaging’ (3 editions, 2002, 2009, 2017).
22.10.2018