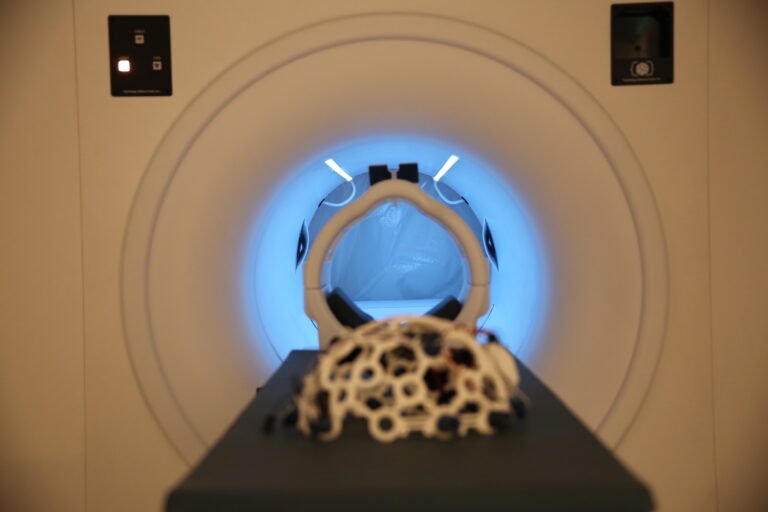
Image credits: INESC TEC
News • Deep learning, infrared and 3D videos
Using AI to classify epileptic seizures
A team of researchers at the Institute for Systems and Computer Engineering, Technology and Science (INESC TEC) and University of Munich, including Carnegie Mellon Portugal (CMU Portugal) Ph.D. student Tamás Karácsony, tested an innovative solution to classify seizures, the main symptom of epilepsy, using infrared radar and 3D videos.
Nature Scientific Reports recently published the results of this work, coordinated by Tamás Karácsony’s supervisor and CMU Portugal Scientific Director, João Paulo Cunha, researcher at INESC TEC and Professor at the Faculty of Engineering of the University of Porto (FEUP).
Despite a vast amount of video material available on seizure classification, studies on the subject are still rare and even more rare are approaches for automated, AI-supported solutions. This new study presents a new approach, which is the first to consider near-real time classification from two second samples, showing the feasibility of a system to support diagnosis and monitoring process (based on action recognition) that uses deep learning. This technique allows distinguishing between frontal and temporal lobes seizures (the two most common classes of epilepsy), or non-epileptic events.
[The approach takes] into account not only the presence of specific movements of interest in different parts of the patients’ bodies, but also their dynamics and their biomechanical aspects
Tamás Karácsony
Epilepsy is a chronic neurological disease that affects 1% of the world population, with seizures as one of the main symptoms – whose semiology is crucial to diagnose potential occurrences. The analysis of seizures is usually done using 2D video-EEG (electroencephalogram) at epilepsy monitoring units (EMUs), by specialized healthcare professionals. “During clinical diagnosis, the clinicians use these videos to visually recognize movements of interests defined by movement features (semiology)”, explained Tamás Karácsony, researcher at INESC TEC and CMU Portugal Ph.D. Student at FEUP.
However, the semiology assessment is limited by a high inter-rater variability among said professionals, and despite being promising, the automatic and semi-automatic approaches using computer vision still depend on considerable “human in the loop” effort. “A patient is usually monitored for several days, which has to be fully reviewed afterwards for the seizures. This requires a lot of time and effort from the clinical staff”, added the researcher.
To overcome this, the team of researchers has developed a deep learning-based approach for the automatic and near real-time classification of epileptic seizures. According to the paper’s first author, Tamás Karácsony, ”We present a new contribution inspired by the way experts analyze the semiology of seizures, taking into account not only the presence of specific movements of interest in different parts of the patients’ bodies, but also their dynamics and their biomechanical aspects, such as speed or acceleration patterns, or range of motion.”
The team turned to the world’s largest 3D video-EEG database, and extracted videos of 115 seizures, first developing a semi-specialised and automatic pre-processing algorithm to remove unnecessary environments from the videos. In practical terms, two image cropping methods are combined – depth and Mask R-CNN –, providing a clean scenario and, consequently, improving the extraction of relevant information from the available videos, minimizing unrelated variations, and improving the seizure classification process.
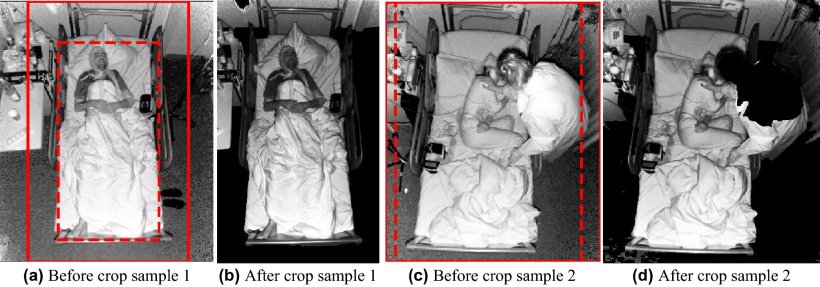
Image source: Karácsony et al., Scientific Reports 2022 (CC BY 4.0)
In a further explanation about the process used, Tamás explained “Our solution uses an action recognition approach with an intelligent 3D cropping of the scene to remove unrelated information, such as clinicians moving around the patients. By removing it, our method significantly improved classification performance”. According to the CMU Portugal Ph.D. candidate, this research has also proven the feasibility of our action-recognition approach to distinguish two classes of epilepsy and the non-epileptic class, with only two seconds of samples, making it useful for near-real-time monitoring. In addition, the solution we propose can be used in other datasets of 3D video for analysis and monitoring of seizures”
Therefore, in translating this knowledge to improved diagnosis and treatment, the approach serves two purposes: “it can be used for monitoring and alarms – which can alarm staff; or, if the approach is transferred to an ambulatory setting, a caregiver, when a seizure is ongoing, resulting in a faster response, which might decrease associated risks and Sudden Unexpected Death in Epilepsy (SUDEP). Without a near real-time approach this would not be feasible”, said Tamás Karácsony.
More research is required before this system can be implemented in clinical practice. Nevertheless, in the long-term the system is expected to benefit the clinicians, clinics, and patients. “With automated diagnosis support, the clinicians have to spend less time reviewing the videos, thus can treat more patients, hopefully make better decisions, which reduces associated costs (material and health) for clinics and society”, he concluded.
Source: Carnegie Mellon Portugal
05.01.2023