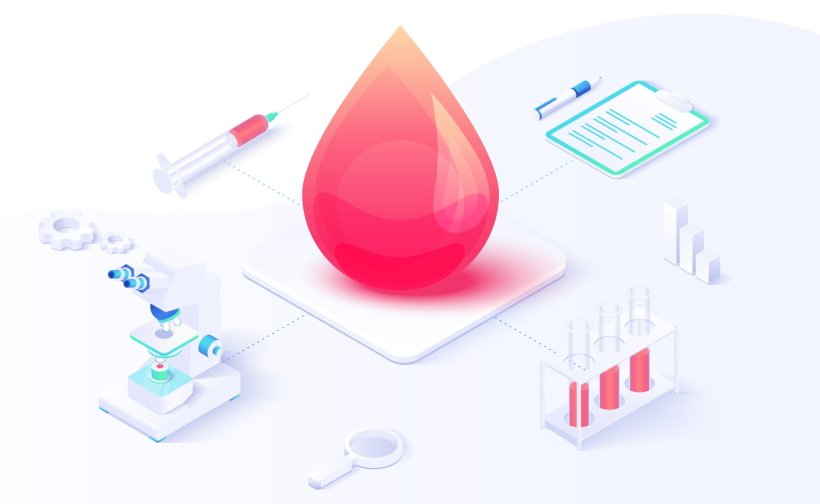
Image source: Adobe Stock/Graf Vishenka
News • Presented at AACC 2023
AI to predict multiple sclerosis, detect contaminated lab samples
A new artificial intelligence model can predict people’s risk of multiple sclerosis years before diagnosis, potentially enabling quicker treatment, according to research revealed at the 2023 AACC Annual Scientific Meeting & Clinical Lab Expo. Another breaking study demonstrates how machine learning can help detect lab samples contaminated with intravenous fluids—a finding that could reduce lab errors that delay diagnosis and raise healthcare costs.
Taken together, the results highlight key advances in the use of artificial intelligence and machine learning to improve patient care.
AI model harnesses patient data to predict MS risk
Multiple sclerosis is a disease of the nervous system that affects more than 2.8 million people worldwide. Scientists don’t fully understand what causes the condition but link its development to autoimmunity—when a person’s immune system mistakenly damages healthy cells—along with genetics, Epstein-Barr virus, and other factors. Currently, clinicians diagnose multiple sclerosis with imaging, studies of cerebrospinal fluid, and clinical history. But early-detection methods are desired because they could help patients start treatment sooner and slow the disease’s progression.
Our model’s performance suggests that AI-based prediction models could identify the risk for multiple sclerosis years before neurological symptoms appear
Raj Gopalan
A team led by Raj Gopalan, MD, at Siemens Healthineers in Tarrytown, New York, trained machine-learning models to predict people’s risk of multiple sclerosis. They harnessed more than 3,000 sets of data from the electronic health records of patients with and without multiple sclerosis from Beth Israel Deaconess Medical Center in Boston. Their “random forest model” parses data on a patient’s age, gender, blood markers, and metabolic data, gathered up to three years before diagnosis.
The model was highly accurate and demonstrated strong predictive power. Most of the model’s ability to distinguish at-risk patients came from blood measurements of neutrophils, red blood cells, and other markers. These predictions remained consistent for as long as three years before the patients were diagnosed.
“Our model’s performance suggests that AI-based prediction models could identify the risk for multiple sclerosis years before neurological symptoms appear,” Gopalan said. “This could reveal which patients should be monitored for periodic neurological and cognitive exams when symptoms appear,” he said. “In addition, early confirmation of the diagnosis with imaging and cerebrospinal fluid studies could facilitate disease-modifying treatment.”
Recommended article
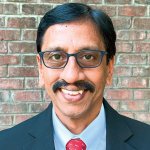
Sponsored • Earlier disease prediction and identification
The potential of AI in routine blood testing
It’s widely known that more than 70% of today’s medical decisions involve the results of laboratory tests, but the insights clinicians derive from these tests today may only be scratching the surface of their potential.
Machine learning flags potentially contaminated lab results
Scientists are also applying machine learning to uncover potential contamination in lab samples that could skew test results. When a sample is collected directly from intravenous (IV) catheters instead of a fresh blood draw, the fluid within the catheter can lead to erroneous lab results that slow diagnosis, raise healthcare costs, and lead to improper treatments. Current methods to detect contaminated samples aren’t always accurate and require technicians to perform labor-intensive manual analysis.
While this won’t immediately reduce the number of contaminated tests, it will hopefully substantially reduce the operational and clinical impact of these events when they do happen
Nicholas Spies
Using a technique called “mixture-of-experts” modeling, researchers have now created a machine-learning pipeline that can identify cases of IV fluid contamination that went undetected by manual methods. The team, led by Nicholas Spies, MD, at Washington University School of Medicine in St. Louis, aggregated more than 9,600,000 chemistry results from patients and simulated IV fluid contamination in some of the samples with common IV solutions. After training various machine-learning models using the simulated results, the scientists generated a final set of predictions.
The models detected meaningful contamination in several thousand samples. Spies said that the pipeline can detect about 5 to 10 times as many contaminated specimens as his group’s current approach. Furthermore, many of these tests evaded being previously flagged with manual methods–up to 94% in the case of samples contaminated with a solution called lactated Ringer’s.
“While this won’t immediately reduce the number of contaminated tests, it will hopefully substantially reduce the operational and clinical impact of these events when they do happen, and provide us with a better quality metric with which we can prioritize areas for improvement initiatives,” Spies said.
Source: Association for Diagnostics & Laboratory Medicine
13.08.2023