Sponsored • Earlier disease prediction and identification
The potential of AI in routine blood testing
By Dr Raj Gopalan
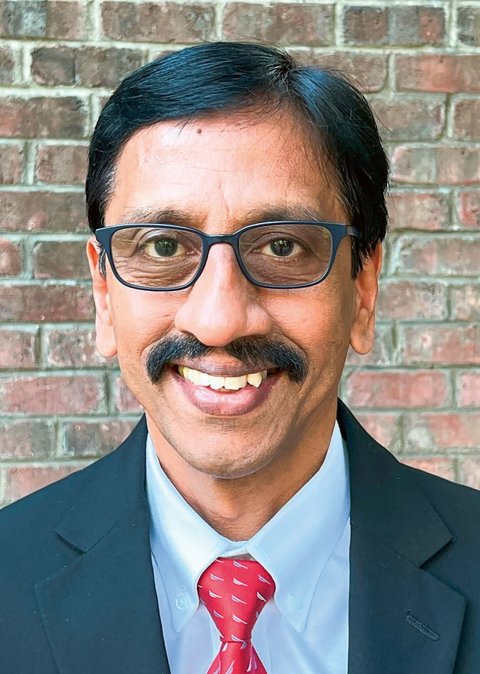
It’s widely known that more than 70% of today’s medical decisions involve the results of laboratory tests1, but the insights clinicians derive from these tests today may only be scratching the surface of their potential. Routine blood tests may also offer opportunities for earlier identification of patients at risk for complex diseases such as cancer, other silent chronic diseases, acute life-threatening infections, and severe Covid-19.
Clinical laboratories have transformed over the years, becoming more efficient, accurate, and consistent. These advances have helped clinicians to better manage large volumes of lab test results, enabling them to more quickly identify results that require further review. The lab’s role in offering decision support to physicians, predominantly, has not expanded much beyond providing basic reference intervals and highlighting the results that fall outside of those intervals.2
The development and implementation of more nuanced clinical decision support in the laboratory remains a tremendous opportunity with the potential to help identify risk of diseases sooner and ultimately support physicians in enhanced triage, advanced testing, and management of patients’ conditions.
Clinical decision support (CDS) as a potential aid in patient management and triage protocols
The biochemical process of any disease is a complex mechanism, and the early signs of many diseases are often evident by changes in blood parameters that occur even before the first symptoms appear. Combined changes in the parameters of commonly ordered routine blood panels may offer clues to underlying disease processes, in order to provide an opportunity for clinicians to consider specific additional tests to confirm or rule out potential diseases.
With the help of artificial intelligence (AI) and machine learning (ML) technologies, it may be possible, for example, to develop and implement algorithms that automatically compare the results of various defined analytes and aggregate that data with the patient’s age and gender (or other dimensions) to generate more advanced insight into a patient’s condition. Taking this example a step further, such an advanced algorithm could potentially generate disease-specific likelihood scores to help the clinician focus on the most probable potential diagnoses or further inform the probability of risks for specific diseases.
The increasing prevalence of widespread cancers, progressive chronic diseases, and acute infections underscores the need for such models. It isn’t long ago that various countries were forced to grapple with the awful decisions of how best to triage thousands of Covid-19 patients flooding hospital EDs and ICUs in the face of inadequate supplies and limited clinical care space. It was clear that physicians and hospitals could have then benefited from advanced tools that provided likelihood-based information based on objective clinical data, namely lab test results.
Testing the theory with the Atellica Covid-19 Severity Algorithm
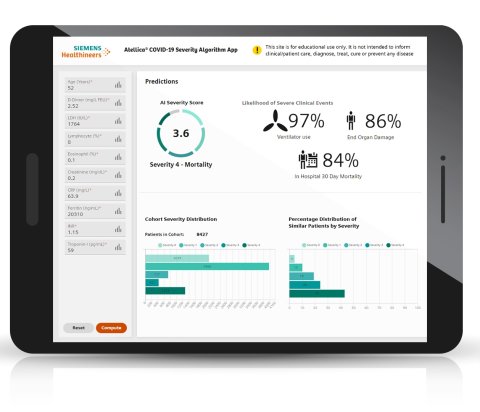
The Atellica Covid-19 Severity Algorithm* is Siemens Healthineers first educational-use-only, AI-based predictive model. The algorithm was developed to help clinicians predict the likelihood of individual patient progression to acute respiratory failure, end-organ dysfunction, and in-hospital 30-day mortality. Combining data from nine specific lab parameters, the model was developed with retrospective data from more than 14,500 Covid-19 patients from research partners at Houston Methodist Hospital (TX, U.S.), Emory University Healthcare (GA, U.S.), and La Paz University Hospital (Spain). Research findings demonstrated that the algorithm was able to predict patient outcomes with good accuracy, even when Delta was largely supplanted by Omicron, and was also a reliable predictor of outcomes in individuals with and without vaccinations.3
Exploring the potential role of AI-based CDS in early identification of cancer and other complex diseases
European residents represent about one-tenth of the global population, yet 1 in 4 of all cancer diagnoses occur in this region. Breast, prostate, lung, and colorectal cancers account for half of all cancers there.4 Liver disease is a leading cause of years of working life lost in Europe.5 As we enter a post-Covid-19 era, we are expanding our AI-based predictive model development with a view to supporting clinicians grappling with other prominent health burdens, namely hepatocellular, lung, and colon cancer; liver disease; chronic kidney disease; and sepsis. We anticipate that this could, for example, enable primary care physicians at outpatient clinics and emergency physicians to earlier identify patients at risk of these life-threatening conditions and support more timely referrals to specialists for special blood marker testing or advanced imaging. We are consciously working on developing these algorithms based on data from routine blood tests with the view to the algorithms also supporting the needs of patient populations lacking access to advanced diagnostics for early detection due to socioeconomic status, lack of insurance for preventive care, or lack of access to care.
After seeing firsthand how seamlessly we were able to integrate the predictive model into our daily laboratory workflow with Atellica Data Manager, I’m confident the algorithm can become an integrated decision support tool that will expand the lab’s contribution to physicians and ultimately aid in critical decision making for enhanced patient care
Dr Antonio Buño, La Paz University Hospital
While we believe that CDS creates a significant opportunity to help clinicians rule in or rule out potential candidates for diagnosis, we are mindful that its success will to some degree also depend on proper integration into the existing physician lab test order/result review workflow. This integration comes with its own set of unique challenges, but we believe that it is possible to overcome them by utilizing laboratory middleware such as Atellica Data Manager as a liaison between the Electronic Medical Record (EMR) and the analyzers. This scenario was tested in connection with our feasibility research conducted for the Atellica Covid-19 Severity Algorithm.
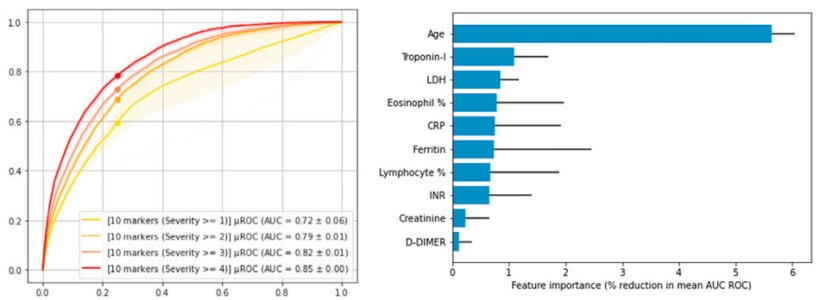
In closing, AI/ML have immense potential to help identify at-risk patients early in disease processes through routine blood examinations. While more research is needed, early findings suggest that predictive models may potentially support a clinician’s interpretation of lab test results to aid in the earlier diagnosis and management of patients and stratification of risk.
References:
- Strengthening clinical laboratories. Centers for Disease Control and Prevention. Accessed on 8/29/2022.
- Artificial intelligence in pathology and laboratory medicine. TS Pillay. Journal of Clinical Pathology, 2021 – jcp.bmj.com. Accessed on 8/29/2022.
- A deep learning approach for predicting severity of COVID-19 patients using a parsimonious set of laboratory markers. V Singh, R Kamaleswaran, D Chalfin, A Buño-Soto… – Iscience, 2021 – Elsevier. Accessed on 8/29/2022.
- Breast, prostate, lung, and colorectal cancers represent over half of all cancer diagnoses in Europe. The Cancer Atlas. Accessed on 8/29/2022.
- Liver disease in Europe. The Lancet. Accessed on 8/29/2022.
* The Atellica Severity Algorithm is intended for educational purposes only. It is not for clinical or patient care, diagnosis, treatment, or to cure or prevent any disease.
06.10.2022
- AI (716)
- blood (314)
- coronavirus (707)
- IT (886)
- Labbook (54)
- laboratory (1078)
- machine learning (162)