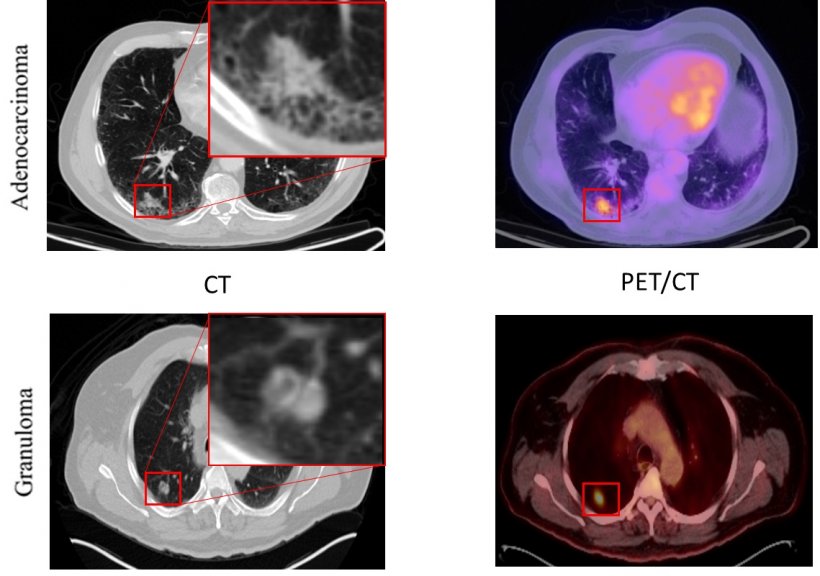
Article • Radiomics
A boost for thoracic radiology
A new radiomics study could help unlock one of the more challenging issues facing thoracic radiologists.
Report: Mark Nicholls
Distinguishing non-small cell lung cancer from benign nodules is a major challenge due to their similar appearance on CT images. Now, however, researchers from Case Western Reserve University in Cleveland, Ohio, have used radiomic features extracted from CT images to differentiate between these two pathologic conditions. Researcher Niha Beig explained that previous work on computer aided diagnosis (CAD) of lung cancer on CT, using radiomics, focused on investigating the suspicious lung nodule alone, but the retrospective study evaluated the computer-extracted (radiomic) features of the nodule in question as well as an immediate region of the lung parenchyma outside the nodule. It is known that tumour-infiltrating lymphocytes and tumour-associated stromal macrophages in the stroma around tumour is associated with the likelihood of malignancy, but her study team wanted to explore the idea of potentially capturing these patterns on CT imaging, via radiomics.
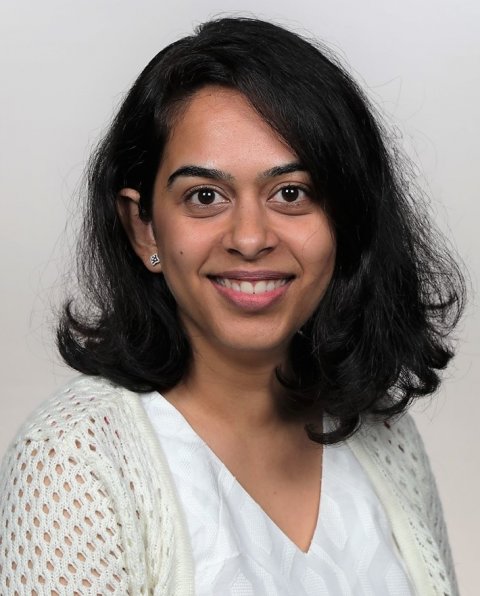
The study demonstrated that a combination of radiomic patterns of heterogeneity within and outside the tumour could distinguish benign nodules from non-small cell lung cancer on CT scans with 80% accuracy. On the same test set, an accuracy of 75% was obtained when they assessed only the nodule by itself. Beig said the main emphasis of the work is (1) a lot of informative signal is present outside the nodule, potentially capturing the malignant micro-environment of a tumour, and (2) combing the radiomic features from this region outside the suspicious nodule with the nodule itself can help build more robust CAD systems.
To reach their conclusion, the researchers developed a supervised machine learning pipeline and a dataset consisting of 290 patients (145 were benign granulomas, and the rest were malignant non-small cell lung cancer nodules). Within the training subset of 145 cases (with equal representation of two classes), radiomic features were extracted from the nodule and the region immediate to the lung parenchyma around the nodule. Following feature selection, a support vector machine (SVM) classifier was built and validated on an independent test set the remaining 145 cases. ‘Using the performance metrics of accuracy, sensitivity and specificity we concluded that radiomic features from inside the nodule and immediately outside the nodule can differentiate malignant tumours from benign nodules,’ Beig said.
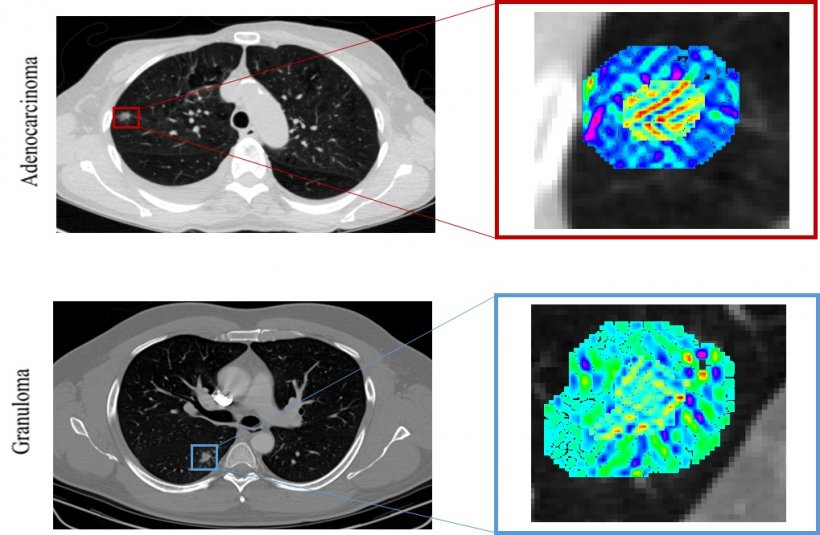
Another important aspect of this study was the qualitative assessment of representative patient histology in an attempt to unravel the morphometric and biological basis for the most predictive radiomic features. ‘We found that the immediate vicinity of 5mm outside the tumour had a unique radiomic signature in adenocarcinomas. We hypothesise that this densely-packed stromal tumour-infiltrating lymphocytes around adenocarcinomas manifest as smooth texture on CT images and potentially results in this distinctive radiomic signature.’
Given that differentiating the two pathologic conditions of non-small cell lung cancer and benign nodules - due to their similar appearance on CT images – is recognised as one of the most challenging issues faced by thoracic radiologists, she explained the significance of these findings. ‘Adenocarcinomas are the most prevalent subtype of non-small cell lung cancer, making it the most common true-positive finding in a given non-contrast lung cancer screening population. Granulomas represent the most common and possibly most confounding false-positive finding,’ Beig explained. ‘Given the similar appearance of these two pathologic conditions on imaging, a CAD based approach to this pressing clinical question is of immense interest. Our study found that CT imaging can be leveraged by using artificial intelligence algorithms to capture sub-visual textural clues of the tumour biology that can’t be appreciated by the naked human eye of an expert.’ She added that findings suggest a significant wealth of malignancy-related information in the peritumoral region of the tumour, such as angiogenic activity that manifests within the peritumoral region on CT imaging.
Our technology can be translated as a simple and inexpensive diagnosis tool, compared to an existing CAT scan, to determine whether a patient needs more invasive and expensive procedures or not
Niha Beig
Beig believes the findings could have an impact on future clinical practice. ‘I think the very context of integrating AI in healthcare is to aid better diagnosis and personalise treatments for prolonged survival in cancer patients. Our work represents a preliminary success for better diagnosis of malignant findings in a given lung cancer screening population. We started with the intention of developing a CAD tool that can reduce the number of interventions and unnecessary imaging needed to confirm diagnosis. We still hope that this technology will have a positive impact on the future clinical practice, and be used for this purpose.’
When implemented in clinical practice, the technology will potentially aid clinicians/radiologists to confirm diagnosis and subsequently make informed treatment decisions for example, whether a biopsy is required or not, as well as to improve the patient management protocol. Beyond that, patient benefits are also significant. The National Lung Screening Trial in the USA showed that 95% of incidental findings on CT are benign, and a majority represent benign granulomas, but many patients still undergo surgical intervention e.g. a biopsy, bronchoscopy or surgical wedge resection for histopathologic confirmation of presence or absence of a malignancy, or multiple CT scans for continued evaluation of the nodule. ‘Recognising that there is an unmet need for decision support tools for analysis, our work would be a far better tool,’ Beig affirmed. ‘Our technology can be translated as a simple and inexpensive diagnosis tool, compared to an existing CAT scan, to determine whether a patient needs more invasive and expensive procedures or not.’ However, she acknowledged that further work needs to be done before its realisation as a cancer screening tool to integrate into clinical practice in terms of validation, planning and more comprehensive analysis.
Profile:
Niha Beig is a third year PhD student in the biomedical engineering department at Case Western Reserve University, Cleveland Ohio, USA, where she works under the guidance of Professor Anant Madabhushi, the principal investigator and scientific guarantor of this study and director of the university’s Center for Computational Imaging and Personalised Diagnostics (CCIPD), where this study was conducted.
26.02.2019