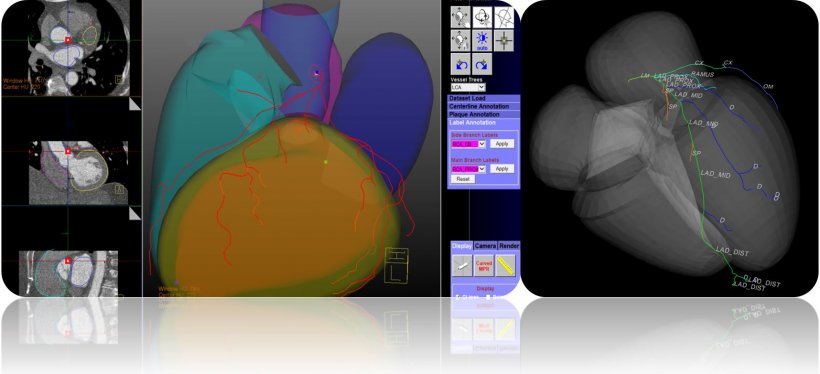
Article • 4D imaging, structured reporting and more
The ascent of AI in thorax-CT
As a highly innovative medical specialisation, radiology increasingly takes advantage of the possibilities offered by artificial intelligence (AI). Yet, there is no risk for the future of the specialty. Demands on this discipline are too complex, too communicative.
Thus, machines will not replace a radiologist any time soon – of that Uwe Joseph Schöpf, professor of radiology, cardiology and paediatrics, and director of the Cardiovascular Imaging department at the Medical University of South Carolina (USA), is convinced. Using a few impressive examples, he explains what AI has to offer the radiologists.
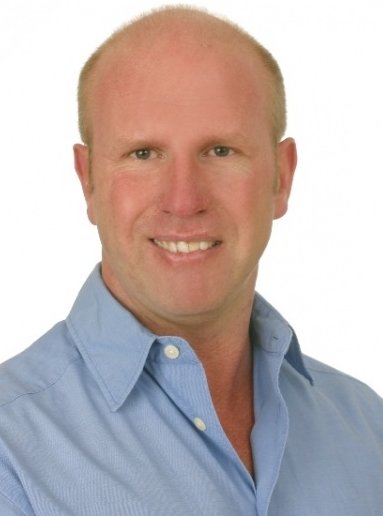
It is true that the popularity of radiology declined in recent years among young medical graduates in the USA. There was just too much worry about entering a specialty that might just be made obsolete by progress in artificial intelligence. Meanwhile the trend has reversed again, simply because that is not expected to happen.
The reason? There are far too many indispensable interpersonal competencies needed for success in radiology: communication with a patient and referring physicians; interdisciplinary procedures and a holistic view of the patient’s current situation – not just of a specific image pattern. ‘These are capabilities that a computer still cannot assume, no matter how good it is,’ Schöpf points out. ‘For that we still need capable and well-educated specialists.’
AI undertakes the time-consuming work
For the professor, AI constitutes a highly potent capacity to seriously improve the diagnosis using a continuously perfected image evaluation. What does this really mean? First, there is the enormous time-saving AI provides – above all for measurements in quantitative imaging. Thus, an appropriately trained computer can automatically and precisely generate the Agatson score for calcification of coronary arteries within seconds. The same measurement takes between 15 and 20 minutes for a radiology trainee or specialist depending on the degree of calcification.
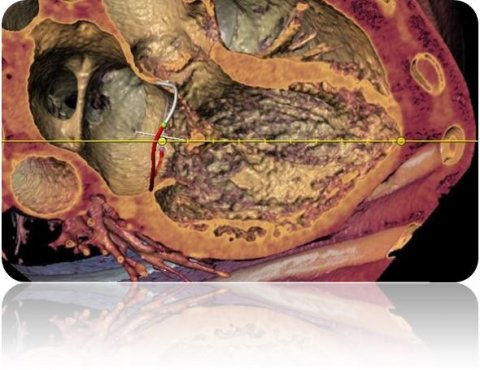
Another example is the automatic measurement of mitral valve dimensions. ‘This is difficult for the human observer, because it moves in a three-dimensional space; even four-dimensional if heartbeat is considered.
The 2-D image on the workstation is not well-suited to this kind of precise measurement.’ The computer recognises no such restrictions. It measures automatically, fast and probably also better.
Genuine value added
That is not all AI can do. It can enrich findings with additional results and thus enhance them. So-called opportunistic screening delivers important information which is not sought routinely but can decisively improve diagnosis. A good example is emphysema quantification. The computer recognises, on its own, whether or not there is a pulmonary emphysema and can even determine its extension simultaneously. Without any further effort, this measurement is taken automatically and precisely with every thoracic CT. The same applies to measuring bone density. Detailed information about bone substance can be highly relevant for diagnostic and therapeutic aspects. A human observer, concentrating on other thoracic structures, easily overlooks this irregularity.
The measurement of the thoracic aorta to find an aortic aneurysm is a third example. Due to numerous curves in the course of the aorta, measurement is difficult for radiologists – but no problem for the computer; here it also measures quickly and accurately.
Two birds with one stone
‘On one hand, we are currently working on the discovery of pulmonary nodules and, on the other, the automatic detection of coronary vessels and plaques.’ Here, the long-term objective is to identify as many potential problem areas as possible with one and the same CT image. That means, with a lung CT, calcification of coronary vessels are automatically measured in parallel and, with a heart CT, there is simultaneous detection of pulmonary nodes.
‘A very efficient procedure, impossible without AI,’ Schöpf observes. ‘We also work with AI especially in cardiovascular diagnosis to assess how serious a coronary stenosis is.’ The focus here is measurement of the flow reserve. Results to date indicate that this examination could become part of clinical routine very soon.
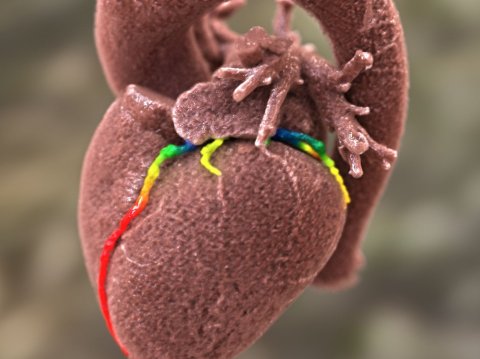
The use of AI is very promising – even beyond image identification. Catchwords: structured reporting. Based on unstructured findings dictated by a physician, AI allows filtering of the relevant text elements and their transfer to a structured diagnosis. The advantage is obvious: more objective diagnosis, easier billing, better use in studies and also easier to understand for a referring physician. ‘I see the value of structured diagnosis, but,’ Schöpf points out, ‘also the problem of practical implementation. The preparation of a structured diagnosis takes longer than one freely dictated. The physician has to click laboriously through web pages, in which all possible structures are queried, even the unobtrusive ones.’ From his experience he sees it as not particularly user-friendly for routine radiological operations. Hence the very promising use of the computer is even more welcome here. Moreover, the procedure can be applied to all organ fields, once the computer has been appropriately trained.
Profile:
Professor Uwe J Schöpf studied medicine in Munich and specialist training at the Institute for Clinical Radiology of the Ludwig-Maximilian-University. In 2001, with a keen interest in cardio-thoracic imaging, he left Bavaria for the USA where, he was to become a radiologist at the Brigham & Women’s Hospital, Massachusetts, until 2004, Today he is professor of radiology, cardiology and paediatrics and directs the cardiovascular imaging department at the Medical University of South Carolina in Charleston.
11.03.2020