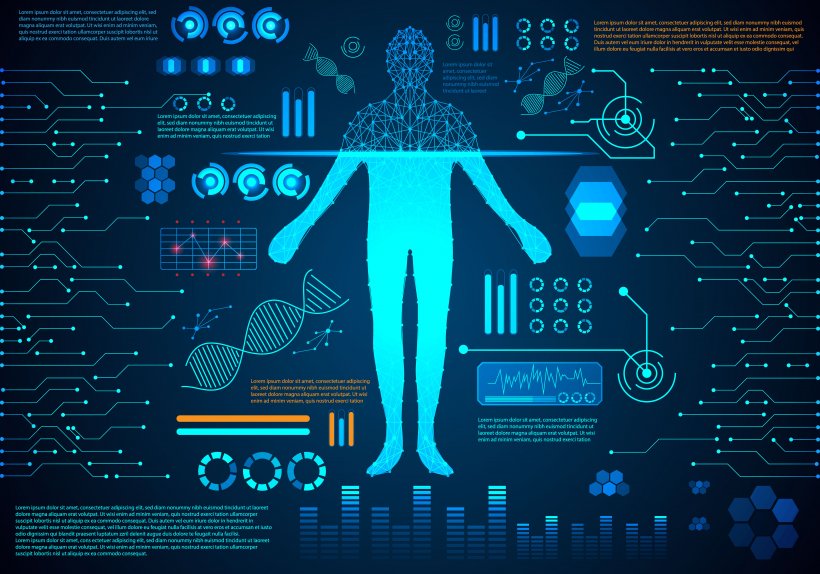
© Shutterstock/Tex vector
Article • Artificial intelligence
Superlative future assistance
As a member of the European Congress of Radiology (ECR) planning committee Professor Elmar Kotter suffered no serious challenge in pinpointing subject matter for the IT sessions. More than 300 submissions were received on artificial intelligence (AI).
Report: Daniela Zimmermann
AI applications to detect lesions or fractures in various contexts are now almost standard. An artificial neural network is trained using an annotated data set. The underlying technology is established and mostly independent of the specific localisation. ‘Image recognition is obviously an important and invaluable application, but the range for the utilisation of AI is much broader than that,’ Kotter points out.
Needs-based resource planning
‘By comparison, I believe that using AI to predict increased patient numbers, for instance, is much more innovative and exciting.’ Once this is established in hospital routine, it could be used in many areas. ‘It could develop into a helpful tool to improve needs-based utilisation of resources without increasing pressure on staff or equipment,’ Kotter believes. The main benefit of AI he sees is primarily in process optimisation - not only in radiology but across an entire hospital. However, he believes a long time will pass before AI can deliver diagnoses autonomously.
Optimisation of exam protocols
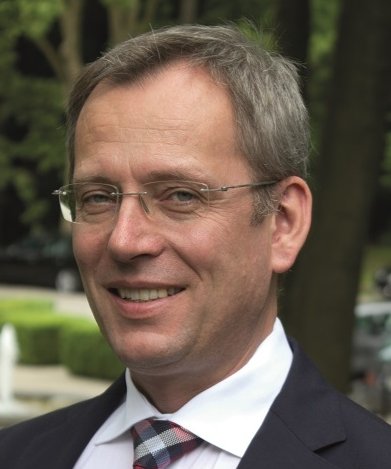
A fundamental problem that affects the optimisation of examination protocols is the inconsistency of exam descriptions, beginning with a request for a specific examination (termed ‘order entry’). Because the exam description is later transferred into the radiology information system, the description must be identical in both systems – the only way to ensure that the procedure requested is clearly defined and that the correct protocol is retrieved on the equipment used.
The situation is aggravated because hospitals use devices from many different manufacturers that use their own descriptions. Currently, to help visualise this clearly, extensive manual mapping is being carried out to align the different descriptions in the systems. ‘To give you an example,’ says Kotter, ‘here in Freiburg we work with 13,000 different examination descriptions.’
The dose management system at the end of the process must merge the different descriptions in identical categories before the data can be analysed – e.g. which dose was used, which outliers were observed etc. The approach of an AI-controlled process could be to automatically recognise what was examined based on images, for example, whether a procedure was a cardio CT or a standard abdominal exam. ‘This AI-controlled process could really help us to avoid inconsistent descriptions and their manual alignment,’ Kotter reasons.
Recommended article
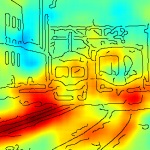
News • Machine learning
How intelligent is Artificial Intelligence?
Scientists put AI systems to the test und provide a glimpse into the diverse “intelligence” spectrum observed in current AI models.
An ideal relationship between image quality and dose
A further area of AI application is image quality recognition. Generally the higher the dose, the better the image. However, the challenge is to find the lowest possible dose that can be used to produce the best possible image and therefore the best diagnosis.
This needs the automatic evaluation of image quality for any given problem for each individual examination, which can then be correlated with the dose used. ‘This is a typical case for AI,’ says Kotter –unfortunately no one is working on this yet.
Conquering the information onslaught
Certain problems can only be analysed with the help of very high information density. At some point, humans reach their limits, with the evaluation of multiparametric MRI data for instance, and certainly when genomics data is included in the diagnostic process. ‘In my view,’ Kotter is convinced, ‘AI-controlled processes are predestined to conquer these enormous amounts of data.’
The hospital discharge letter provide another example. Depending on individual patients, it is not unusual to see twenty pages of discharge documentation – a real time problem for radiologists.
‘It would be extremely helpful if artificial intelligence could extract relevant patient data in an intelligent way, supplement it with other information (such as pathology data), and then evaluate and present it intelligently,’ Kotter suggests, concluding: ‘I imagine AI as a kind of superlative, executive assistant.’
Profile:
Dr Elmar Kotter studied Medicine and Computer Science at University of Montpellier (France) and Université René Descartes in Paris (France). Since 2003, Dr Kotter is vice chairman of the Department of Radiology, University Hospital Freiburg and Associate Professor of Radiology at Freiburg University since 2008. Dr. Kotter served as chair of the information technology working group of the ‘Deutsche Röntgengesellschaft’ (German Society of Radiology) from 2006 to 2015. He is also vice-president of the European Society of Medical Imaging Informatics (EuSoMII).
20.09.2019