Photo courtesy of the Society for Imaging Information in Medicine
Article • PACS administrators as quality control monitors of algorithms
The “watchdogs” of AI radiology tools
Radiology AI products are a whole new world. So is running them safely and efficiently in production.
Report: Cynthia E. Keen
Artificial intelligence (AI) products are starting to demonstrate that they can improve efficiency and quality in medical imaging. Radiology software vendors are rapidly developing AI tools, and government regulatory agencies are starting to clear them for clinical use.
However, radiology AI quality control “watchdog” tools for monitoring and assessment have not been deployed as rapidly. New skills and resources are needed to use radiology AI tools safely and cost effectively, Raym Geis, MD, told attendees of the Samuel J. Dwyer III Memorial Lecture, the most prestigious event of the 2024 Society for Imaging Informatics in Medicine (SIIM) Annual Meeting.
Avoiding the “Challenger Shuttle moment”
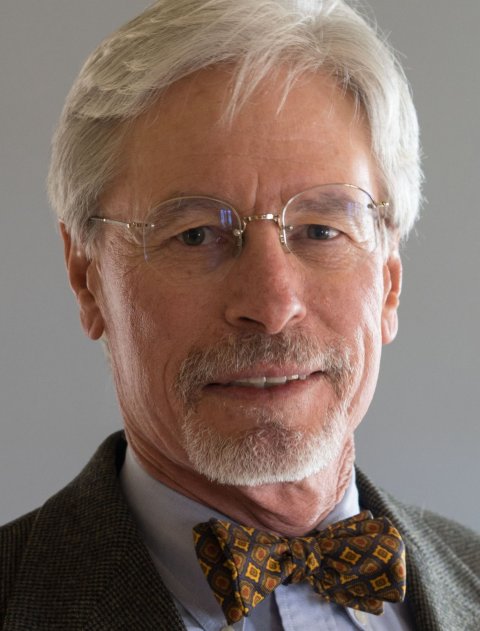
Photo courtesy of Dr. Geis
He warned that as radiology AI tools proliferate and begin to automatically communicate with each other, making independent decisions based upon their interactions, they will quickly evolve into highly complex models. These will require sophisticated, quantitative monitoring to prevent human injury, or as Geis calls this, “the Challenger Shuttle moment”. If an incident in which AI tools were to cause harm to many people, clinical medical imaging AI would come to an abrupt halt, pending lengthy investigation.
Geis’ lecture, entitled “Reliability Engineering for Intelligent Medical Imaging Systems”, suggested that radiology Picture Archiving and Communication Systems’ (PACS) administrators are best qualified to perform this role, but will need to learn challenging and sophisticated new skills. He believes SIIM should take the lead in this new initiative, as it is the professional medical society that pioneered radiology PACS development and implementation.
Geis, a radiologist and a radiology informatics specialist, Adjunct Associate Professor of Radiology at National Jewish Health in Denver, Colorado, and Clinical Assistant Professor of Radiology at the University of Colorado School of Medicine, foresees a remarkable new future for SIIM: Leading the experts to make medical imaging AI into reliable, safe, efficient, and cost-effective clinical systems.
‘PACS administrators already do many things that AI will need: installation, monitoring, management, repair and rollback. Radiology lags behind other industries in systems reliability engineering (SRE), but this works for us. We can adopt a lot of what other people in different industries have developed and are using,’ he said. ‘SIIM should take the lead to educate current members on the practice and methods of SRE as it applies to our specialized field, to promote and to define the research we need to understand, and to define best practices to operate these AI systems in medical imaging,’ emphasized Geis. ‘SIIM members’ strength is not to build these AI models, but to know how to manage these systems reliably so that there will be no medical catastrophe.’
Recommended article
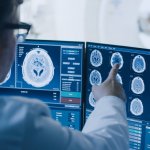
Article • Focus on radiology
PACS: the central node of imaging
Radiology without a picture archiving and communication system has become unthinkable: It records and sorts image data, controls access to the files – and gains traction also in other specialties.
The inevitable downward drift of AI tools
The challenges are many. ‘The initial one is that there is no way to predict which best AI tool will work over the long haul,’ he noted. ‘AI-modelled behaviour is determined by data. If the training data changes, the model changes. Accuracy will change. A PACS administrator currently doesn’t know which data features are the most critical,’ he explained.
Even if initially perfect, your AI tool’s results will get worse over time. [...] AI tools fail silently, slowly, and subtly
Raym Geis
‘What we do know is that any AI tool works better on the data it was trained on than the data it utilizes in a real-world clinical application. Unless the model is trained solely on your data, and even if you never change a tiny thing about how to generate the data, your results will be worse,’ said Geis. ‘The AI tool may work well on some of your exams, but not all. But you won’t know until you try it and then evaluate it.
‘Even if initially perfect, your AI tool’s results will get worse over time. Usually this is because the input data changes. Your department changes techniques and protocols. The patient population changes. The diseases change. A new scanner has been purchased that produces slightly different data. Who knows what else? You need to be observant through monitoring. All of these changes are ultimately going to happen and your results are going to drift downward. AI tools fail silently, slowly, and subtly.’
Higher stakes
Geis noted that if an AI model used by Google fails, advertisers may lose money. But nobody dies. But in radiology, rigorous monitoring is essential. In his lecture, Geis described the steps to evaluate, adopt, monitor, retrain, and decommission an AI model. He emphasized the importance of understanding and being able to access the data a vendor used to train the model, and the importance of logging versions of both the vendor software model and data set(s) tested. He explained the concept of “banded assessment monitoring”, where specific groups of features are assessed for QC and performance accuracy.
Some questions for evaluation include: What is the accuracy level stated by the vendor and how does it compare? Is AI accuracy consistent among imaging locations, different models/vendors of an imaging modality? What is the impact of different radiotherapists performing the exam, or radiologists interpreting it? How does an AI tool interpret atypical exams?
Setting the standards
Geis exhorted SIIM as an organisation to develop model card templates for medical imaging. In other industries, model cards contain model number, use case, architecture, information about training data and how it is evaluated, ethical considerations when the AI tool should not be used, and contact information to enable direct contact with the creators. He urged SIIM to set the standard for vendor AI software tool model cards, and to be the preeminent decider of information needed for monitoring to make AI tools safe and reliable.
To avoid a “Challenger Shuttle” disaster.
‘Monitoring is expensive. It is essential. PACS administrators need to grasp the emerging opportunity to become well paid Reliable Systems Engineers. Compared to other professional organizations, SIIM is positioned develop a Certified Intelligent Imaging Informatics Professional credential.’ He concluded, ‘This is a really exciting time. SIIM needs to grasp the opportunity and rejuvenate, to again become the leader and pioneering medical society of AI in radiology.’
Profile:
Raym Geis, MD, a radiologist and imaging informaticist, is Adjunct Associate Professor at National Jewish Health in Denver, Colorado, USA, and a Clinical Assistant Professor at the University of Colorado School of Medicine in Aurora. His interests include systems engineering for medical imaging AI, as well as radiology data, standards, and ethics of new data science approaches for medical imaging. Geis is a past chair of the Society for Imaging Information in Medicine and former Vice Chair of the American College of Radiology Informatics Committee.
07.11.2024