Image source: Unsplash/Pierre Bamin (Edited through Triangulator)
Article • 'Chaimeleon' project
Removing data bias in cancer images through AI
A new EU-wide repository for health-related imaging data could boost development and marketing of AI tools for better cancer management. The open-source database will collect and harmonise images acquired from 40,000 patients, spanning different countries, modalities and equipment. This approach could eliminate one of the major bottlenecks in the clinical adoption of AI today: Data bias.
Report: Mélisande Rouger
The project, named 'Chaimeleon', has been injected with € 9 million from the European Commission to create a homogeneous dataset to predict tumour behaviour with the help of AI by 2024. 18 partner institutions and external providers will select and analyse images that have been acquired at different European sites in patients who have and will be diagnosed with breast, colorectal, lung and prostate cancer between 2015 and 2024.
The researchers will design a multimodal analytical data engine to facilitate the interpretation and extraction of the relevant information stored in the database. Leading developers will validate applicability and performance for AI experimentation, and the selected tools will undergo early validation in observational clinical studies.
Harmonising images of 40,000 patients
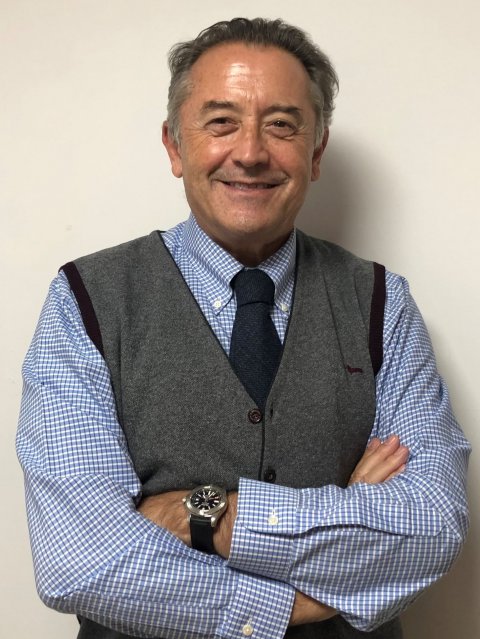
A key step of the project is to harmonise images. This enables the algorithms to obtain relevant data from them that will help clinical practice. The developers are confident that this will help overcome data heterogeneity, one of the main obstacles to the wider use of imaging biomarkers. After harmonisation, image features can be correlated to other biological findings to track changes produced by a lesion or a drug treatment, according to Chaimeleon coordinator, Dr. Luis Marti-Bonmatí, director of the medical imaging department at La Fe Hospital in Valencia, Spain. “We want to be able to predict tumour behaviour using images regardless of the equipment, protocol or version that was used for their acquisition,” he said. “Reducing variation between imaging studies is critical for AI to have a real impact.”
Images obtained from different hospitals or even different departments within the same hospital will always produce heterogeneous results. Variations in imaging equipment and constantly evolving technologies make it near-impossible to reliably generate comparable images in clinical practice, even when following the same imaging protocols. However, working with heterogeneous images may compromise data impartiality in the quantification phase. “When images come from different centres and scanners, you run the risk of working with biased data,” Marti-Bonmatí said. Because of its wide scope, the data repository should be applicable to other forms of cancer across the EU.
Selecting relevant use cases
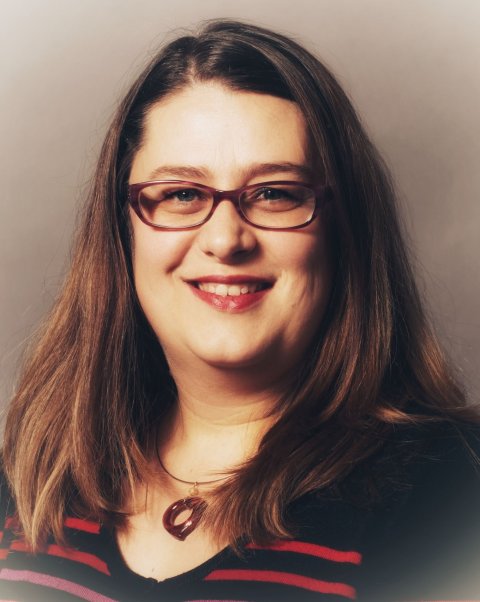
Chaimeleon will tackle regulatory, technical and ethical issues, and then include pertinent clinical use cases. Dr. Laure Fournier, professor of radiology at the Georges Pompidou European Hospital and Paris University in France, is in charge of developing the scientific content, under the aegis of Dr. Jean-Paul Beregi and the French radiology teachers association (CERF). “From image acquisition to imaging biomarkers reporting, the project is focused on inciting AI research,” she said. “Everything has to be built from scratch, that's one of the most interesting aspects of the project."
Once the database is structured, researchers will have to feed it and decide what kind of images and data they want to put in. Their aim is to find a model that is viable across the EU. Criteria for inclusion in the dataset will be defined according to the clinical question - for example, to predict response to immunotherapy in lung cancer. This determines which kind of images should be sorted out and which type of biological and clinical data can help provide relevant information.
Even though harmonisation is a huge challenge, diversity of data is paramount to avoid being too specific. “It’s very important that the images come from different sites, equipment and acquisition methods, with different slice thicknesses, etc.,” Fournier said. To achieve this, a proper balance between quantity and feasibility must be found - which inevitably leads to trade-offs: “The more relevant data you collect, the fewer patients you have.” The selected imaging data must also add value to existing clinical practice, for example PSA in prostate cancer, she added.
Pioneering new combinations to explain AI
Our goal is to demonstrate that this combination is possible to reduce the bias in quantification, by building models that are more explainable
Angel Alberich Bayarri
The Chaimeleon repository will also be an opportunity to test combinations of advanced computational techniques, in order to make AI more understandable. Researchers will evaluate the combination of self-supervised learning and Generative Adversarial Networks (GANs) to enhance reproducibility of radiomics features and parameters extracted from cross-vendor and cross-institution CT-MR-PET/MR imaging data. The effort will be led by the Imperial College of Science Technology Medicine in London, UK, GE Healthcare and Quibim, a medtech company that extracts imaging biomarkers from radiology images. “Our goal is to demonstrate that this combination is possible to reduce the bias in quantification, by building models that are more explainable,” Angel Alberich Bayarri, CEO and co-founder at Quibim, said. “Think of an iPad. You never give the instruction manual to a kid, they learn by interacting with the tool. In self-supervised learning, you interact with the data to find patterns within.”
The researchers thus want to explore the use of self-supervised learning as a paradigm shift in AI, where models are autonomously developed without the need of annotated data. In self-supervised schemes, part of the data contained in the images is withheld during the training process. This way, the algorithm learns to predict hidden parts from the knowledge acquired from complete images. The project will also build on tools that have been validated for image harmonisation of multi-vendor or multicentric data and interpretability of AI. Quibim will notably use its experience in building the cloud-based platform of another EU project called PRIMAGE, which gathers thousands of pediatric oncology cases by integrating hospitals' repositories from all across Europe.
Recommended article
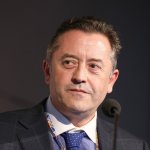
Article • PRIMAGE project
Aiming AI at lethal paediatric tumours
La Fe University and Polytechnic Hospital in Valencia, Spain, is coordinating EU-funded program PRIMAGE, which uses precision information from medical imaging to advance knowledge of the most lethal paediatric tumours, by establishing their prognosis and expected treatment response using radiomics, imaging biomarkers and artificial intelligence (AI).
Profiles:
Dr. Luis Marti Bonmatí is chairman of radiology and director of the medical imaging department at La Fe University and Polytechnic Hospital, Valencia. He is full member of the Spanish Royal National Academy of Medicine representing radiology, and was founder and director of the research group on biomedical imaging (GIBI230) within La Fe Health Research Institute. He has been president of the European Society of Magnetic Resonance in Medicine and Biology (ESMRMB), Spanish Society of Radiology (SERAM), Spanish Society of Abdominal Radiology (SEDIA) and European Society of Gastrointestinal and Abdominal Radiology (ESGAR).
Dr. Laure Fournier is professor of radiology at the Georges Pompidou European Hospital and Paris University (Université de Paris), France. Her time is divided between clinical work on urogenital cancers and imaging research in the INSERM U970 imaging research lab. She works on functional imaging, radiomics and big data, to extract quantitative parameters from images reflecting tumor physiology and biology, more specifically to define response to therapy. She organizes the curriculum on AI for French radiology residents and is a member of the scientific committee of the DRIM France AI national image database and the medical imaging chair in the PRAIRIE interdisciplinary AI institute.
Dr. Angel Alberich Bayarri is CEO and co-founder of Quibim, a medtech company based in Valencia, Spain. A trained PhD in biomedical engineering, he served as scientific-technical director of the biomedical imaging research group (GIBI230) and as a board member of the European Society of Medical Imaging Informatics (EUSOMII) and the European Imaging Biomarkers Alliance (EIBALL). He has more than ten years of experience in the development of quantitative image analysis solutions and their integration in clinical practice. He has co-authored more than 60 research papers and has participated in numerous international research projects in medical imaging.
04.02.2021