Image source: Adobe Stock/SergeyBitos
Article • Radiology & artificial intelligence
How to integrate AI in the clinical workflow: 7 lessons
In radiology, it is not about if but about when artificial intelligence (AI) will be used, said Professor Dr Tim Leiner of Utrecht University Medical Center at this year’s European Congress of Radiology in Vienna. For all those who are new to AI, the Dutch radiologist gave an overview of the lessons he and his team have learnt so far.
Report: Michael Krassnitzer
And indeed Professor Leiner offered seven distinct and entirely practice-oriented lessons.
Lesson 1: Identify the use cases
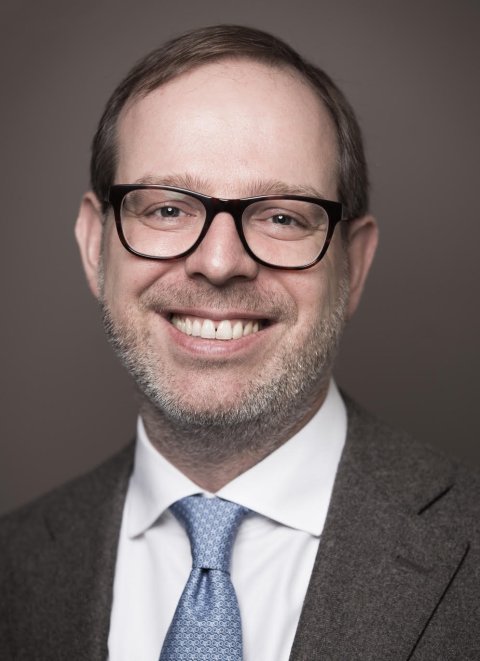
‘Identify the use cases. Artificial intelligence is not an end in itself; it is a means – the solution to specific problems. Focus on a problem which you want to solve. Ask yourself where the use of AI really makes a difference.’
Lesson 2: Set up a team of experts
‘Set up a team of people with diverse expertise – among them obviously clinicians who contribute basic knowledge of the medical discipline in question. However, you also need healthcare IT and informatics specialists, e.g. PACS administrators. Moreover, I would recommend bringing AI experts on board since they can realistically assess the providers’ claims and promises. In addition, a patient representative is a good idea. Many algorithms are mere “black boxes” and the exchange with a patient representative can offer insights into patient psychology: some might feel uneasy about the technology’s lack of transparency and reject it outright.’
Lesson 3: Choose a platform
‘Choose a platform that fits into your environment. Take a long-term perspective: the range of available applications will grow. In Utrecht, we tried to be as open as possible. We wanted a platform where we decide what’s running on it, not the platform provider. It was crucial for us that the platform was able to process algorithms by different providers as well as the algorithms we will develop in-house.’
Recommended article
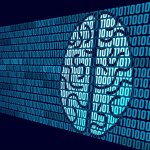
Article • Technology overview
Artificial intelligence (AI) in healthcare
With the help of artificial intelligence, computers are to simulate human thought processes. Machine learning is intended to support almost all medical specialties. But what is going on inside an AI algorithm, what are its decisions based on? Can you even entrust a medical diagnosis to a machine? Clarifying these questions remains a central aspect of AI research and development.
Lesson 4: Choose a test product
‘After you have decided on a platform, choose a product that promises to be a good solution for your specific clinical problems.’
Lesson 5: Define criteria of success
‘We recommend establishing specific criteria beforehand that define when the implementation of an application is considered successful. Think about the goals you want to achieve with the application, e.g. higher throughput, higher precisions, saving time, or decreasing costs.’
Lesson 6: Conduct a pilot project in the hospital
Never, ever buy an algorithm you did not test in your own clinical workflow. Don’t rely on demos
Tim Leiner
‘Conduct a pilot project in your own institution. Never, ever buy an algorithm you did not test in your own clinical workflow. Don’t rely on demos. Collect as much feedback as possible from radiologists and clinicians. The test should run over a longer period of time, at least a few weeks, preferably some months. Assess the success of the pilot project using the criteria you defined before the test. If you don’t do that you are setting yourself up for disappointment. Buy the algorithm only after a thorough assessment.’
Lesson 7: Implement smoothly
‘When you are satisfied with the platform and the product, implement them smoothly. It is crucial to involve the users! Set up a team of “ambassadors” who gathered experience during the pilot project and can thus answer questions during and after roll-out.’
In addition to offering these lessons, Professor Leiner briefly addressed the obstacles that have to be surmounted when introducing AI in radiology. He pointed out that many algorithms were developed on the basis of very few cases: ‘Due to the General Data Protection Regulation and other privacy considerations the data sets are frequently small and biased. This is something we need to always keep in mind.’ Professor Leiner’s take-home message: ‘The introduction of artificial intelligence in healthcare is difficult and hard work.’
27.11.2022