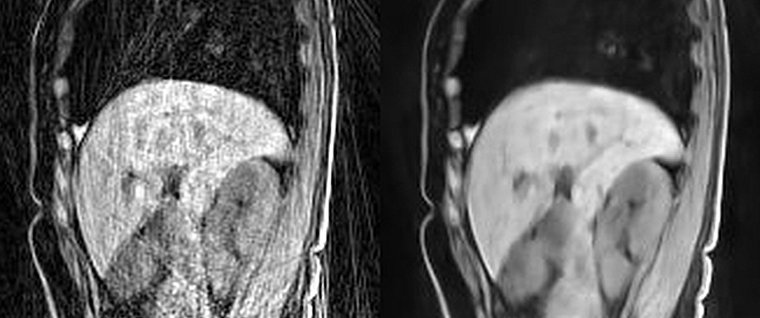
Image source: Washington University in St. Louis
News • Imaging assistance
Deep learning method boosts MRI results without new data
A team of researchers from Washington University in St. Louis has found a new deep learning method that can minimize artifacts and other noise in MRI images that come from movement and a short image-acquisition time.
When patients undergo an MRI, they are told to lie still because even the slightest movement compromises the quality of the images and can create blurred spots and speckles known as artifacts. Moreover, a long acquisition time is usually required to provide high-quality MRI images.
Hongyu An, a professor of radiology at the School of Medicine’s Mallinckrodt Institute of Radiology (MIR), and Ulugbek Kamilov, assistant professor of computer science and engineering and of electrical and systems engineering in the McKelvey School of Engineering, led an interdisciplinary team that developed the Phase2Phase deep learning method, which they trained using images with artifacts and without a ground truth, in this case, a perfect image without artifacts. Results of the work were published in Investigative Radiology.
In an MRI, it may be easy or hard to scan someone, depending on their physical health, but everyone still has to breathe
Ulugbek Kamilov
Deep learning learns directly from the training data how to determine the signal from artifacts and noise, or variations in signal intensity in an image. Many existing deep learning-based MRI reconstruction methods are able to remove artifacts and noise but they learn from a ground truth reference, which can be difficult to obtain. “In an MRI, it may be easy or hard to scan someone, depending on their physical health, but everyone still has to breathe,” Kamilov said. “When they breathe, their internal organs move, and we have to determine how to correct for those movements.”
In Phase2Phase, the team feeds the deep learning model with only sets of bad images and trains the neural network to predict a good image from a bad one without a ground truth reference. Weijie Gan, a doctoral student in Kamilov’s lab and a co-first author on the paper, wrote the software for Phase2Phase to remove noise and artifacts. Cihat Eldeniz, an instructor of radiology at the Mallinckrodt Institute of Radiology and co-first author, worked on the MRI acquisition and motion detection used in the study. They modeled Phase2Phase after an existing machine learning method known as Noise2Noise, which restores images without clean data.
In a retrospective study, the team evaluated MRI data from 33 participants — 15 healthy persons and 18 patients with liver cancer, all of whom were allowed to breathe normally while in the scanner. These results were compared with images reconstructed with another deep learning method, UNet3DPhase, which is trained on a high-quality ground truth; compressed sensing; and multicoil nonuniform fast Fourier transform (MCNUFFT). In addition, this Phase2Phase method has successfully reconstructed 66 MRI data sets acquired at another institution using different acquisition parameters, demonstrating its broad applicability.
Recommended article
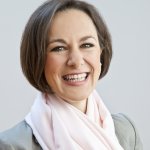
Article • Innovation
AI helpers simplify clinical MRI scans
The new 1.5 Tesla MRI from Siemens Healthineers, Magnetom Sola, is packed with helpful algorithms and other functions. AI-supported systems monitor patients and scan parameters and ensure consistent image quality. Whilst visitors at this year’s ECR-Expo admired the new device, Prof. Ulrike Attenberger has already tested it in practice.
Two radiologists, who were blinded to which reconstruction method was used on the images, reviewed the images for their sharpness, contrast and artifacts. They found that the Phase2Phase and UNet3DPhase images had higher contrast and fewer artifacts than the compressed sensing images. The UNet3DPhase and Phase2Phase images were reported to be sharper than the compressed sensing images by one reviewer, but not by the other. The Phase2Phase and UNet3DPhase images were similar in sharpness and contrast, while the UNet3DPhase images had fewer artifacts than the Phase2Phase. The Phase2Phase images preserve the motion vector fields, while the compressed sensing images artificially reduced the motion vector fields. “The Phase2Phase deep learning method provides an excellent solution for a rapid reconstruction of high-quality 4D liver images using only a fraction of acquisition time,” said An, who also is a professor of neurology. “It improves image quality for better clinical diagnosis.”
The research team and Siemens Healthineers collaborated to develop a work-in-progress (WIP) software. This WIP software was disclosed to the Washington University in St. Louis Office of Technology Management, and a patent application was jointly filed.
Source: Washington University in St. Louis
08.09.2021