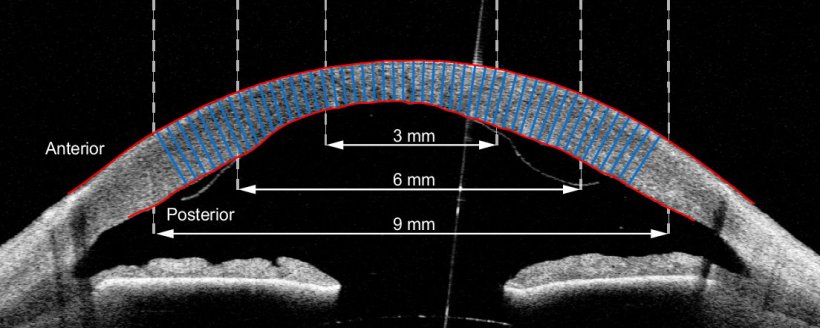
Image source: Heslinga et al., Scientific Reports 2021 (CC BY 4.0)
Article • Keratoplasty
Corneal transplantation surgery: AI for an eye
Many consider our eyes to be the windows to the soul. Yet the cornea, the panes of these windows, sometimes need significant repair. Friso Heslinga, PhD, created artificial intelligence models to support surgeons in one of the oldest and most common transplantation procedures.
Report: Sascha Keutel
Our eye has several layers. The cornea is the transparent, dome-shaped outer layer that helps focus light, so we can see clearly. The light passes through the cornea and is then focused by the lens onto the retina, where it interacts with photoreceptor cells to generate signals for the brain to process. A damaged or diseased cornea can become less transparent, which can prevent light reaching the retina and cause the transmitted image to be distorted or unclear. When this happens, the patient might need corneal transplant surgery, in which the surgeon removes all or part of the damaged cornea and replaces it with a healthy layer of tissue. The type of keratoplasty, or corneal graft transplantation, depends on which part is damaged or how much of the cornea needs replacing. “Over the years, corneal transplantation surgery has improved a lot. Surgeons started by replacing the whole layer. Now, it only comprises the endothelial cells. For the cornea it’s only a monolayer, a single layer of cells,” Friso Heslinga, from the Medical Image Analysis group at the Eindhoven University of Technology, said. He added: “It's an immaculate procedure. They scrape it away from the donor, roll it up, make a small hole in the patient’s cornea. Then they put the roll through that hole and roll it out again and use a small bubble, e.g., air, to push it on the cornea.”
Deep learning research
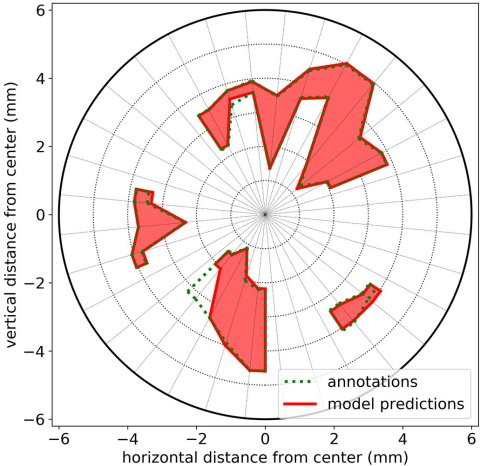
Image source: Heslinga et al., Translational Vision Science & Technology 2020 (CC BY-NC-ND 4.0)
Heslinga and his collaborators did four projects on the corneal transplantation surgery. First, they developed an AI model to determine intraoperatively whether or not the donor graft is orientated correctly. “An expert can look at the images and figure it out, but it takes time. And it’s not that easy,” the expert said. “We used intraoperative optical coherence tomography (OCT) imaging to take images intra-operatively. So, the quality is very often suboptimal. It’s very hard to see the graft, very often you see only part of it,” he said.
With collaborators from the ophthalmology department at University Medical Center (UMC) Utrecht, Heslinga trained an AI segmentation model to automatically assess the orientation of the new layer of tissue. This is important because if it is upside-down, then it cannot be attached to the existing cornea. Furthermore, they did two post-operative projects: first, to assess the level of attachment of the graft after transplantation surgery. “We collaborated with corneal specialists from the Rigshospitalet–Glostrup in Copenhagen to design a method that finds any spots where the donor tissue is detaching from the underlying tissue.”
For the second post-operation project, Heslinga and his collaborators developed AI models, which were trained using supervised deep learning, to automatically measure corneal thickness at every point in the eye and construct a detailed thickness map. An abnormal corneal thickness is linked to various corneal disorders. “Corneal thickness is something you could measure by hand; you could draw the lines on the monitor. You still would need a software to do a thickness profile. Again, that takes time doctors rarely have in clinical practice. That is where the AI models come in: to save time,” he said.
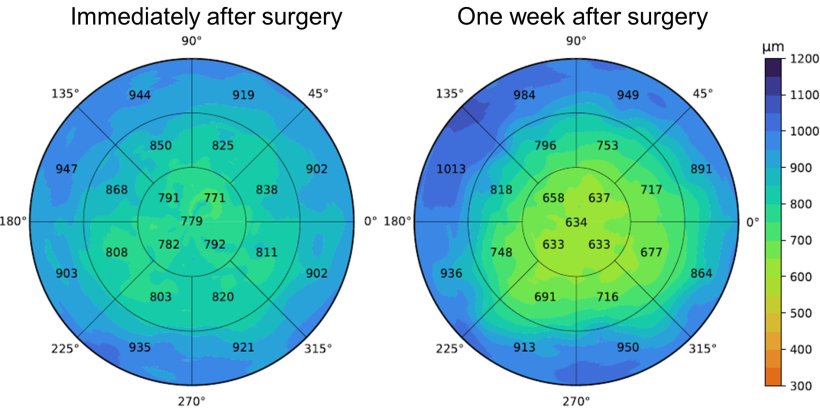
Image source: Heslinga et al., Scientific Reports 2021 (CC BY 4.0)
For the fourth project, Heslinga developed a model for assessing specular microscopy images. Looking at the individual cells, the technique gives the physician an idea about the state of the cornea. When they zoom in, they can see the number of cells in a square millimetre or differences in cell size. Similar to the corneal thickness maps, these results can then be provided to an ophthalmologist to establish the success of the transplantation procedure and help determine if further treatment is needed. “For an assessment of these types of images, much depends on the experience of the surgeon. There is quite some information being lost, and that’s the part where we think our models will help: by bringing this extra information, we can do measurements and relate these measurements to outcome,” Heslinga is convinced.
From research to clinical practice
For good implementation, we need collaborations with the manufacturer of the OCT scanners and validate for all patients using that scanner
Friso Heslinga
Currently, the AI models are only used for research. They still need extensive clinical testing and validation before physicians can use them in clinical settings. Heslinga is optimistic that soon, artificial intelligence will support ophthalmologists: “We’ve shown that the systems work in a specific case using data from the hospital in Denmark. However, showing that the models work for one data set isn’t enough. We want to show that it works for different data sets, or patient groups,” Heslinga said. “For good implementation, we need collaborations with the manufacturer of the OCT scanners and validate for all patients using that scanner. That way, we could work on all the images that type of scanner gets from patient groups with different demographics. That would make sure that everything we provide is useful for the patients.”
Profile:
Friso Gerben Heslinga, PhD, works with the Medical Image Analysis group at the Eindhoven University of Technology, the Netherlands. His PhD research was on deep learning for corneal and retinal image analysis. Friso obtained a BSc. and a MSc. in biomedical engineering at the University of Twente. Heslinga’s research interests include biomedical physics, medical image analysis, deep learning, and health economics.
17.10.2022