Article • The role of the radiographer
AI in radiation protection: a potential game changer
Radiographers could help design new artificial intelligence (AI) tools for radiation protection, Mark McEntee, professor of diagnostic radiography at University College Cork, Ireland, argued during the annual meeting of the European Society of Medical Imaging Informatics (EuSoMII) in October.
Report: Mélisande Rouger
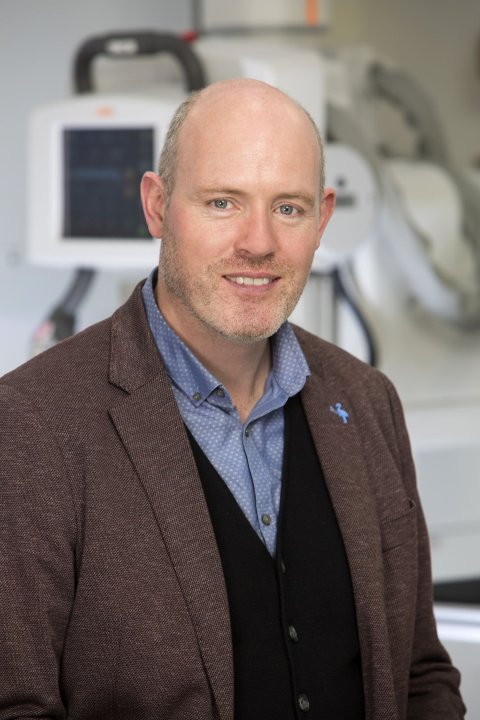
Image source: University College Cork
‘There are a lot of papers on AI, but not in the area of radiation protection and AI,’ he told delegates in Valencia, Spain. ‘AI in radiation protection is in its infancy, but it could be a game changer.’ The expert identified three pillars of AI in radiation protection: justification of x-ray examinations; optimization of the examinations; and dose limitation. McEntee encouraged AI companies to aim for the low hanging fruits in those three areas. For example, justification is virtually untouched, he said: ‘You could prevent unjustified examinations by impeding those that are unnecessary. You might be surprised, but it happens a lot. Sometimes mistakes are made or, because results aren’t available yet, doctors refer the patients again. The cumulative effect of dose to patient is something physicians should be worried about and it’s a target for AI.’
AI could also help clinicians identify the correct examination to carry out. Some clinical decision-making tools already exist, but there is room for more, McEntee believes. Guidelines such as the American appropriateness criteria, the ESR iGuide or recommendations issued by the RANZC can help AI developers, but they are static resources that are not based on evidence, he explained. ‘Information on justification is often based on the consensus of experts rather than well-designed controlled trials.’
Consider, when you design your products, that you have radiographers in the loop [...]. Give us the tools to label [the images] and we will
Mark McEntee
Another challenge is patient consent. ‘Many times, patients don’t understand that there’s radiation involved,’ the expert pointed out. In some cases, medical experts face difficulties in explaining the procedures. ‘Consent of patients is lacking, so decision support tools and potential radiology products could help here as well,’ he said. Verifying the quality of data relies on humans, so it is expensive and challenging. AI companies should think of radiographers as a translation between the request of the doctor and the actual needs. ‘Consider, when you design your products, that you have radiographers in the loop, and if you make them part of your workflow, they can do things like labelling examinations and artifacts,’ he said. ‘Give us the tools to label these things and we will.’
Design along the clinical question to further reduce dose
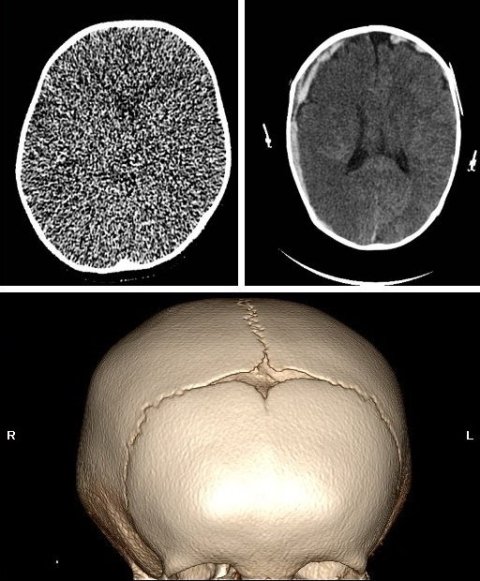
Once the examination has been justified it must be optimised. That’s also an area where radiographers can help, McEntee continued. ‘Optimisation is a shared responsibility, but at the patient’s side it’s done by radiographers.’ Vulnerable patients who return on multiple occasions to the hospital – for example patients with chronic disease – need lifelong imaging. This is ‘an opportunity to learn from the previous imaging and look at things in ways we can enhance current imaging sets based on previous imaging sets. We can reduce the dose and learn from patients,’ said the expert, who presented the case of a paediatric patient with cranial steatosis to illustrate this point. ‘The problem was in the shape of the head’: Because the fontanelle didn’t open as it should have, the only are relevant for imaging was these bony windows. ‘But in this case, the entire brain was scanned – this wasn’t essential. They used 80% more dose than what was necessary,’ he said. The clinical question should drive the protocol, he insisted. ‘How decisions are made regarding the appropriate protocols for justification is a really important step.’
Another way to help reduce dose would be for radiographers to borrow radiology equipment such as AI-automated 3D cameras to better position the patient in radiation therapy. ‘We already have good tools on automatic exposure control and we’re increasingly using AI. (…) That would be a major way in which we can reduce dose.’
Adjusting dose to a patient is complex, he went on, taking the example of breast screening. ‘There is no average breast. The average is based on an estimate by the physician that 50% of the glandular tissue of the breast is normal and 50 isn’t,’ he explained. ’We want to use AI to measure the density of this breast. It does far better with far less radiation. If you used that classification of breast density to replace the mean average, then you would actually calculate the appropriate dose required for a woman.’ Radiologists and radiographers can feed in an algorithm as they go along the workflow. ‘If we build the infrastructure of AI so that it’s collecting data of users as we go along, we’d have potential benefits for patients and healthcare systems, including shorter waiting times, fewer unnecessary examinations, quicker diagnosis, and reduced instances about missed diseases because radiologists aren’t overwhelmed looking at thousands of images,’ he concluded.
27.11.2022