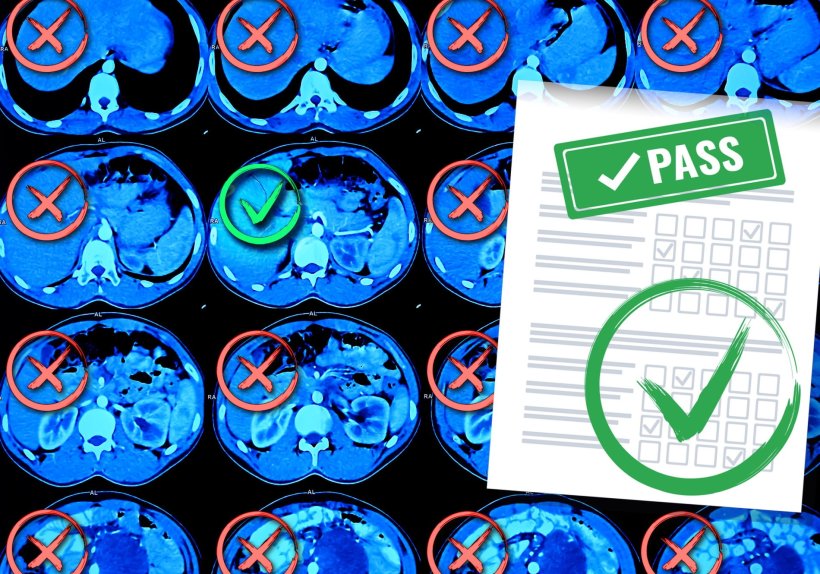
Image credit: NLM
News • Integration into medical decision-making
AI adept at answering medical questions – but fails to explain how
Researchers at the National Institutes of Health (NIH) found that an artificial intelligence (AI) model solved medical quiz questions—designed to test health professionals’ ability to diagnose patients based on clinical images and a brief text summary—with high accuracy.
However, physician-graders found the AI model made mistakes when describing images and explaining how its decision-making led to the correct answer. The findings, which shed light on AI’s potential in the clinical setting, were published in npj Digital Medicine. The study was led by researchers from NIH’s National Library of Medicine (NLM) and Weill Cornell Medicine, New York City.
“Integration of AI into health care holds great promise as a tool to help medical professionals diagnose patients faster, allowing them to start treatment sooner,” said NLM Acting Director, Stephen Sherry, PhD “However, as this study shows, AI is not advanced enough yet to replace human experience, which is crucial for accurate diagnosis.”
This technology has the potential to help clinicians augment their capabilities with data-driven insights that may lead to improved clinical decision-making. Understanding the risks and limitations of this technology is essential to harnessing its potential in medicine
Zhiyong Lu
The AI model and human physicians answered questions from the New England Journal of Medicine (NEJM)’s Image Challenge. The challenge is an online quiz that provides real clinical images and a short text description that includes details about the patient’s symptoms and presentation, then asks users to choose the correct diagnosis from multiple-choice answers. The researchers tasked the AI model to answer 207 image challenge questions and provide a written rationale to justify each answer. The prompt specified that the rationale should include a description of the image, a summary of relevant medical knowledge, and provide step-by-step reasoning for how the model chose the answer.
Nine physicians from various institutions were recruited, each with a different medical specialty, and answered their assigned questions first in a “closed-book” setting, (without referring to any external materials such as online resources) and then in an “open-book” setting (using external resources). The researchers then provided the physicians with the correct answer, along with the AI model’s answer and corresponding rationale. Finally, the physicians were asked to score the AI model’s ability to describe the image, summarize relevant medical knowledge, and provide its step-by-step reasoning.
The researchers found that the AI model and physicians scored highly in selecting the correct diagnosis. Interestingly, the AI model selected the correct diagnosis more often than physicians in closed-book settings, while physicians with open-book tools performed better than the AI model, especially when answering the questions ranked most difficult.
Importantly, based on physician evaluations, the AI model often made mistakes when describing the medical image and explaining its reasoning behind the diagnosis—even in cases where it made the correct final choice. In one example, the AI model was provided with a photo of a patient’s arm with two lesions. A physician would easily recognize that both lesions were caused by the same condition. However, because the lesions were presented at different angles—causing the illusion of different colors and shapes—the AI model failed to recognize that both lesions could be related to the same diagnosis.
Recommended article
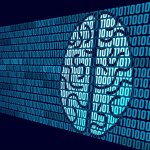
Article • Technology overview
Artificial intelligence (AI) in healthcare
With the help of artificial intelligence, computers are to simulate human thought processes. Machine learning is intended to support almost all medical specialties. But what is going on inside an AI algorithm, what are its decisions based on? Can you even entrust a medical diagnosis to a machine? Clarifying these questions remains a central aspect of AI research and development.
The researchers argue that these findings underpin the importance of evaluating multi-modal AI technology further before introducing it into the clinical setting. "This technology has the potential to help clinicians augment their capabilities with data-driven insights that may lead to improved clinical decision-making,” said NLM Senior Investigator and corresponding author of the study, Zhiyong Lu, PhD “Understanding the risks and limitations of this technology is essential to harnessing its potential in medicine.”
The study used an AI model known as GPT-4V (Generative Pre-trained Transformer 4 with Vision), which is a ‘multimodal AI model’ that can process combinations of multiple types of data, including text and images. The researchers note that while this is a small study, it sheds light on multi-modal AI’s potential to aid physicians’ medical decision-making. More research is needed to understand how such models compare to physicians’ ability to diagnose patients.
The study was co-authored by collaborators from NIH’s National Eye Institute and the NIH Clinical Center; the University of Pittsburgh; UT Southwestern Medical Center, Dallas; New York University Grossman School of Medicine, New York City; Harvard Medical School and Massachusetts General Hospital, Boston; Case Western Reserve University School of Medicine, Cleveland; University of California San Diego, La Jolla; and the University of Arkansas, Little Rock.
Source: NIH/National Library of Medicine
23.07.2024