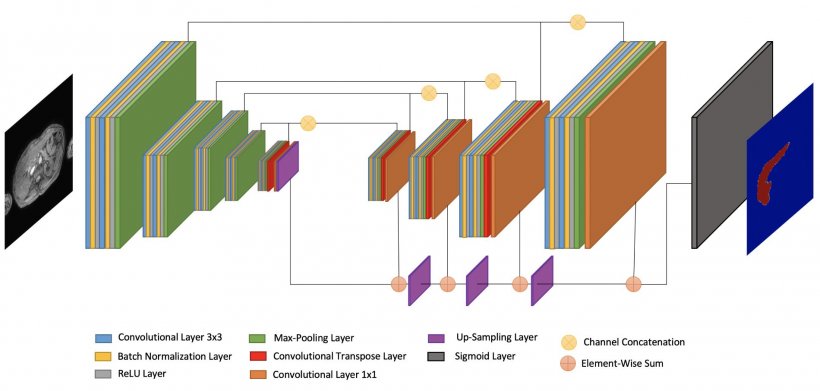
Article • Expectations vs. reality
AI in clinical practice: how far we are and how we can go further
Luis Martí-Bonmatí, Director of the Medical Imaging Department at La Fe Hospital in Valencia, highlighted the need to assess utility when developing AI tools, as he looked into the present and future of artificial intelligence’s adoption in radiology during ECR 2020.
Report: Mélisande Rouger
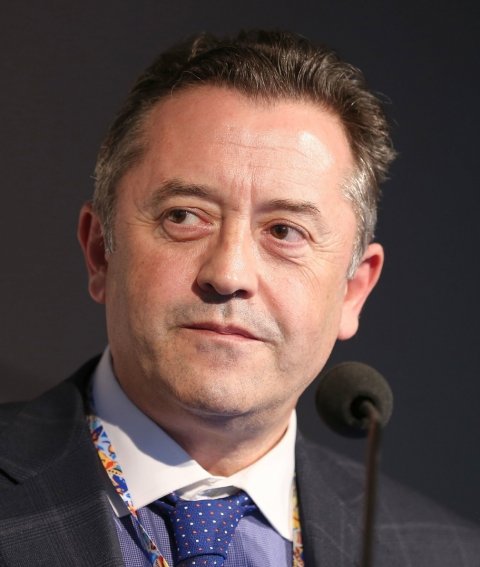
Artificial intelligence (AI) can impact and improve many aspects of clinical practice. But current expectations are too great and need to be toned down by looking at opportunities, according to Luis Martí-Bonmatí. “AI is revolutionizing our expectations. But we need to look at areas in which we’ve showed utility. This is where AI makes more sense,” he said. Radiologists must help develop but also critically assess solutions that tackle image acquisition, data collection, annotation, training testing, validating verifying and innovation.
Image reconstruction software that enable faster and better acquisitions have already been developed by the industry and are used in clinical practice. Deep Learning and CNN algorithms are also available to detect and classify disease, and to draw the radiologist’s attention to potential lesion areas through attention maps.
La Fe Research Group has developed an algorithm that enables to do prostate virtual biopsy, via automatic and simultaneous segmentation and quantification using end-to-end AI methods for IVIM, Kurtosis, Stretched and Ktrans nosologic maps. Segmentation is a huge area of interest not just for virtual biopsies but also virtual dissections, to virtually extract the whole organ, bone or lesion of interest. La Fe researchers have already commercialized a Virtual Dissection tool that enables to automatically segment the liver using Deep Supervision methods for whole liver Fat and Iron quantification (see illustrations).
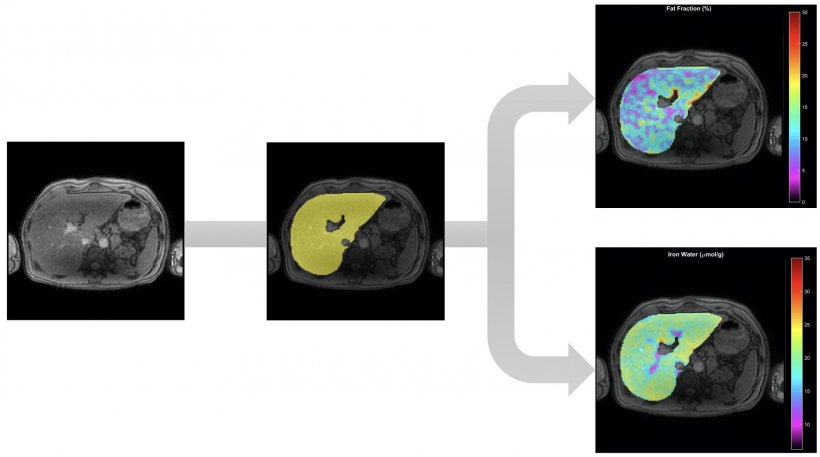
The team is now working on a classification tool to perform massive analysis of source images, to obtain imaging biomarkers that are linked to endpoints for aggressive prostate cancer detection. The software should receive CE mark in the coming months. Many solutions on the market are also available for data mining and knowledge extraction, which are of much interest to data scientists. Efforts must be focused on reproducibility, as variability in highly heterogeneous images – due to different vendors, releases and protocols – currently limits quantitative biomarkers replication studies.
In October 2020, the Chaimeleon project, a new EU-funded initiative, will look into that issue, by promoting compatibility between different sets of images. “We will train neural networks to recognize and minimize differences between images. That’s an important step to accelerate market transition of AI tools for cancer management,” said Martí-Bonmatí, who will coordinate the study.
In addition to standardized images, a lot of data is required to advance AI. All this data should be present in the quantitative structured report (SR), which must become an AI data provider, by incorporating quantitative parametric images and measurements, and offering comprehensive presentation supports classification. “The SR must be our link to treatment allocation, hospital database systems and data mining solutions. This is how we can help solve problems all the way from predisposition and risk of suffering a disease to follow-up and health monitoring,” he said.
Strong vs. weak AI will determine its future
AI must not only give a solution to a problem, but many solutions to many problems that may be entwined
Luis Martí-Bonmatí
Next steps need to be taken must be centered on interactive-continual learning, and involving radiologists in the process is a way to increase prediction accuracy. “AI needs to be implemented with the aim to improve it. AI’s output must be analyzed by the radiologist, who can make further adjustments if needed. We need to allow trial and error, and have a continuous feedback based on experience,” he said.
Another key direction will be to focus on augmented imaging challenges, by promoting a strong vs. weak AI. “A weak AI is focused on one narrow task and this is what occurs in most current AI applications. A strong AI completes any task that humans can perform and generates augmented human intelligence. It enables a more efficient and precise diagnosis, reducing human intervention in time-consuming conventional imaging tasks,” he said.
By adopting this approach, radiologists might uncover some unexpected new imaging outcomes that can facilitate or enhance the diagnosis, for example through opportunistic screening, phenotyping, predicting or prognosis. “AI must not only give a solution to a problem, but many solutions to many problems that may be entwined. It’s not about just giving a lesion’s radiomics or grade, it’s also about which lesion I should treat, how I should treat it and what will be the final prognosis,” he concluded.
Profile:
Dr Luis Martí-Bonmatí is Chairman of Radiology and Director of the Medical Imaging Department at La Fe University and Polytechnic Hospital, Valencia. He is full member of the Spanish Royal National Academy of Medicine representing Radiology, and was founder and Director of the Research Group on Biomedical Imaging (GIBI230) within La Fe Health Research Institute. He has been President of the European Society of Magnetic Resonance in Medicine and Biology (ESMRMB), Spanish Society of Radiology (SERAM), Spanish Society of Abdominal Radiology (SEDIA), and European Society of Gastrointestinal and Abdominal Radiology (ESGAR).
16.07.2020