Sponsored • A discipline transforming
Adding value with AI in medical imaging
In the next five to 10 years, artificial intelligence is likely to fundamentally transform diagnostic imaging. This will by no means replace radiologists, but rather help to meet the rising demand for imaging examinations, prevent diagnostic errors, and enable sustained productivity increases.
Imaging in the age of AI
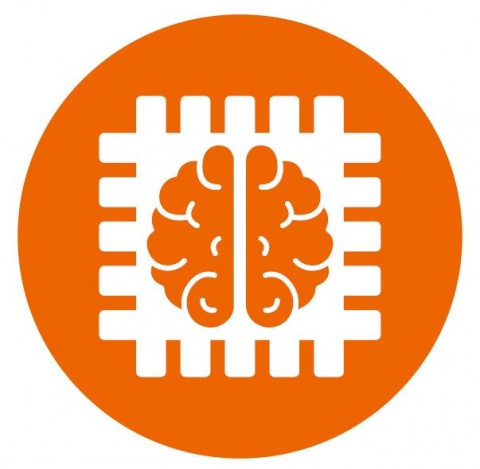
In recent years, artificial intelligence (AI) gained entry into everyday life in various ways, from language recognition tools on smartphones to the analysis of financial transactions, to algorithms for self-driving cars, or for playing the strategic board game Go.1 Medical imaging, too, is likely to undergo a fundamental transformation in the near future. “It is easy to predict that AI will be increasingly implemented in medical imaging systems,” Italian doctor Francesco Sardanelli commented in an editorial feature on dominant trends in radiology.2 Similarly, according to a recent poll, over 50 percent of global healthcare leaders expect the role of AI in monitoring and diagnosis to expand.3
Although the use of AI is already common practice in some aspects of the field of imaging, market analysis foresees a further boom over the next five to ten years.4 Newer AI methods, such as “deep learning,” could pave the way for quantitative, standardized, yet also personalized imaging, while helping to prevent diagnostic errors and, at the same time, enabling sustained productivity increases. Radiologist Keith Dreyer of Harvard Medical School emphasized at an expert meeting in the US that “Meaningful AI will improve quality, efficiency, and outcomes.”5
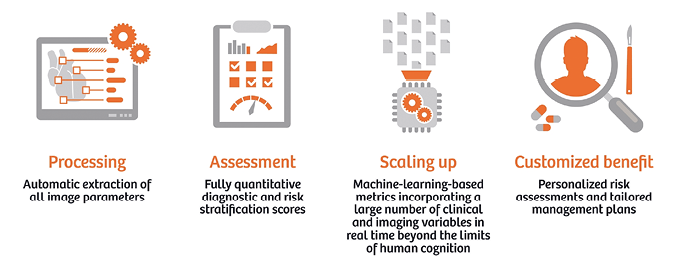
The case of cardiac imaging
Challenges for a transforming discipline
There are several factors simultaneously driving integration of AI in radiology. First, in many countries around the world there is a shortage of doctors trained in radiology, considering the rising demand for diagnostic imaging. This leads to greater demands for work efficiency and productivity. For example, the radiology consultant workforce in England went up five percent between 2012 and 2015, while in the same period the number of CT and MR scans increased by 29 and 26 percentage points respectively.6 Today, the average radiologist interprets an image every three to four seconds, eight hours a day.7
Second, the image resolution of today’s scanners is continuously improving – resulting in an ever greater volume of data. Indeed, the estimated overall medical data volume doubles every three years. There is high future potential in the transformation of radiology from qualitative interpretations to a quantitative discipline, deriving clinically relevant information from extensive data sets (“radiomics”). “Images are more than pictures, they are data,” state American radiologist Robert Gillies and his colleagues.8 However, this transformation will require automated procedures, at least some of which will come under the field of AI.
Not least, diagnostic errors are an unresolved problem. Studies show that erroneous interpretations occur in about four percent of all radiology diagnoses, with the error rate varying individually and depending heavily on the procedure.9 Moreover, overlooked pathological findings lead not only to worse patient outcomes, but also to missed billing opportunities. Indeed, AI could help to overcome these challenges, proving an indispensable aid for efficient, data-based, and less-error-prone diagnostics. The astonishing advances in the field justify this optimism.
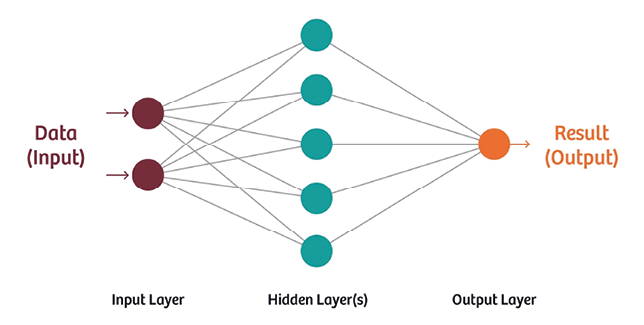
Making AI a clinical routine
The use of AI in medical imaging is not new – algorithms today are, however, much more powerful than traditional applications.10 Unlike previous AI methods, first introduced in the US in the late 1990s – particularly mammography screening with its many shortcomings – today’s technologies will likely prove to be transformative. In particular, “deep learning” – an innovative machine learning approach – is a powerful tool for analyzing imaging data.11 Deep learning rests upon computer programs known as artificial neural networks (ANNs) that are inspired by the neurobiological architecture in the brain. In image recognition tasks, the error rate of such ANNs is now down to just a few percent.12
For example, almost all cases of lung tuberculosis can be detected on chest radiographs (with 97 percent sensitivity and 100 percent specificity), when using two deep neural networks for image analysis and having a radiologist evaluate only equivocal cases, according to a pilot study.13 Such a workflow could have great practical relevance, particularly in regions with few radiologists on hand. Other clinical AI applications that are within reach range from improved detection of pulmonary nodules in CT 14 to quantitative analyses of brain structures and neurological diseases with imaging biomarkers and “lab-like” results,15 to personalized mortality risk scores in cardiovascular patients.16
Already today, AI is speeding up radiological workflows. For instance, Siemens Healthineers has developed an AI-based tool for its 3D diagnostic software “syngo.via,” which automatically detects anatomical structures, independently numbers vertebrae and ribs, and also aids in precisely overlaying different examination dates or even different modalities. The company holds 400 patents and patent applications, and supports various research collaborations with top-level hospitals to promote the field. Numerous other applications are thus in development or can be expected from a range of companies in coming years.17
The way forward
These upcoming developments will not replace the role of physicians but will provide them with highly accurate tools to detect disease, stratify risk in an easy-to-understand manner, and optimize patient-specific treatment and further tests
Piotr Slomka
It is clear that the implementation of AI in practice will require interdisciplinary collaboration in which radiology experts have a significant role to play.18 In addition, it will be crucial to demonstrate the benefit of each new algorithm and account for the demands of licensing procedures and technological standards. That said, however, it is highly probable that advanced AI methods will set a benchmark in diagnostic imaging, allowing not only higher automation and productivity, but also an unprecedented use of quantitative imaging data beyond the limits of human cognition. “These upcoming developments will not replace the role of physicians but will provide them with highly accurate tools to detect disease, stratify risk in an easy-to-understand manner, and optimize patient-specific treatment and further tests,” Piotr Slomka of Cedars-Sinai Medical Center in Los Angeles and his colleagues write in a recent expert review.19
Specifically, in areas, such as cardiac imaging, that are already quantitatively oriented, the adoption of AI may be particularly swift. In many other fields, however, AI-based algorithms could soon establish themselves as virtual “second readers,” 20, 21 thereby advancing radiology towards a more value-based and efficient delivery of care.
www.healthcare.siemens.com/artificial-intelligence
[1]Mozur, Paul (May 23, 2017) “Google’s AlphaGo Defeats Chinese Go Master in Win for A.I.” https://www.nytimes.com/2017/05/23/business/google-deepmind-alphago-go-champion-defeat.html?module=ArrowsNav&contentCollection=Business%20Day&action=keypress®ion=FixedLeft&pgtype=article (accessed July 13, 2017)
[2] Sardanelli F (2017) Trends in radiology and experimental research. European Radiology Experimental 1:1 (DOI: 10.1186/s41747-017-0006-5)
[3] The Economist (2017) The future of healthcare – Realities or science fiction? http://thefutureishere.economist.com/thefutureofheathcare-infographic.html (accessed October 9, 2017)
[4] Signify Research (2017) Machine Learning in Medical Imaging – World Market Report
[5] Ridley, Erik L. (June 1, 2017) “SIIM: AI poised to enhance all aspects of radiology”.
http://www.auntminnie.com/index.aspx?sec=ser&sub=def&pag=dis&ItemID=117495 (accessed July 13, 2017)
[6]The Royal College of Radiologists (2016) Clinical radiology UK workforce census 2015 report. https://www.rcr.ac.uk/system/files/publication/field_publication_files/bfcr166_cr_census.pdf (accessed July 13, 2017)
[7]Choi E, Schuetz A, Stewart WF, Sun J (2016) Medical Concept Representation Learning from Electronic Health Records and its Application on Heart Failure Prediction. https://arxiv.org/abs/1602.03686 (accessed October 9, 2017)
[8]Gillies RJ, Kinahan PE, Hricak H (2016) Radiomics: Images Are More than Pictures, They Are Data. Radiology 278:563-77
[9]Radiology Quality Institute (2012) Diagnostic Accuracy in Radiology: Defining a Literature-Based Benchmark. http://www.radisphereradiology.com/wp-content/uploads/Diagnostic-Accuracy-in-Radiology (accessed July 14, 2017)
[10]van Ginneken B (2017) Fifty years of computer analysis in chest imaging: rule-based, machine learning, deep learning. Radiol Phys Technol 10:23-32
[11]Lee JG, Jun S, Cho YW et al. (2017) Deep Learning in Medical Imaging: General Overview. Korean J Radiol 18:570-584
[12]He K, Zhang X, Ren S, Sun J (2015) Deep Residual Learning for Image Recognition. https://arxiv.org/abs/1512.03385 (accessed July 16, 2017)
[13]Lakhani P, Sundaram B (2017) Deep Learning at Chest Radiography: Automated Classification of Pulmonary Tuberculosis by Using Convolutional Neural Networks. Radiology. April 24, 2017. doi: 10.1148/radiol.2017162326 (Epub ahead of print)
[14]Cheng JZ, Ni D, Chou YH et al. (2016) Computer-Aided Diagnosis with Deep Learning Architecture: Applications to Breast Lesions in US Images and Pulmonary Nodules in CT Scans. Sci Rep 6:24454
[15]Akkus Z, Galimzianova A, Hoogi A (2017) Deep Learning for Brain MRI Segmentation: State of the Art and Future Directions. J Digit Imaging. June 2, 2017. doi: 10.1007/s10278-017-9983-4 (Epub ahead of print)
[16]Motwani M, Dey D, Berman DS et al. (2017) Machine learning for prediction of all-cause mortality in patients with suspected coronary artery disease: a 5-year multicentre prospective registry analysis. Eur Heart J 38:500-507
[17]Signify Research (2017) Machine Learning in Medical Imaging – World Market Report
[18]Kohli M, Prevedello LM, Filice RW, Geis JR (2017) Implementing Machine Learning in Radiology Practice and Research. AJR Am J Roentgenol 208:754-760
[19]Slomka PJ, Dey D, Sitek A et al. (2017) Cardiac imaging: working towards fully-automated machine analysis & interpretation. Expert Rev Med Devices 14: 197-212
[20]Zhao Y, de Bock GH, Vliegenthart R et al. (2012) Performance of computer-aided detection of pulmonary nodules in low-dose CT: comparison with double reading by nodule volume. Eur Radiol 22:2076-84
[21] Venkatesh SS, Levenback BJ, Sultan LR et al. (2015) Going beyond a First Reader: A Machine Learning Methodology for Optimizing Cost and Performance in Breast Ultrasound Diagnosis. Ultrasound Med Biol 41:3148-62
27.02.2018