Article • Parsimony in genetic data study
Simplifying what is complex
Genetics stepping ahead as Spain-based researchers develop biomedical robots that can help in the study of rare diseases, neurodegenerative disorders and cancer, as well as the creation of orphan drugs.
Report: Mélisande Rouger
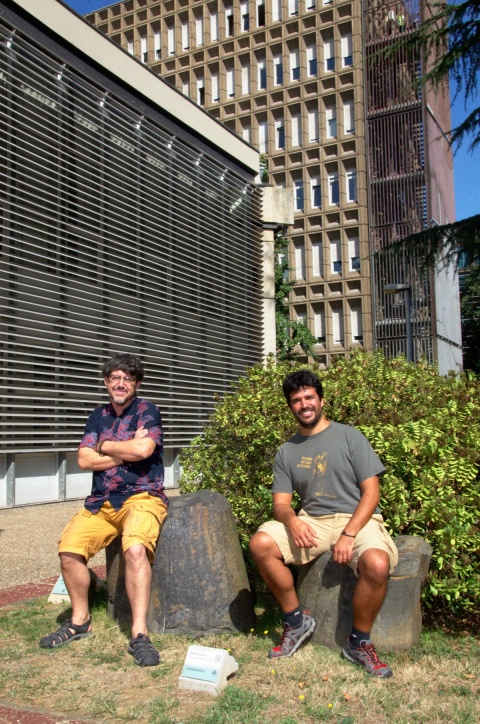
Predicting disease development and anticipating adequate treatment may appear to be as uncertain as gazing into a crystal ball. But, what if there was a way to sort out and classify the myriad available genetic information to help doctors to do so? A multidisciplinary team from Oviedo University is beginning to demonstrate that possibility by using biomedical robots that can adapt and improve predictions as they unravel phenotype – how genetic information expresses itself in a given environment.
‘Searching for classifications that can optimally explain gene expression is a little bit like trying to open a safe deposit box with multiple combinations. We are working with 50,000 genetic probes in cohorts of 100 or 200 patients at a time; this means we are dealing with an extremely high level of indetermination. We need tools that take into account the degree of uncertainty contained in phenotype prediction and understand that genetic networks have their own structure,’ explained Professor Juan Luis Fernández-Martinez, Head of Inverted Problems at the Mathematics Department in Oviedo University.
Combining knowledge from applied mathematics, statistics and computational science, since 2010 Fernández-Martinez and team have been designing biomedical robots with Biomodels, a company headed by Dr Steve Sonis from Harvard University and Brigham and Women Hospital in Boston. Their robots are able to learn from complex data sets and help doctors make decisions and plan personalised treatment according to a patient’s needs; in a word, they enable personalised medicine.
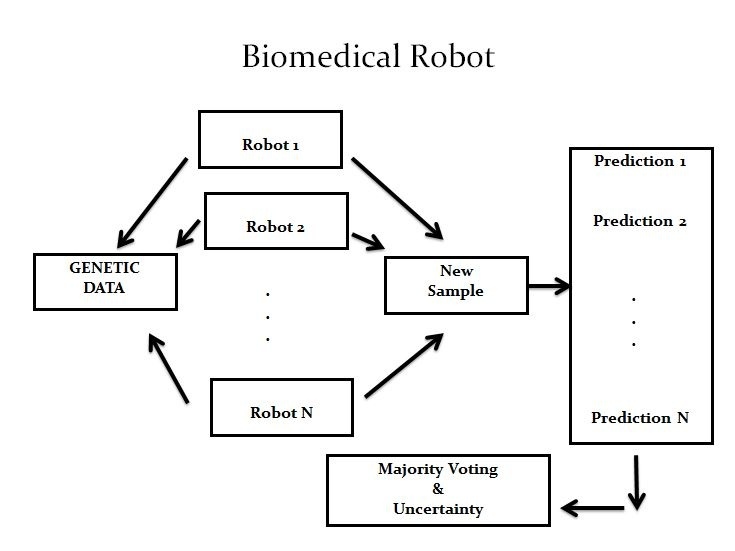
The principle behind designing such machines is simpler than one might have thought. ‘People believed they needed highly complex computers to treat so much data, but we actually did it with a pretty simple model. Basically, we applied the concept of parsimony. Uncertainty comes from ambiguity in knowledge, so it’s interesting to look at things through that angle and simplify what is complex,’ Fernández added.
The engineer and his colleagues believe the machines will not only help in the study of rare and neurodegenerative disease and cancer, but also in designing orphan drugs and new treatments, and tailoring therapy to each patient. The team has just published a study in the Journal of Computational Biology, in which they show robotic potential in chronic lymphocytic leukaemia, inclusion body myositis and amyotrophic lateral sclerosis.
In leukaemia, the researchers have highlighted the relevance of Lipoprotein lipase, an enzyme that decomposes triglycerides in free fatty acids and glycerol. The team has also discovered the importance of certain genes and how viruses and bacteria influence the genesis of myositis, an autoimmune disease affecting the muscular system, by showing how the immune system mistakes the organism’s proteins with those of the invader.
Biomedical robots have also been able to underline the importance of caspases, a group of proteins controlling programmed cell death and which have been related to mutations in gene SOD1, a cause for amyotrophic lateral sclerosis. All these findings still need clinical validation, Fernández-Martinez stressed.
Meanwhile, the Oviedo team has developed tools that can help differentiate between genes called headers, which are responsible for most of the phenotype, and helpers, adjuvant genes that bring further detailed information on discrimination. Researchers have applied this method to a toxicity study and will publish results soon on Parkinson and Alzheimer’s disease from the so-called Finisterrae project (http://www.slideshare.net/ifalberti/effective-analysis-of-biomedical-big-data-for-the-optimisation-of-drugs-for-rare-and-neurodegenerative-diseases-and-cancer).
The Oviedo researchers also recently published a paper questioning most of what has been said so far on microarray pre-processing techniques by showing their impact in biological pathways (Impact of Microarray Pre-processing Techniques in Unravelling Biological Pathways, Journal of Computational Biology, http://online.liebertpub.com/doi/abs/10.1089/cmb.2016.0042).
‘Genetics is still an understudied field and gene function is often misunderstood. Our long-term goal is to make a genetic test as common as a blood test,’ Fernández-Martinez said. ‘We will multiply cooperation projects with the medical field to do so.’
Profile:
Trained as a petroleum engineer in Paris (1988) and London (1989), and after years as an IT software engineer in France, in 1994 Juan Luis Fernández-Martínez gained a PhD in mining engineering from the University of Oviedo, in Spain. He joined the university’s mathematics department and became Professor of applied mathematics. During 2008-2010 he was a visiting and research professor at UC Berkeley-Lawrence Berkeley Laboratories and Stanford University. His expertise includes cooperative global optimisation methods, with applications in oil and gas, biometry, finance and biomedicine – in which he aims to design biomedical robots in translational medicine for diagnosis, prognosis, and treatment optimisation. The Finisterrae project particularly aims to find effective solutions for rare and neurodegenerative diseases, as well as various types of cancer.
09.01.2017