Pathology
Unlocking imaging potential
Automated image analysis shows significant potential within histopathology to help identify novel and subtle prognostic features. UK expert Dr Peter Caie also believes such image analysis can turn aspects of histopathology from a traditionally semi-quantitative field into a fully quantifiable and standardised science. However, he also points out that challenges remain before the full potential is seen within digital pathology.
Report: Mark Nicholls
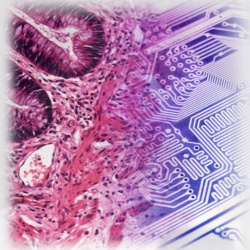
During the Digital Pathology Conference to be held this December in London, Caie will outline the advances in the ‘Profiling Big Data Histopathology through Image Analysis’ session.
As a Senior Research Fellow working on Digital and Systems Pathology at the University of St Andrews in Scotland, Dr Caie’s aim is to demonstrate how complex image analysis of digital pathology specimens can now create robust hierarchical ‘big data’.
Ahead of the conference, he told European Hospital: ‘I will be outlining how automated image analysis can not only quantify set histopathological features in a standardised and reproducible manner - such as tumour buds, lympho-vascular invasion, lymphatic vessel density and tumour nuclear morphometry - but also can be utilised as an investigative tool to identify novel prognostic or predictive features that have previously gone unnoticed or unreported.’
Caie explained that modern image analysis of digital pathology slides can now also create big data sets associated with multiple export parameters from computer segmented and classified objects within the digital tissue section.
These can be parameters associated with set histopathological features - such as their shape and extent - or features captured in an unbiased manner, where every segmentable and visible object is captured and morphometric, texture, number of objects and spatial information (such as heterogeneity, distribution, location, neighbouring to other objects) is extracted.
‘That big data must then be mined with appropriate bioinformatics to identify the significant prognostic or predictive parameters, or combination of parameters, to stratify the patient population in question,’ he pointed out.
This emerging field is termed ‘Tissue Phenomics’, a phrase first coined by Gerd Binnig, Nobel Prize winner and founder of the image analysis software company Definiens. However, Caie acknowledges that there are multiple challenges in digital pathology and image analysis. Reproducibility and validation are key to standardised quality big data histopathology, he said, and stressed that the image analysis algorithms themselves must be of a high enough quality to deal with complex and heterogeneous tissue, whereas simple algorithms may report back false results or classifications due to heterogeneous cell populations.
‘Similarly when quantifying histopathological features in the complex tumour microenvironment, image analysis may also report false positives or inaccurate parameters due to non-specific staining or autofluorescence within the tissue,’ he added.
Other challenges include the need for fast IT infrastructure to enable digital pathology to be routinely used, as well as large and secure data stores to archive the digital specimens and their associated analysis.
Another challenge, he said, is for the traditional field of pathology to accept the novel field of image analysis and ‘tissue phenomics’ and allow it to be implemented into routine clinical use.
Big data pathology has a range of benefits for clinicians and patients. Caie: “Image analysis allows reproducible and standardised reporting of biomarkers or histopathological features that negate observer variability. It can also free up a pathologists time to concentrate on complex cases if the quantification of histopathological features in more routine cases becomes automated.’
Image analysis can quantify fluorescence in situ hybridisation (FISH) and proteins across a dynamic range with the application of fluorescence, leading to more accurate patient results, as big data pathology can identify subtle or complex patterns within the tissue section which may be difficult to reproducibly identify by eye.
‘Therefore,’ Caie pointed out, ‘ it can provide the clinician with novel and significant new biomarkers to aid in clinical decision making, and the patient can receive a more personalised and informed answer to their individual case.’
The next step in big data histopathology, he suggests, is to validate the technology in large retrospective and prospective clinical trials to demonstrate its full potential.
Big data histopathology will increase in power as technology evolves. This includes multiplexing many biomarkers, which can be used to map entire pathways within a single cell.
Caie concludes: ‘Co-registering multi-omics, such as single cell transcriptomics, genomics and histopathological data, with protein biomarkers onto the same tissue section will also make for a more informative and powerful big data pathology, which again will provide insight into disease progression and biomarkers for predictive studies and drug trials.’
04.09.2015