
Image source: Eisemann N, Bunk S, Mukama T et al., Nature Medicine 2025 (CC BY 4.0)
News • Results from the PRAIM study
Mammography screening enhanced by AI
Researchers from the University of Lübeck and the University Medical Center Schleswig-Holstein (UKSH), Campus Lübeck, published a study on the successful implementation of AI in mammography screening.
The study conducted by the University of Lübeck and UKSH, Campus Lübeck, in collaboration with the company Vara, has demonstrated that artificial intelligence (AI) can improve breast cancer detection rates by nearly 18% within Germany’s Mammography Screening Program (MSP). Remarkably, this improvement does not lead to an increase in false positives or unnecessary follow-up examinations. The results of this study, published in the journal Nature Medicine, also highlight the potential of AI to reduce the workload of radiologists without compromising diagnostic quality.
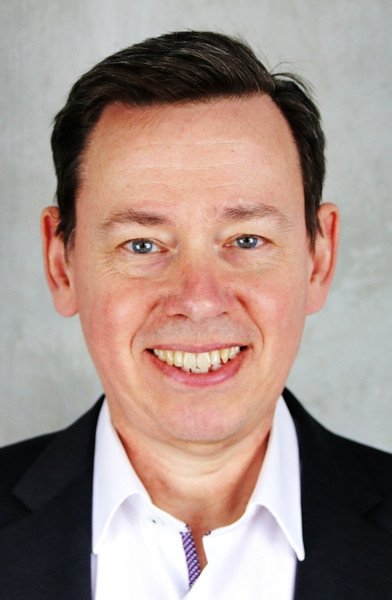
Image source: University of Lübeck; photo: private
The study, named PRAIM, evaluated data from over 460,000 women who participated in the MSP between 2021 and 2023 across 12 screening sites in Germany. Approximately half of the mammograms were analyzed using AI, while the other half were assessed through traditional double reading by radiologists. “Our initial aim was to demonstrate that AI-based evaluations are equivalent to human assessments,” explained Prof. Dr. Alexander Katalinic, principal investigator and Director of the Institute of Social Medicine and Epidemiology at the University of Lübeck and UKSH, Campus Lübeck. “However, the findings exceeded our expectations: AI significantly improves breast cancer detection rates.”
The study revealed that AI identified 6.7 cases of breast cancer per 1,000 women screened, compared to 5.7 cases per 1,000 detected through traditional methods. This equates to one additional cancer case detected per 1,000 women screened. Importantly, the rate of women referred for further testing remained stable, with 37.4 per 1,000 for AI assessments compared to 38.3 per 1,000 for traditional double readings. Stefan Bunk, Chief Technology Officer of Vara, emphasized the global significance of these findings: “The PRAIM study highlights the immense potential of AI to enhance screening programs worldwide. This evidence will elevate discussions about integrating AI into healthcare systems to a new level.”
Recommended article
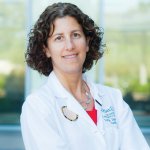
News • BAC as predictor of cardiovascular disease
Mammograms can detect more than breast cancer, study finds
Measuring the calcium build up in the arteries of the breast, researchers have developed an AI-generated score for predicting cardiovascular disease in women from their mammograms.
Another key finding of the study is the potential for AI to improve efficiency in breast cancer screening. Simulations suggest that if all cases flagged as normal by AI were not reviewed by human readers, the breast cancer detection rate would still be 16.7% higher. Additionally, the number of unnecessary recalls could be reduced by 15%.
Given that radiologists in Germany currently analyze 24 million individual images annually, the implementation of AI could significantly alleviate their workload. “We hope that the higher detection rates enabled by AI will improve outcomes for women with breast cancer. This will be the focus of future investigations,” said Prof. Katalinic.
Breast cancer is the most common cancer among women in Germany, with 78,000 new cases diagnosed each year. The MSP, aimed at early detection, screens over 3 million women aged 50 to 75 annually. Despite the high accuracy of double readings, some breast cancer cases remain undetected. AI-based systems have the potential to address this diagnostic gap while simultaneously reducing the burden on radiologists.
The PRAIM study represents a significant step forward in integrating AI into clinical practice. Its findings underscore the transformative potential of AI to enhance cancer detection, improve efficiency, and ultimately contribute to better outcomes for patients. Future research will focus on evaluating the long-term impact of AI on patient prognosis and its integration into routine clinical workflows.
Source: University of Lübeck
10.01.2025