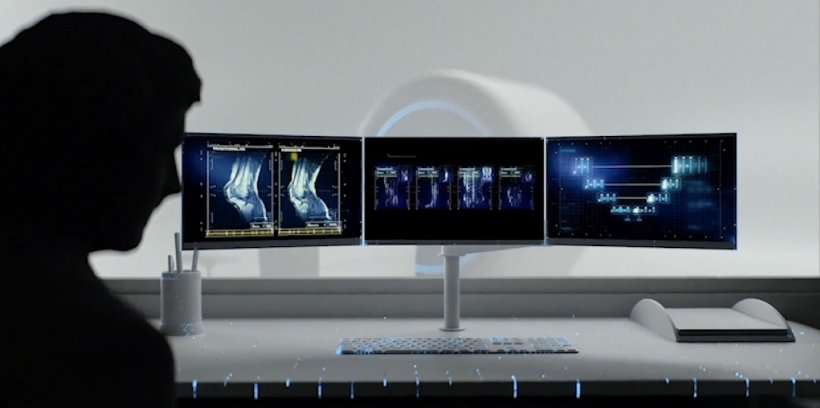
Image source: FAIR / NYU
Article • 'Thumbs up' for image reconstruction
Facebook AI accelerates MRI exams
Artificial intelligence (AI) image reconstruction dramatically reduces magnetic resonance imaging (MRI) scan time, according to new research.
Report: Cynthia E. Keen
The first clinical study comparing AI-accelerated knee MRI scans with conventional scans shows that the AI scans are not only diagnostically interchangeable with conventional ones, but also produce higher quality images. Results of this interchangeability study represent a major milestone in a joint initiative launched by NYU Langone Health of New York City and the Facebook Artificial Intelligence Research (FAIR) group in 2018 to accelerate the MRI scanning process.
The research was published in the American Journal of Roentgenology.
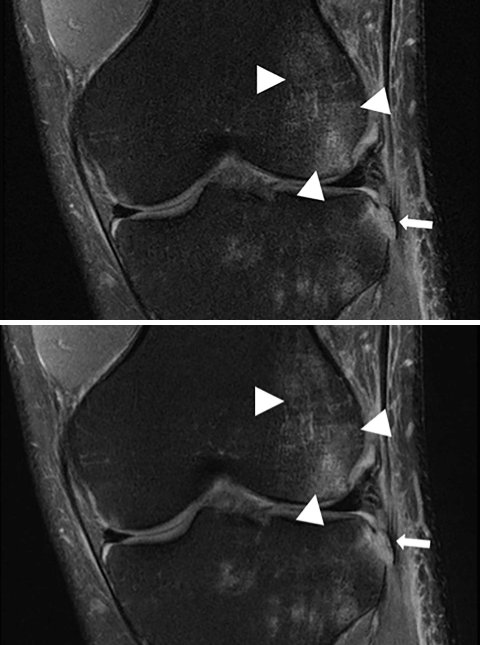
Image source: FAIR / NYU
AI-accelerated protocol image reconstruction has the ability to create diagnostic quality images using a fraction of raw acquisition data compared to conventional techniques. For the interchangeability study, the diagnostic quality of AL-DL reconstructed images using only 25% of raw data of 108 MRI knee exams performed on a 3T scanner (Siemens Healthineers) were assessed and compared with conventionally acquired and processed images using a standard clinical MR protocol. The AI reconstructed images were accelerated four-fold, because only 25% of the raw data used in fully sampled images were utilised
Six musculoskeletal radiologists evaluated each image set for the presence of meniscal tears, ligament abnormalities, chondral defects, and subchondral bone marrow signal abnormalities, scoring them on a one to four normality scale. The radiologists also rated images for sharpness, subjective signal-to-noise ratio (SNR), the presence of artifacts, and overall image quality on a four-point scale. They were also asked to identify the 216 exams as being either conventional exams or those created by the AI-accelerated protocol.
Accelerated sequence better than clinical sequence
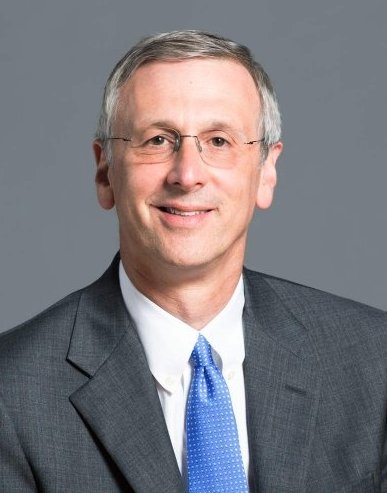
‘The study demonstrated that the AI-accelerated images were diagnostically interchangeable with the conventional images,’ writes lead author Michael P Recht MD, Louis Marx Professor and Chair of Radiology, and co-researchers. ‘Results showed that interchanging the sequences would result in discordant clinical opinions no more than 4% of the time for any feature evaluated. Moreover, the accelerated sequence was judged by all six readers to have better quality than the clinical sequence.’
The study is important: it paves the way for continuing research of AI image reconstruction models with other types of MRI exams. It also shows that ‘fastMRI’ is creating better quality images than parallel imaging and compressed sensing techniques, which also can shorten scan time length. Compressed sensing techniques tend to preserve SNR better than parallel imaging but compression algorithms tend to oversimplify image content, resulting in residual blurring and a loss of realistic image textures.
Staff at the Center for Advanced Imaging Innovation and Research (CAI2R) of NYU School of Medicine’s radiology department and Facebook AI initiated the collaborative fastMRI research project, aiming to enable AI to accelerate MRI scan acquisition by up to 10 times. Such a dramatic time reduction could make MRI more feasible for emergency patients, claustrophobics or patients unable to stay still or hold breath, and children. MRI suite throughput could significantly increase.
The challenges of building a neural network and fastMRI model require a neural network capable of effectively and reliably bridging the gaps in scanning data with extreme accuracy, without creating missing or incorrectly modelled pixels
Nafissa Yakubova
The two organisations had complementary aims. Since 2016, CAI2R had been investigating deep learning as a method to accelerate MRI reconstruction, and the Facebook group was looking for AI and medical imaging projects that could have a significant real-world impact. ‘Facebook AI has some of the world’s best computer vision and AI talent and a track record of solving difficult AI problems through fundamental open research at scale,’ Nafissa Yakubova, program manager Facebook AI, told Healthcare in Europe. ‘The NYU Langone Health team are expert clinical radiologists, leaders in research advancing medical imaging technology, and experienced in machine learning. Additionally, they had a biomedical imaging centre dedicated to advancing MRIs.’ They had worked on accelerating MRI reconstruction before this joint project began, she added.
The team trained AI models with a data set of images from 10,000 deidentified clinical knee MRI cases, which was open sourced by NYU Langone at the start of the project. After building a neural network, the researchers used the knee data set to train it to create diagnostic quality images using only 25% of the raw data. ‘The challenges of building a neural network and fastMRI model require a neural network capable of effectively and reliably bridging the gaps in scanning data with extreme accuracy, without creating missing or incorrectly modelled pixels. Additionally, the images created must be visually indistinguishable to not have an unfamiliar appearance that could distract interpreting radiologists,’ Yakubova noted.
Thousands of model variations
‘The fastMRI team experimented with thousands of model variations. The final model reconstructs MR images directly from under-sampled raw data, using multicoil under-sampled k-space data and applying 12 cascades, a sequence of 12 refinement steps. The sequence of cascades allows the network to iteratively fill in missing data points. Low noise levels were added to the images to enhance perceived sharpness and make the images visually appear more similar to the AI-reconstructed ones.’
Recht told Healthcare in Europe that additional testing includes prospective under-sampling, in which patients are being scanned twice, once as a full scan, followed by an accelerated scan that captures less data. Interchangeability studies for accelerated MRIs of brain and abdominal scans are being planned, as well as a multi-site, multi-vendor study for knee MRIs. ‘Last year,’ Recht said, ‘we encouraged the AI and medical research community to use the open source fastMRI knee dataset to train their models, and then evaluated their results on a challenge test set.’ Participation was strong, so a second challenge, using the open-source fastMRI brain scan dataset is now on. ‘By open-sourcing fastMRI,’ he concluded, ‘we’re empowering other researchers to build on our work and enabling hardware vendors to test our code on their machines and move forward with submitting for regulatory approvals,’
21.01.2021