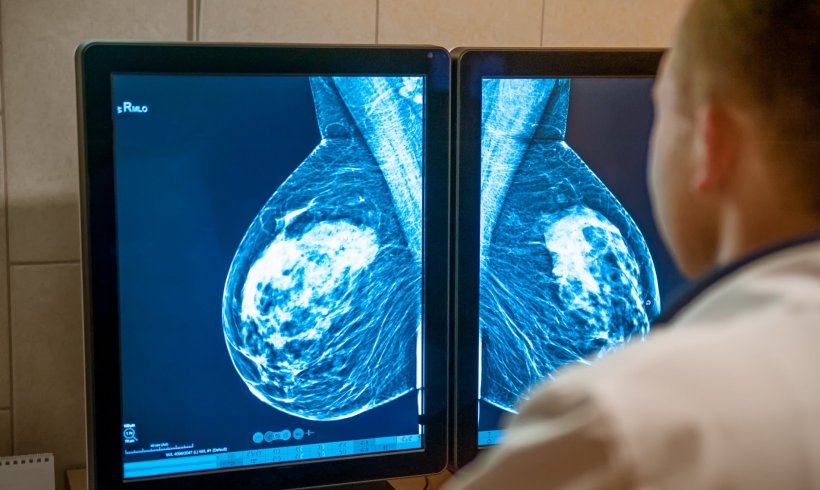
© okrasiuk – stock.adobe.com
Article • Lesion detection and mammographic texture analysis
Dual AI model to optimize breast cancer risk assessment
A team of Danish and Dutch researchers has combined an AI diagnostic tool with a mammographic texture model to improve the assessment of short- and long-term breast cancer risk. This new approach represents a significant step forward in refining the ability to predict the complexities of breast cancer risk.
Report: Bernard Banga
One in ten women will develop breast cancer at some point in their life. It is the most common cancer in women, and is diagnosed primarily in patients over the age of 50. Current screening programs use mammography as the primary diagnostic tool for detecting breast cancer at an early stage, but some lesions still remain difficult for radiologists to identify. In particular, 55% of cases are accompanied by the presence of microcalcifications: tiny spots of calcium deposits, often no more than 0.1 mm in size, localized or broadly diffused in the breast area. These calcifications are frequently associated with premalignant and malignant lesions. At present, the majority of breast cancer screening programmes base a woman’s estimated lifetime risk of developing breast cancer on the same standard protocols.
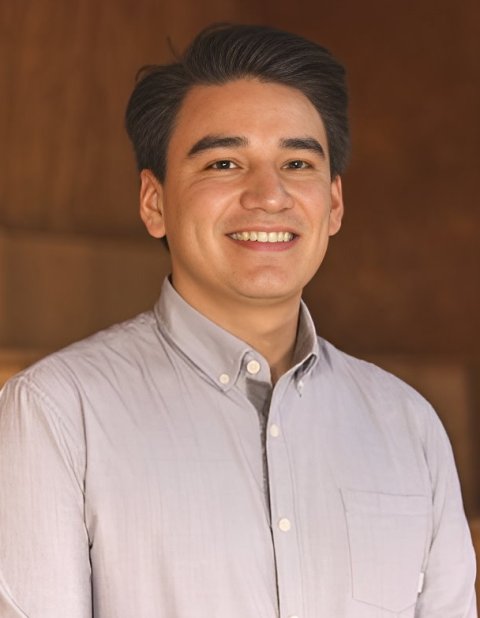
Artificial intelligence (AI) can be used ‘for the purpose of diagnosing breast cancer earlier by automatically detecting breast cancers in mammograms and measuring the risk of future breast cancer,’ said Dr Andreas D. Lauritzen, PhD, from the Department of Computer Science at the University of Copenhagen in Denmark. His team collaborated with researchers from the Department of Radiology and Nuclear Medicine at Radboud University, Nijmegen, in the Netherlands, on a project to combine two kinds of AI tools to leverage the respective strengths of both approaches: diagnostic models to estimate short-term breast cancer risk, and mammographic texture AI models to identify breast density, an important marker for assessing long-term risk.
A retrospective study of Danish women
The team of seven researchers from Denmark and the Netherlands sought to identify whether a commercially available diagnostic AI tool and an AI texture model, trained separately and then subsequently combined, could improve breast cancer risk assessment. They used the diagnostic AI system Transpara, version 1.7.0, from the Nijmegen-based company Screenpoint Medical B.V., and the texture model consisting of the deep learning encoder SE-ResNet 18, release 1.0, which they developed themselves. ‘A Dutch training set of over 39,245 exams was used to train the deep learning models,’ said the second author of the study, Dr My C. von Euler-Chelpin, associate professor at the Centre for Epidemiology and Screening, Institute of Public Health, University of Copenhagen. The short- and long-term risk models were combined using a three-layer neural network. The combined AI model was tested on a study group of more than 119,650 women included in a breast cancer screening programme in the Capital Region of Denmark over a three-year period from November 2012 to December 2015, with at least five years of follow-up data. The average age of the women was 59 years.
Key findings show benefits
According to the results of their study, published in Radiology and presented at the most recent Radiological Society of North America (RSNA) annual meeting in Chicago in November 2023, the combination model achieved a higher area under the curve (AUC) than the diagnostic AI or texture risk models separately, for cancers diagnosed within two years of screening (interval cancers) and those diagnosed after this period (long-term cancers) grouped together.
Using our model, risk can be assessed with the same performance as the clinical risk models but within seconds from screening and without introducing overhead in the clinic
Andreas Lauritzen
The combined AI model also made it possible to identify women at high risk of breast cancer, with women identified as having the 10% highest combined risk accounting for 44.1% of interval cancers and 33.7% of long-term cancers. ‘Our findings indicate that mammography-based breast cancer risk assessment is improved when combining an AI system for lesion detection and a mammographic texture model,’ concluded Dr Lauritzen and his colleagues. Using AI to identify a woman’s breast cancer risk from a single mammogram will not only result in earlier cancer detection, but will also help alleviate the strain on the healthcare system due to the worldwide shortage of specialist breast radiologists.
A rapid, single mammogram approach with no clinic overheads
‘Current state-of-the-art clinical risk models require multiple tests such as blood work, genetic testing, mammograms and filling out extensive questionnaires, all of which would substantially increase the workload in the screening clinic. Using our model, risk can be assessed with the same performance as the clinical risk models but within seconds from screening and without introducing overhead in the clinic,’ observed Dr Lauritzen in an RSNA press release.
The Danish-Dutch research team will now focus on investigating the combination model architecture and further determining whether the model adapts sufficiently to other mammographic devices and institutions. ‘Additional research should focus on translating combined risk to lifetime or absolute risk for comparison with traditional models,’ the team concluded in their paper.
Profile:
Dr Andreas David Lauritzen is a postdoctoral researcher affiliated to the Datalogisk Institut (DIKU), the Department of Computer Science at the University of Copenhagen. His expertise lies in the field of deep learning, specifically applied to medical image analysis. As part of a breast cancer risk prediction project with the Copenhagen University Hospital – Rigshospitalet he spent 8 months with the Yale University School of Medicine’s Image Processing and Analysis group. There, he honed skills crucial for applying deep learning to translational medical image analysis and high-dimensional datasets, under the guidance of Prof. Xenophon Papademetris and Dr John A. Onofrey, experts in biomedical informatics, data science and machine learning.
15.04.2024