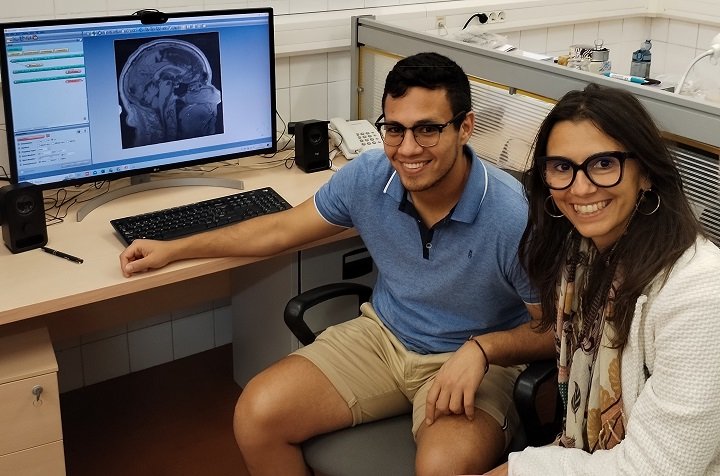
Image source: University of Barcelona
News • Bias in datasets
Diversity is a challenge for rare disease-detecting AI, study finds
Most AI techniques for rare disease diagnosis use anthropometric measurements of European origins, ignoring the genetic and morphological diversity of humans, a new study finds.
Up to 40% of rare diseases show facial alterations that enable researchers to identify some pathologies and they can even help them to establish an early diagnosis. Historically, the visual evaluation and use of some classic anthropometric measurements —diameter of the head, etc.— have enabled having an early diagnosis in rare diseases. With the most sophisticated and automated techniques —based on artificial intelligence (AI)— it is now possible to apply more objective methods in the diagnosis. However, most of the AI-generated algorithms have databases with populations of European origins and they ignore the genetic and morphological diversity of human populations of around the world.
Including populations of Amerindian, African, Asian and European origins in the AI-generated algorithms is decisive for improving the diagnostic methods of rare diseases, as stated in an article published in Nature’s journal Scientific Reports. The study is led by Neus Martínez-Abadías, lecturer at the Faculty of Biology of the University of Barcelona, and it includes the participation of experts of Ramon Llull University, the ICESI University in Colombia, the Center for Research on Congenital Anomalies and Rare Diseases (CIACER) and the Valle del Lili Foundation in Colombia.
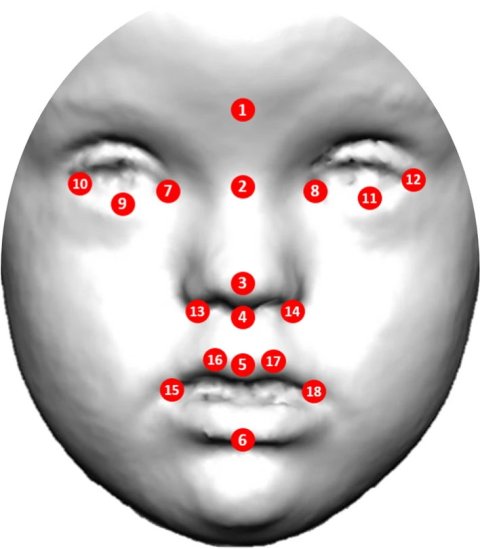
Image source: Echeverry-Quiceno et al., Scientific Reports 2023 (CC BY 4.0)
Automatic diagnosis based on artificial intelligence can reveal patterns of severe or mild dysmorphologies that are characteristic of each syndrome "but with significant differences that can be detected when a quantitative analysis of facial morphology is carried out", stresses Neus Martínez-Abadías, expert on biological anthropology and member of the Department of Evolutionary Biology, Ecology and Environmental Sciences of the UB. To address this issue, the team assessed the facial phenotypes associated with four genetic syndromes —Down (DS), Morquio (MS), Noonan (NS) and Neurofibromatosis type 1 (NF1)— in a Latino-American population with individuals that presented a great variation of miscegenation and genetic ancestry.
In order to quantitatively assess the facial features associated with each syndrome, they recorded the 2D cartesian coordinates of 18 facial landmarks in a sample of 51 people diagnosed with these syndromes and 79 controls. The facial differences were studied using the Euclidian distance matrix analysis (EDMA), based on the statistical comparison of prominent anatomical distances. “Moreover, we tested the accuracy of the diagnostic of an AI algorithm —known as Face2Gene— used in the clinical practice to identify these diseases through the analysis of facial morphometric traits. In cases of Down and Morquio syndromes, we could compare the diagnostic results between the Colombian and the European samples”, adds Martínez-Abadías.
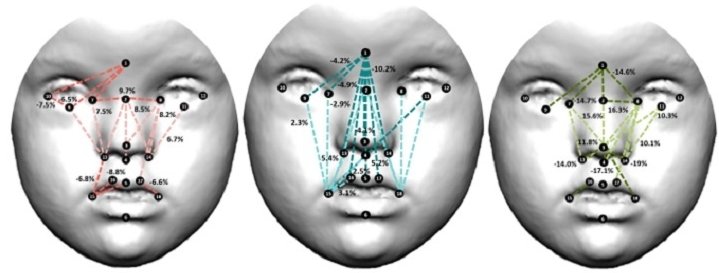
Image source: University of Barcelona; from: Echeverry-Quiceno et al., Scientific Reports 2023 (CC BY 4.0)
According to the results, people diagnosed with DS and MS presented the most severe facial dysmorphologies, with 58.2% and 65.4% of facial traits significantly different in people diagnosed with these conditions regarding the control population. The phenotype was lighter in NS (47.7%) and not significant in NF1 (11.4%). The diagnostic accuracy of the deep learning automated algorithm used in the study was very high in the case of DS and very low (less than 10%) in MS and NF1. “Each syndrome presented a characteristic facial pattern, which supports the potential capacity of facial biomarkers as diagnostic tools. In general, the observed traits coincided with those described in the library based on European populations. However, specific traits of the Colombian population were detected for each syndrome”, notes Luis Miguel Echevverry, doctoral student of Biomedicine at the UB and first author of the article.
Developing unbiased predictive models is crucial to support doctors in their decision-making and provide an accessible, universal, and effective technology for all human populations
Luis Miguel Echevverry
Compared to an European sample, the study reveals that, despite the diagnostic accuracy for Down syndrome was 100% in both populations, the variation in the average facial similarities between people diagnosed with DS and the automated algorithm model was significantly larger in the Colombian sample. In the case of Noonan syndrome, the accuracy was significantly lower, going from 66.7% in the Colombian sample to 100% in the European sample. Furthermore, it was observed for all syndromes, mixed-race individuals were precisely those with the lowest facial similarities.
In the case of Noonan syndrome, the accuracy was significantly lower, going from 66.7% in the Colombian sample to 100% in the European sample. Furthermore, it was observed that for all syndromes, mixed-race individuals were precisely those with the lowest facial similarities.
Therefore, AI-based automatic diagnosis algorithms are optimized in European populations but do not work with the same accuracy in mixed populations of different genetic origins. "Developing unbiased predictive models is crucial to support doctors in their decision-making and provide an accessible, universal, and effective technology for all human populations", the team points out. "With a greater understanding of the facial dysmorphologies specific to each syndrome and the diversity of the population, it is possible to improve diagnosis rates, try to reduce the personal and family odyssey to find a diagnosis and thus be able to design earlier treatments for people affected by rare minority pathologies. This is particularly relevant in countries with scarce resources and more difficulties in carrying out other diagnostic tests based on genetic and molecular techniques which are much more expensive", conclude the experts.
Source: University of Barcelona
11.05.2023