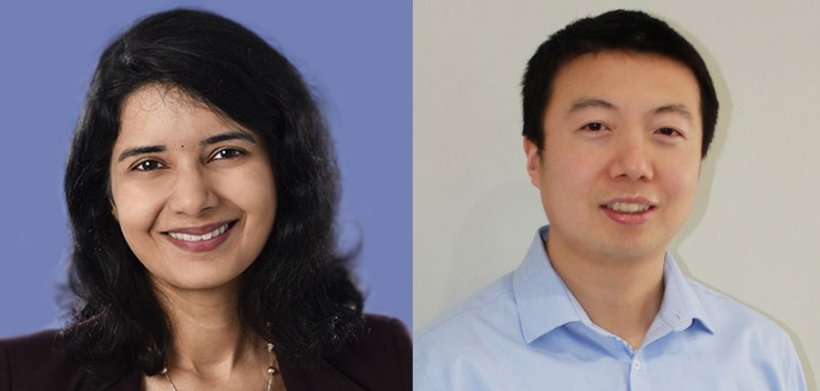
Photos: Courtesy Drs. Bhat and Wang
News • Outcome prediction
Deep learning to maximize lifespan after liver transplant
Researchers from the Canadian University Healh Network (UHN) have developed and validated a deep learning model to predict a patient's long-term outcome after receiving a liver transplant.
First of its kind in the field of Transplantation, this model is the result of a collaboration between the Ajmera Transplant Centre and Peter Munk Cardiac Centre (PMCC). The study, published in Lancet Digital Health, shows it can significantly improve long-term survival and quality of life for liver transplant recipients. "Historically, we have seen good advances in one-year post-transplant outcomes, but survival in the longer term hasn't significantly improved in the past decades," explains Dr. Mamatha Bhat, a hepatologist with the Ajmera Transplant Centre at UHN, and co-senior author of the study. "This model can guide physicians and help anticipate when and how complications may rise. It can really be paradigm-changing in how we support liver transplant recipients in personalizing their care and helping them live better and longer."
For liver transplant recipients, long-term survival beyond one-year is significantly compromised by an increased risk of cancer, cardiovascular mortality, infection and graft failure. Clinical tools to identify patients at risk of these complications are limited.
Deep learning enables timely processing of large-scale datasets, finding patterns and signals that can aid clinicians in better predicting the clinical outcomes and creating specific treatment recommendations
Bo Wang
This model will help clinicians enhance post-liver transplant care using machine learning, allowing them to identify potential risks when formulating patient-specific treatment plans. The study results show this model is more than 80 per cent accurate in predicting potential complications for liver transplant recipients at any point post-transplantation, based on their medical history and comparing to the millions of data points compiled using artificial intelligence (AI). "Deep learning enables timely processing of large-scale datasets, finding patterns and signals that can aid clinicians in better predicting the clinical outcomes and creating specific treatment recommendations," says Dr. Bo Wang, AI Lead at the PMCC, and co-senior author of this study.
The algorithms for this model were created based on the Scientific Registry of Transplant Recipients (SRTR) – a national medical database in the United States, with data from more than 42,000 liver transplant recipients. They were then validated using the local dataset from UHN's Ajmera Transplant Centre, which had over 3,200 cases. The research team now plans to share this model with clinicians so that it can be used across the world. Work is also in progress to evaluate what are the best formats to streamline its use, either developing a software or mobile application.
Source: University Health Network
15.04.2021