Article • Digital Pathology
Deep Blue meets Hematoxilin and Eosin
In the nineties Deep Blue, the famous chess computer, defeated Kasparow. Only a year ago Google’s Deepmind managed to master the ancient Chinese Go, known for its utmost complexity.
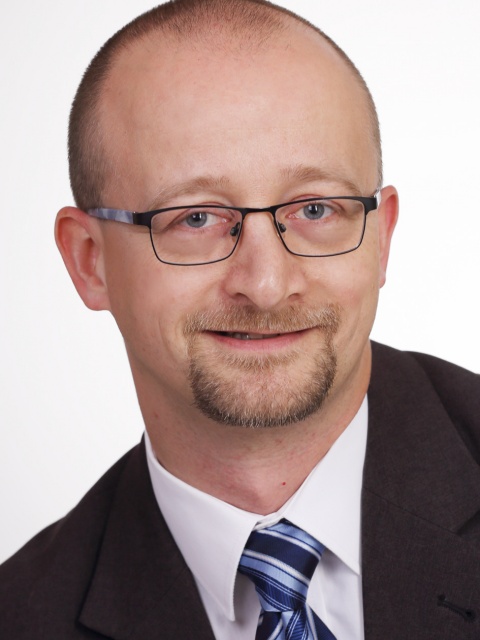
Today, machine learning is a topic in the mainstream media and we are awaiting computers to master the complexity of reading tissue sections. ‘Is there a new Deep Violet out there?’ asks computer scientist Dr Christian Münzenmayer.
CT, MRI or PET scanners have placed radiology at the forefront of digital imaging in medicine. Fully integrated workflows within clinical information systems are tightly connected to large picture archiving and computer-assisted diagnostic algorithms, to detect automatically lung or breast cancer, provide second opinions, support digital workflows, thus paving the way for other disciplines. Hence, current clinical pathology can be compared to radiology 20 years ago, when X-ray images were reviewed on light boxes, film was physically carried and stored in large archives. Similarly, clinical histopathology still works with tissue samples prepared on glass slides, reviewed visually under microscopes and stored and transported in boxes.
There is, of course, the complexity of three-dimensionality, of sectioning and staining, of variability in tissue, reagents and processes. Nevertheless, digitisation of pathology has begun.
Modern microscopy-based slide scanners are a key technology for digital pathology, which are e.g. offered by companies such as 3DHistech and Sysmex, Hamamatsu, Leica Biosystems, Olympus and others. Large-scale integrated solutions from companies such as Omnyx (GE/UPMC), Ventana Medical Systems (Roche) and Philips offer a capacity to scan hundreds of thousands of slides annually. Technical requirements on handling, transportation and archiving of such huge data is still among the major cost drivers slowing digital pathology adoption. Increasing demand of about 8-10% more slides per year and the increasing shortage of pathologists drive the need for automation and efficiency.
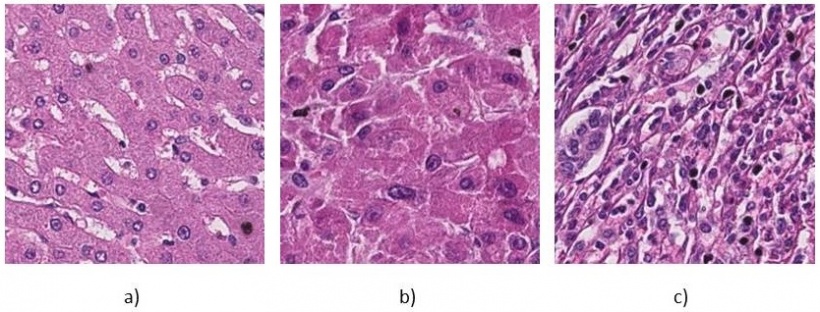
Applications
Typical applications for image analysis in digital pathology are marker quantification, biomarker research and workflow improvements. The classic example for marker quantification is proliferation counting for breast cancer diagnosis. Tissue sections immunohistochemically stained with an antigen to Ki67 display proliferating cells in brown tones against the normal cell nuclei in blue. The tumour region may be selected within the slide or using serial sections the tumorous region may be detected in the hematoxylin and eosin stain (H&E stain) and co-registered to the Ki67. An automated counting software, e.g. as developed at Charité, will obviously come up with more accurate and quantitative results compared to a pathologist’s estimate, provided standardisation, sample and staining quality is ensured.
Biomarker research
Precision medicine, personal diagnostics and development of companion diagnostics basically drive biomarker research. Tissue micro arrays (TMA) are among the most important methods for parallel processing of hundreds of samples to detect and verify specificity of biomarkers for cancer subtypes. Automated support to generate high-quality TMA with devices such as the 3DHistech TMA Grandmaster and services, like the ‘next-generation TMA’ (ngTMA) offered by the Translational Research Unit at the Bern Institute of Pathology, will further drive the demand for automated processing of digitised samples.
Parameters extracted from the digital whole-slide images (WSI) provide information about phenotypes, in addition to what next generation sequencing (NGS) can offer. Thus, quantification of morphology and biomarker expression produces real big data – a specialty of the industry leaders Definiens.
Estimation of infiltration depth, detection of mitotic activity and spreading tumour cells are important for colon cancer diagnosis. Being a new biomarker for metastatic activity, the identification and quantification of tumour buds is gaining importance and is an active clinical research entering routine diagnostics. Such buds are defined as single tumour cells or clusters up to five cells in the tumour stroma separating from the main tumour and may be detected in H&E or pan-cytokeratin staining.
Having high potential to stratify patients for neoadjuvant therapies, and to forecast lymph node metastasis, consensus guidelines are under development. Automated detection and quantification methods we are working on may support this process in the future.
Another important factor in prognosis and treatment aiming for precision medicine is the determination of cancer stem cells (CSC), known for their resistance to chemotherapy and involvement in tumour recurrence. Using immunohistochemistry with CSC markers like CD13, CD133 and others is one way to identify CSC (cmp).
In our work we aim to identify CSC presence on ubiquitous H&E staining as an inexpensive tool for routine histopathology based on their distinct morphological features. Applying ‘texture analysis’ and deep learning methods we reached grading accuracies in the >90%. Finally, image analysis may provide workflow improvements that will unleash gains in efficiency needed to keep pace with the ever-increasing workload in laboratories and is promoted by industry leaders such as Philips. In view of the still low number of marker quantification products cleared for clinical use and an estimated 70% percent of routine cases that are handled in H&E without further markers, there seems to be a high potential to improve routine workflows.
An automated pre-analysis can check the quality of slides for tissue folds, staining problems, artefacts or scanning faults – factors that are also important for Biobanking where Charité is active. In a fully digital routine laboratory this can be used to re-order another slide and update the work list.
Automated detection of tumorous regions in the H&E also can be used to order other stains according to pre-defined panels and thus optimise time and resource usage to present the pathologist with a ‘complete’ case, including all necessary stainings for immediate diagnostic decisions. Advance measuring and counting markers can further reduce pathologist’s idle time. Image registration can help to pre-align serial sections for intuitive navigation and provide virtual double-stainings, as provided by companies like Visiopharm or MicroDimensions.
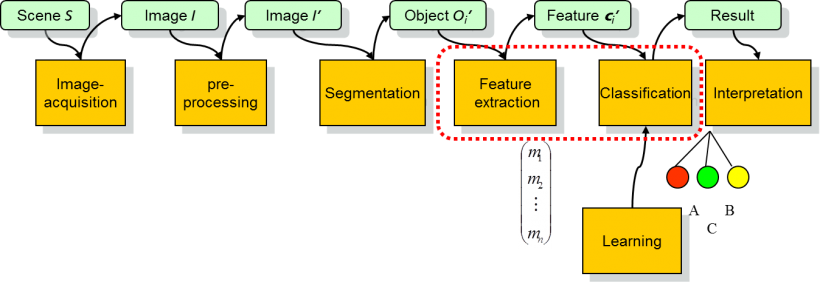
Technology
The classical approach to pattern recognition and image analysis works as a serial pipeline of processing steps. Today, these steps may no longer be separated so strictly and may also have feedback loops included. This pipeline starts with the sample being digitised in the scanner and results in a digital (whole slide) image for further processing. Image pre-processing as the next step typically includes geometric and colour normalisation, or deconvolution, to remedy variations in sample preparation, staining and scanning. Image alignment via so-called registration algorithms may be considered as a (fairly complex) pre-processing step.
The next step is segmentation of the WSI into separate objects, which may be slide sub-regions, cell clusters, cells or nuclei. For the automated analysis of WSI the main focus is often on the segmentation of cell nuclei and there exists little work that explicitly uses features of cytoplasm and stroma, although some researchers have hinted at the need for such features. Similar to the pathologist working from low to high magnification when analysing a slide a multi-resolution approach has been used to classify and retrieve high-resolution whole-slide histopathology images. To characterise individual objects, e.g. cells and cell nuclei classically features, mimicking cytologic features have been used.
The architecture of the tissue can be characterised by quantifying spatial distribution of nuclei implemented by mathematical graphs. Thus, the mathematical framework of graph theory can be used to extract quantitative features that are correlated with tissue structures. A complementary approach quantifies complex patterns in WSI by so-called texture analysis. Frequency analysis using wavelets and Fourier Transforms, statistical co-occurrence histograms, non-linear statistical geometrical features or local binary patterns to name just a few representatives, are powerful approaches that we combine with automated parameter optimisation and feature selection in the development of such systems. The final step in pattern recognition are the classifiers that decide, based on the object features if, for example, an image region may be considered benign or tumorous. Classical approaches are the ‘k-nearest-neighbour’, support vector machines, decision trees and neural networks. As mentioned, in recent years the so-called deep learning and convolutional neural networks (CNN) gained increasing interest and application also in digital pathology.
CNN are an extension of the self-learning artificial neural networks (ANNs), which had been an important research topic in image analysis and artificial intelligence in the mid-1990s. With the computing power of multi-core CPU, graphical processing units (GPU) and high-performance computing (HPC) combined with appropriate learning algorithms now available, such networks can be trained with far more layers in a decent amount of time. In contrast to ANNs, CNNs make use of up to twelve or more layers of data processing. Secondly, within the original ANNs, feed-forward, back-propagation architectures needed adequate and representative input data to converge to a stable and robust classification scheme. CNNs specifically incorporate this feature-extraction and selection process directly in the convolutional lower layers of the CNN and thus promise to reduce the need for application-specific and expensive application development as we could also retrace in several of our projects on histopathology, blood cell counting in bone marrow and malaria detection.
Conclusion
Undeniably, dramatic developments have occurred in recent years in machine learning and image analysis, reflected in research papers as well as high dynamics in industry – considering activities of Google, IBM Watson, Facebook, nvidia and others. This trend from the mainstream already has had a high scientific impact on digital pathology and will create new business opportunities for technology providers. Considering digital pathology, experts, industry and market studies agree that automation is one of the key drivers to adopt this technology and image analysis will play a major role in it.
Profile:
Christian Münzenmayer PhD MSc received his computational engineering degree at the German Friedrich-Alexander University Erlangen-Nuremberg and Computer Science doctorate from the University of Koblenz-Landau. At the Fraunhofer Institute for Integrated Circuits IIS in Erlangen since 2000, he became head of Medical Image Processing there in 2008. For his proposal on the automated analysis of micrographs of bone marrow smears he received the medical technology innovation prize in 2010, and the 2011 Boston-Scientific innovation award for his work on image-based classification of polyps in endoscopic images.
03.09.2017