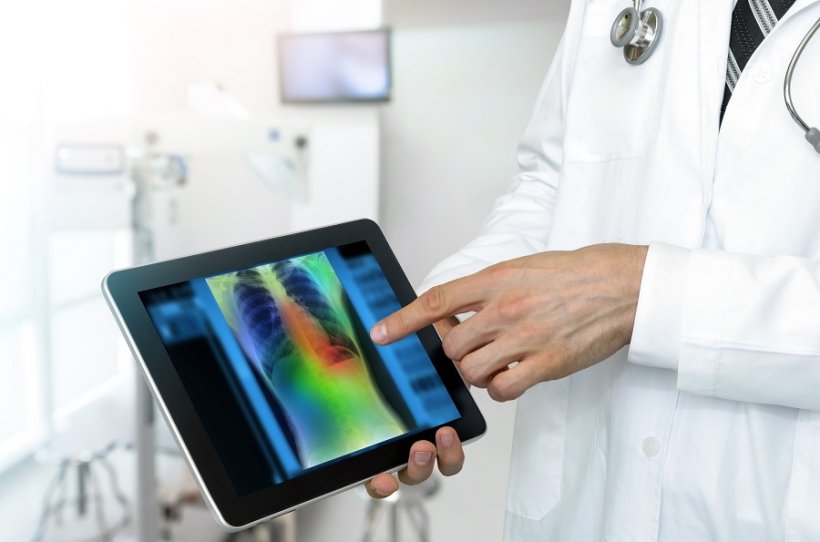
Source: Adobe Stock/zapp2photo
Article • Current and emerging imaging applications
Challenges of AI in chest radiography
Physicians use artificial intelligence-powered technology (AI) for faster and earlier detection of diseases. At ECR Overture, Dr Steven Schalekamp, PhD, of the Department of Medical Imaging at Radboud university medical center (UMC), discussed Current and emerging application of AI for chest radiography in paediatrics.
Report: Sascha Keutel
There are several challenges with current AI products in radiology, Schalekamp pointed out – one of them being mainly developed for the adult population. The Dutch expert is part of “AI for Radiology”, a non-commercial project at Radboud UMC that tracks all CE-certified AI products in radiology as well as supporting literature and research studies evaluating these algorithms.
To date, 191 AI software solutions with the CE Mark for radiology are listed in the database, with a majority designed for neuroradiology (35 percent). The second largest group is chest radiology, with 57 products from 28 vendors. However, as Schalekamp noted, none of these products were specifically designed for children.
Only one product, CAD4TB, which was developed to automatically detect tuberculosis on chest radiographs, is applicable to paediatric patients. CAD4TB was trained on chest images from children ages four and up. “It's being used in multiple, often low-resource countries where there aren’t many radiologists available,” he said.
Schalekamp pointed out that great discrepancies between children and adults were also present in research. For example, entering the search query “artificial intelligence chest” in pubmed.gov yields 2311 results – in contrast, “artificial intelligence pediatric chest” only leads to 75 hits.
Paediatric vs adult chest
Another major challenge for these AI systems is the difference between adult and paediatric thoraxes. Children have a growing chest that is constantly changing. For example, the size and proportions of an infant's chest differ from that of a young adult. There is also greater variability in patient monitoring and inspiratory levels, the radiologist said.
Moreover, in paediatric patients, clinicians are generally looking for different abnormalities than in adults, such as paediatric pulmonary sequestration and congenital pulmonary airway malformations. Schalekamp added that only few large paediatric datasets exist, partially because of less imaging being performed in children due to radiation risks.
The expert also warned that vendors often do not report whether their product applies to paediatric patients, which raises concerns over the potential for off-label use. “That can result in lower performance when using these products in the paediatric population,” he said.
To overcome some of these challenges, vendors should “aim to develop specific systems for the paediatric population. A first could be to re-train current AI systems on paediatric datato try to improve their performance and make them available for paediatric data as well.”
Recommended article
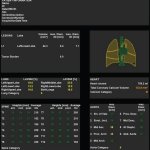
News • Diagnostic imaging
AI saves one hour of daily chest CT interpretation time
Incorporating AI support into clinical practice can reduce repetitive tasks, saving approximately one hour of chest CT interpretation time in a radiologist’s typical workday.
Commercial AI
The development of AI products in chest radiography is changing, Schalekamp pointed out. “A couple of years ago, most products only were able to detect one or two abnormalities, mainly lung cancer or pneumothorax. Now, products focus on multiple abnormalities and try to assess the whole image. There are even products available, claiming to detect up to 124 abnormalities,” the expert said.
The vendors have different ways of presenting their findings, for example with heatmaps, by drawing circles around suspicious areas or giving abnormality scores of an image. “In the literature, we see that the products get better and better,” he said.
Possible applications of AI
Aside from CAD4TB, other paediatric products are being developed. “In the near future, we can expect some products on pneumonia detection in children, but also detection of lung metastases,” Schalekamp said. “These products are already available for the adult population and could be easily transferred to the paediatric population.”Researchers in South Africa developed CAD4Kids, an algorithm aimed at detecting pneumonia, which still carries a high mortality rate in infants worldwide. The algorithm was trained on a dataset of chest x-rays from children under five years old. The system achieved reasonable sensitivity (76 percent) and specificity (80 percent), but the analysis revealed the system was still inferior to a reference observer, the expert reported.
An international group led by Merck scientists used a World Health Organization dataset of radiographs from 431 children to develop an AI algorithm for detecting severe pneumonia in children. Schalekamp said that the algorithm reached an impressive area under the curve (AUC) of 0.977 and was within the range of interobserver reliability of the reference observers in the study.
There is also a lot of research on the detection and tracking of bronchopathy (cystic fibrosis). Researchers from the University of Bordeaux and the University of Cincinnati in Ohio developed an AI algorithm to help evaluate cystic fibrosis on CT imaging in children based on a dataset of images from patients between the ages of four and 54. “They were able to demonstrate that patients on treatment on follow-up CTs had lower amounts of specific biomarkers, which was easily picked up by this AI system,” Schalekamp said.
For him, another interesting application would be the assessment of neonatal radiographs, the grading of idiopathic respiratory stress syndrome (IRDS) in premature babies, and the detection of lines and tubes in radiographs.
Schalekamp concluded that AI in thoracic radiology is well developed, but no specific applications are available for the paediatric population and that “more research and data is needed.”
More information on the the "AI for Radology" implemention guide here.
Profile:
Dr Steven Schalekamp, PhD, of the Department of Medical Imaging at Radboud university medical center (UMC) in Nijmegen, the Netherlands.
27.06.2022