News • Deep learning & CNN
Algorithm differentiates small renal masses on multiphase CT
A deep learning method with a convolutional neural network (CNN) can support the evaluation of small solid renal masses in dynamic CT images with acceptable diagnostic performance.
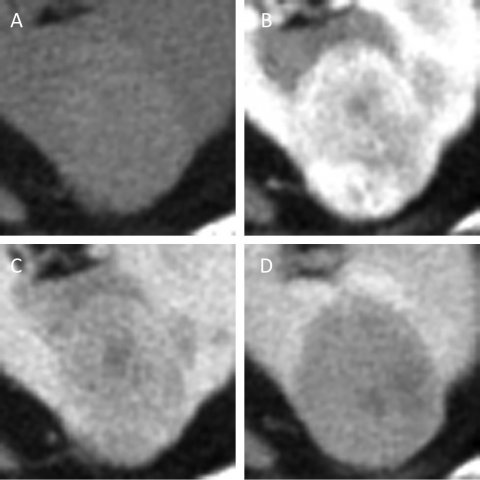
Image source: Tanaka et al., 2020; AJR
The article was published in the March issue of the American Journal of Roentgenology (AJR).
Between 2012 and 2016, researchers at Japan’s Okayama University studied 1807 image sets from 168 pathologically diagnosed small (≤ 4 cm) solid renal masses with four CT phases—unenhanced, corticomedullary, nephrogenic, and excretory—in 159 patients.
Masses were classified as malignant (n = 136) or benign (n = 32) using a 5-point scale, and this dataset was then randomly divided into five subsets.
As lead AJR author Takashi Tanaka explained, “four were used for augmentation and supervised training (48,832 images), and one was used for testing (281 images).”
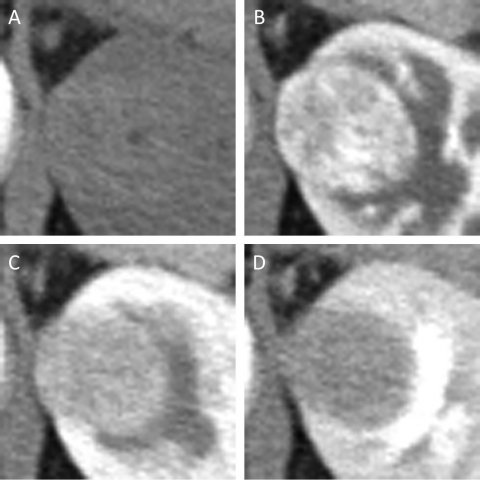
Image source: Tanaka et al., 2020; AJR
Utilizing the Inception-v3 architecture CNN model, the AUC for malignancy and accuracy at optimal cutoff values of output data were evaluated in six different CNN models. Finding no significant size difference between malignant and benign lesions, Tanaka’s team did find that the AUC value of the corticomedullary phase was higher than that of other phases (corticomedullary vs excretory, p = 0.022).
Additionally, the highest accuracy (88%) was achieved in the corticomedullary phase images. Multivariate analysis revealed that the CNN model of corticomedullary phase was a significant predictor for malignancy, “compared with other CNN models, age, sex, and lesion size,” Tanaka concluded.
Source: American Roentgen Ray Society (ARRS)
13.01.2020