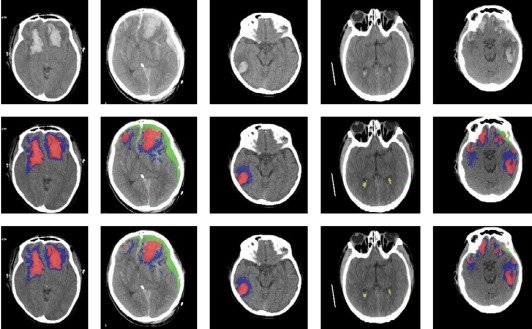
Image source: Monteiro et al., The Lancet Digital Health 2020 (CC BY 4.0)
News • Lesion differentiation
AI successfully identifies different types of brain injuries
Researchers have developed an AI algorithm that can detect and identify different types of brain injuries.
The researchers, from the University of Cambridge and Imperial College London, have clinically validated and tested the AI on large sets of CT scans and found that it was successfully able to detect, segment, quantify and differentiate different types of brain lesions. Their results, reported in The Lancet Digital Health, could be useful in large-scale research studies, for developing more personalised treatments for head injuries and, with further validation, could be useful in certain clinical scenarios, such as those where radiological expertise is at a premium.
Detailed assessment of a CT scan with annotations can take hours, especially in patients with more severe injuries
Virginia Newcombe
Head injury is a huge public health burden around the world and affects up to 60 million people each year. It is the leading cause of mortality in young adults. When a patient has had a head injury, they are usually sent for a CT scan to check for blood in or around the brain, and to help determine whether surgery is required. “CT is an incredibly important diagnostic tool, but it’s rarely used quantitatively,” said co-senior author Professor David Menon, from Cambridge’s Department of Medicine. “Often, much of the rich information available in a CT scan is missed, and as researchers, we know that the type, volume and location of a lesion on the brain are important to patient outcomes.”
Different types of blood in or around the brain can lead to different patient outcomes, and radiologists will often make estimates in order to determine the best course of treatment. “Detailed assessment of a CT scan with annotations can take hours, especially in patients with more severe injuries,” said co-first author Dr Virginia Newcombe, also from Cambridge’s Department of Medicine. “We wanted to design and develop a tool that could automatically identify and quantify the different types of brain lesions so that we could use it in research and explore its possible use in a hospital setting.”
The researchers developed a machine learning tool based on an artificial neural network. They trained the tool on more than 600 different CT scans, showing brain lesions of different sizes and types. They then validated the tool on an existing large dataset of CT scans. The AI was able to classify individual parts of each image and tell whether it was normal or not. This could be useful for future studies in how head injuries progress, since the AI may be more consistent than a human at detecting subtle changes over time. “This tool will allow us to answer research questions we couldn’t answer before,” said Newcombe. “We want to use it on large datasets to understand how much imaging can tell us about the prognosis of patients.” “We hope it will help us identify which lesions get larger and progress, and understand why they progress so that we can develop more personalised treatment for patients in future,” said Menon.
Recommended article
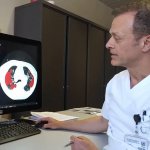
News • Imaging collaboration
AI quantifies COVID-19 in chest CT images
Hospitals and organizations worldwide joined forces with AI imaging company icometrix in a global initiative to leverage artificial intelligence (AI) in the fight against COVID-19. The multinational collaboration resulted in the development of an AI algorithm, icolung, which received CE-marking for clinical use in Europe. icolung is the first CE-marked AI solution for CT resulting from a…
While the researchers are currently planning to use the AI for research only, they say with proper validation, it could also be used in certain clinical scenarios, such as in resource-limited areas where there are few radiologists. In addition, the researchers say that it could have a potential use in emergency rooms, helping get patients home sooner. Of all the patients who have a head injury, only between 10 and 15% have a lesion that can be seen on a CT scan. The AI could help identify these patients who need further treatment, so those without a brain lesion can be sent home, although any clinical use of the tool would need to be thoroughly validated.
The ability to analyse large datasets automatically will also enable the researchers to solve important clinical research questions that have previously been difficult to answer, including the determination of relevant features for prognosis which in turn may help target therapies.
Source: University of Cambridge (CC BY 4.0)
21.05.2020