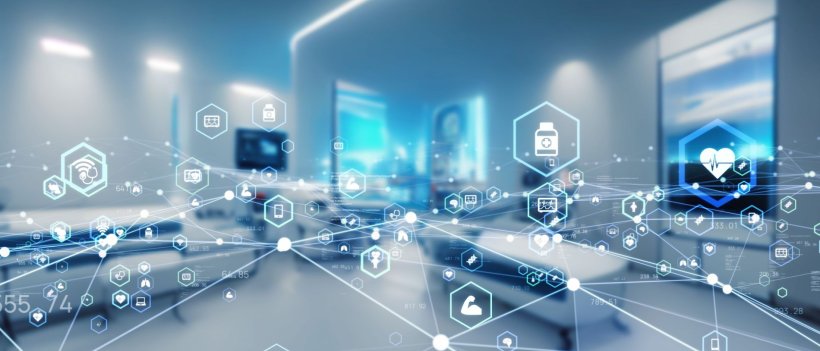
stock.adobe - metamorworks
News • AI efficiency
AI in everyday clinical practice: not always efficient
The use of artificial intelligence (AI) in hospitals and patient care is steadily increasing. Especially in specialist areas with a high proportion of imaging, such as radiology, AI has long been part of everyday clinical practice. Researchers at the University Hospital Bonn (UKB) and the University of Bonn have conducted a comprehensive analysis of existing studies on the effect of AI. They were able to show that AI does not automatically lead to an acceleration of work processes.
Their results have now been published in the journal npj Digital Medicine.
Although AI is often seen as a solution for handling routine tasks such as monitoring patients, documenting care tasks and supporting clinical decisions, the actual effects on work processes are unclear. Particularly in data-intensive specialties such as genomics, pathology and radiology, where AI is already being used to recognise patterns in large amounts of data and prioritise cases, there is a lack of reliable data on efficiency gains.
‘We wanted to find out to what extent AI solutions actually improve efficiency in medical imaging,’ explains Katharina Wenderott, lead author of the study and a doctoral student at the University of Bonn at the UKB's Institute for Patient Safety (IfPS). ’The widespread assumption that AI automatically speeds up work processes often falls short.’
Local conditions and individual work processes have a major influence on the success of implementation.
Matthias Weigl
Consistent evaluation of studies is difficult
The research team conducted a systematic review of 48 studies that examined the use of AI tools in clinical settings, particularly in radiology and gastroenterology. Of the 33 studies that looked at the processing time of work processes, 67 per cent reported a reduction in working hours, but the meta-analyses did not show any significant efficiency gains. ‘Some studies showed statistically significant differences, but these were insufficient to draw general conclusions,’ says Wenderott.
In addition, the team analysed how well AI is integrated into existing workflows. It was found that the success of implementation depends heavily on the specific conditions and processes on site. However, the heterogeneity of the study designs and the technologies used made it difficult to conduct a uniform evaluation.
‘Our results make it clear that the use of AI in everyday clinical practice must be considered in a differentiated way,’ emphasises Prof. Matthias Weigl, Director of the IfPS at the UKB, who also conducts research at the University of Bonn. ’Local conditions and individual work processes have a major influence on the success of implementation.’
The study provides important initial insights into how AI technologies can influence clinical work processes. ‘A key finding is the need for clearly structured reporting in future studies in order to better evaluate the scientific and practical benefits of these technologies,’ summarises Prof. Weigl.
Publication: Katharina Wenderott, Jim Krups, Fiona Zaruchas, Matthias Weigl: Effects of artificial intelligence implementation on efficiency in medical imaging - A systematic literature review and meta-analysis; npj digital medicine; DOI: https://doi.org/10.1038/s41746-024-01248-9
Source: University of Bonn
14.10.2024