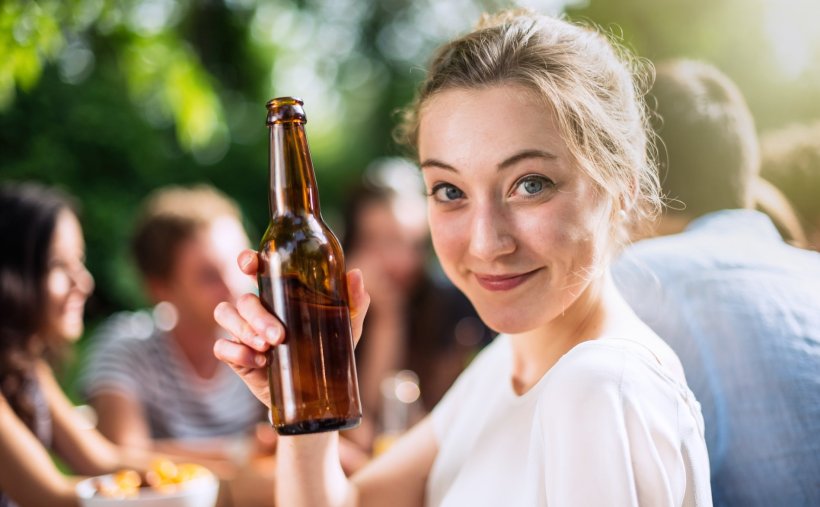
© jackfrog – stock.adobe.com
News • Misleading medical analyses
AI “predicts” beer drinking based on knee X-rays – why this is not only wrong, but dangerous
Medicine, like most fields, is transforming as the capabilities of artificial intelligence expand at lightning speed. AI integration can be a useful tool to healthcare professionals and researchers, including in interpretation of diagnostic imaging.
Where a radiologist can identify fractures and other abnormalities from an X-ray, AI models can see patterns humans cannot, offering the opportunity to expand the effectiveness of medical imaging. A study led by Dartmouth Health researchers, in collaboration with the Veterans Affairs Medical Center in White River Junction, VT, and published in Nature’s Scientific Reports, highlights the hidden challenges of using AI in medical imaging research. The study examined highly accurate yet potentially misleading results—a phenomenon known as “shortcut learning.”
These models can see patterns humans cannot, but not all patterns they identify are meaningful or reliable. It’s crucial to recognize these risks to prevent misleading conclusions and ensure scientific integrity
Peter L. Schilling
Using knee X-rays from the National Institutes of Health-funded Osteoarthritis Initiative, researchers demonstrated that AI models could “predict” unrelated and implausible traits, such as whether patients abstained from eating refried beans or drinking beer. While these predictions have no medical basis, the models achieved surprising levels of accuracy, revealing their ability to exploit subtle and unintended patterns in the data.
“While AI has the potential to transform medical imaging, we must be cautious,” said Peter L. Schilling, MD, MS, an orthopaedic surgeon at Dartmouth Health’s Dartmouth Hitchcock Medical Center (DHMC), who served as senior author on the study. “These models can see patterns humans cannot, but not all patterns they identify are meaningful or reliable. It’s crucial to recognize these risks to prevent misleading conclusions and ensure scientific integrity.”
Schilling and his colleagues examined how AI algorithms often rely on confounding variables—such as differences in X-ray equipment or clinical site markers—to make predictions rather than medically meaningful features. Attempts to eliminate these biases were only marginally successful—the AI models would just “learn” other hidden data patterns.
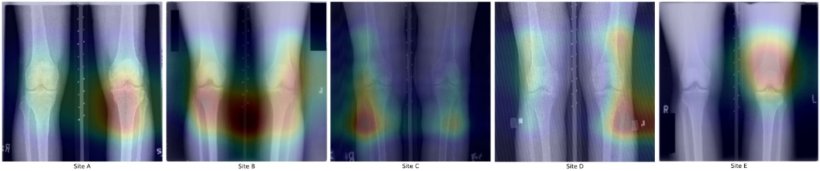
Image source: Hill BG, Koback FL, Schilling PL, Scientific Reports 2024 (CC BY-NC-ND 4.0)
The research team’s findings underscore the need for rigorous evaluation standards in AI-based medical research. Over-reliance on standard algorithms without deeper scrutiny could lead to erroneous clinical insights and treatment pathways. “This goes beyond bias from clues of race or gender,” said Brandon G. Hill, a machine learning scientist at DHMC and one of Schilling’s co-authors. “We found the algorithm could even learn to predict the year an X-ray was taken. It’s pernicious; when you prevent it from learning one of these elements, it will instead learn another it previously ignored. This danger can lead to some really dodgy claims, and researchers need to be aware of how readily this happens when using this technique.”
“The burden of proof just goes way up when it comes to using models for the discovery of new patterns in medicine,” Hill continued. “Part of the problem is our own bias. It is incredibly easy to fall into the trap of presuming that the model ‘sees’ the same way we do. In the end, it doesn’t. It is almost like dealing with an alien intelligence. You want to say the model is ‘cheating,’ but that anthropomorphizes the technology. It learned a way to solve the task given to it, but not necessarily how a person would. It doesn’t have logic or reasoning as we typically understand it.”
Source: Dartmouth Health
13.12.2024