News • New method
3D imaging to determine prostate cancer aggressiveness
A team led by the University of Washington has developed a new, non-destructive method that images entire 3D biopsies instead of a slice. The 3D images provided more information than a 2D image — specifically, details about the tree-like structure of the glands throughout the tissue.
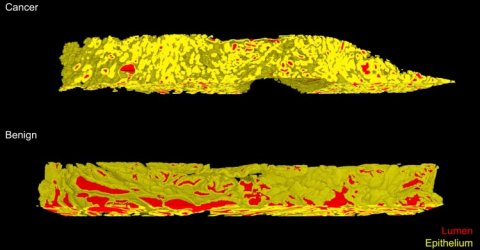
Prostate cancer is the most common cancer for men, and for men in the United States, it's the second leading cause of death. Some prostate cancers might be slow-growing and can be monitored over time, whereas others need to be treated right away. To determine how aggressive someone's cancer is, doctors look for abnormalities in slices of biopsied tissue on a slide. But this 2D method makes it hard to properly diagnose borderline cases.
Now a team led by the University of Washington has developed a new, non-destructive method that images entire 3D biopsies instead of just a slice. In a proof-of-principle experiment, the researchers imaged 300 3D biopsies taken from 50 patients—six biopsies per patient—and had a computer use 3D and 2D results to predict the likelihood that a patient had aggressive cancer. The 3D features made it easier for the computer to identify the cases that were more likely to recur within five years.
"We show for the first time that compared to traditional pathology—where a small fraction of each biopsy is examined in 2D on microscope slides—the ability to examine 100% of a biopsy in 3D is more informative and accurate," said senior author Jonathan Liu, a UW professor of mechanical engineering and of bioengineering. "This is exciting because it is the first of hopefully many clinical studies that will demonstrate the value of non-destructive 3D pathology for clinical decision-making, such as determining which patients require aggressive treatments or which subsets of patients would respond best to certain drugs."
The researchers used prostate specimens from patients who underwent surgery more than 10 years ago, so the team knew each patient's outcome and could use that information to train a computer to predict those outcomes. In this study, half of the samples contained a more aggressive cancer.
To create 3D samples, the researchers extracted "biopsy cores"—cylindrically shaped plugs of tissue—from surgically removed prostates and then stained the biopsy cores to mimic the typical staining used in the 2D method. Then the team imaged each entire biopsy core using an open-top light-sheet microscope, which uses a sheet of light to optically "slice" through and image a tissue sample without destroying it.
The 3D images provided more information than a 2D image—specifically, details about the complex tree-like structure of the glands throughout the tissue. These additional features increased the likelihood that the computer would correctly predict a cancer's aggressiveness.
The researchers used new AI methods, including deep-learning image transformation techniques, to help manage and interpret the large datasets this project generated.
"Over the past decade or so, our lab has focused primarily on building optical imaging devices, including microscopes, for various clinical applications. However, we started to encounter the next big challenge toward clinical adoption: How to manage and interpret the massive datasets that we were acquiring from patient specimens," Liu said. "This paper represents the first study in our lab to develop a novel computational pipeline to analyze our feature-rich datasets. As we continue to refine our imaging technologies and computational analysis methods, and as we perform larger clinical studies, we hope we can help transform the field of pathology to benefit many types of patients."
The team published these results in Cancer Research.
Source: University of Washington
10.12.2021