Article • The InnerEye Project
AI drives analysis of medical images
Some time in the distant future artificial intelligence (AI) systems may displace radiologists and many other medical specialists. However, in a far more realistic future AI tools will assist radiologists by performing very complex functions with medical imaging data that are impossible or unfeasible today, according to a presentation at the RSNA/AAPM Symposium during the Radiological Society of North America’s 2017 meeting.
Report: Cynthia E. Keen
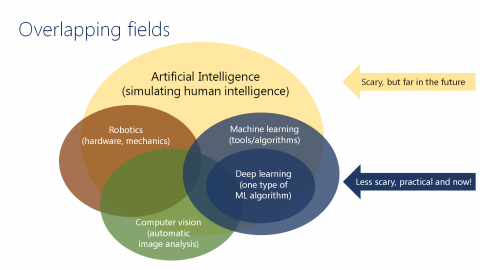
Research scientist Antonio Criminisi, and colleagues at Microsoft UK’s InnerEye project in Cambridge, have been developing machine-learning techniques for the automatic delineation of tumours and healthy anatomy for ten years. Intended applications are extraction of targeted radiomics measurements for quantitative radiology, rapid treatment planning for radiotherapy, and precise surgical planning and navigation. At the RSNA/AAPM Symposium he explained how the team developed algorithms for AI-drive analysis of medical images and their future use.
AI types to simulate human intelligence include robotics, computer vision, and machine learning. Computer vision utilises algorithms for automatic image analysis that understands semantics. Deep learning, just one type of practical machine learning, is part of life – e.g. analysing online buying and consumers’ interactions via computer.‘
AI research for medical image analysis originated with Microsoft Kinect, developed for game play, Criminisi explained. Kinect uses machine learning that takes input test depth images and, through pixel classification, determines body part segmentation to replicate 3-D human movement. ‘Eureka!’ The researchers decided that Kinect’s technology could help create 3-D images of CT, MRI, and PET scans. Thus InnerEye began a decade of research in automatic semantic segmentation of radiology images.
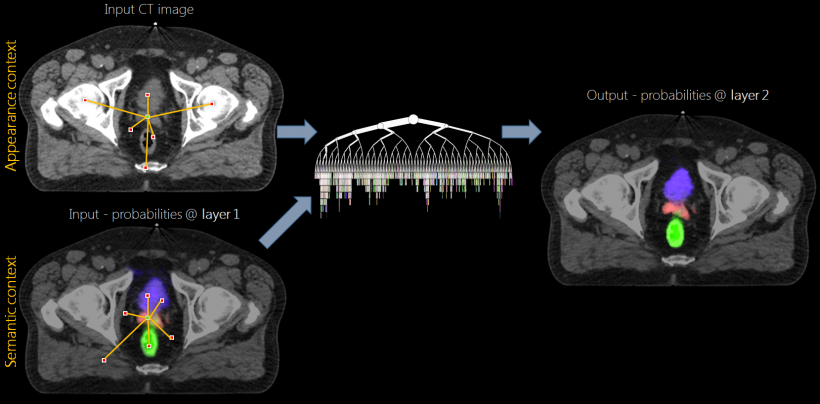
The goal of automatic 3-D segmentation? To build useful radiomics tools. Criminisi showed how data from two axial slices of the same CT scans overlaid could create 3-D images in seconds. ‘Voxel-wide semantic segmentation is difficult to achieve,’ he explained. ‘The sources of variability factors are huge. These include the same Hounsfield unit (HU) for different anatomies, large deformations, beam-hardening artefacts, different image resolutions, differing degrees of image noise, the presence/absence of contrast medium, and different patient preparations. All these need an algorithm, and the only way to deal with these large sources of variability consistently is through machine learning.’
Algorithms must work with everything, so training data must represent all these variables. For algorithm training, the researchers gathered image data from hundreds of patients in hospitals worldwide, produced by various modalities/models, with different acquisition protocols and image resolutions.
From this, a representation data set was created for segmentation, with each voxel and pixel in an image assigned to an anatomical structure. Supervoxels created from clusters of voxels were taught to associate voxels with anatomical structures. Hundreds of millions of voxels from data of hundreds of patients were used to create a ground truth model.
Decision tree techniques created forests of decision tree layers. A cascade of forest layers, each with unique probabilities, creates a trained deep decision forest model. ‘Designing a task-specific algorithm addresses a task at hand, but often doesn’t teach us how to address other tasks. When using a decision forest technique, for each new task the model remains the same, as do the training and runtime algorithms. We just enable new families of visual features.
Deep decision forests vs. cellular neural networks
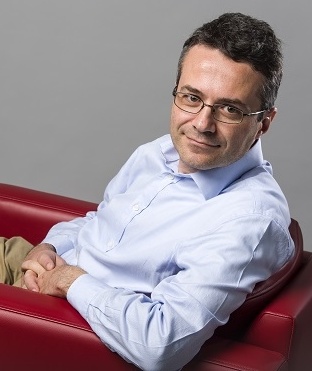
‘Once a deep decision forest model for semantic segmentation is developed, it’s then applied to previously unseen images to train it, with feedback applied to make improvements continuously. We use the word “deep” because this means we are reusing the output layer to create new layers, each of which improves upon the segmentation image being developed.’ At the event, Criminisi then demonstrated the cloud-based radiomics service.
Forests may be better for medical image analysis, so are preferred instead of cellular neural networks (CNN) for the semantic segmentation algorithms. Algorithmically they differ little, but Criminisi advised forests need less training data, may be faster, do not need GPUs, and may deal better with class imbalance.
‘Our algorithms have been extensively validated on diagnostic images of both boney structures and soft tissue. In accuracy, there has been no statistical difference between ours and expert radiologists. When compared with comparable regulatory agency-approved algorithms, our algorithms results were as good or better.’
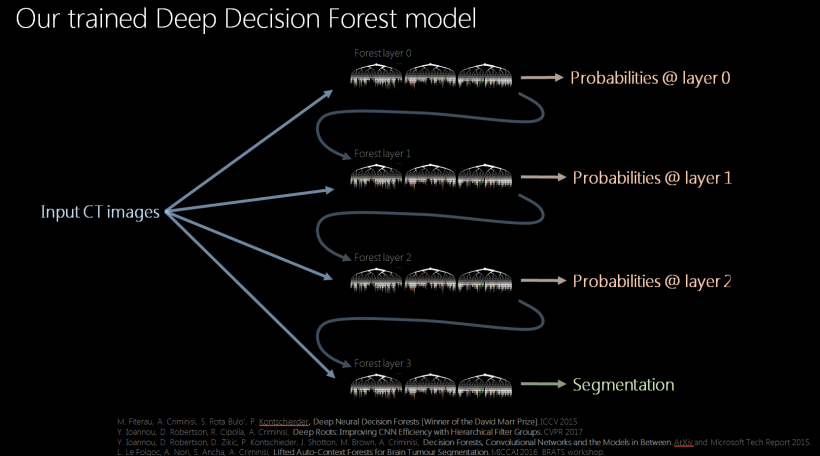
Assistive AI for radiotherapy planning
Currently, the clinical workflow for image-guided radiotherapy is first to acquire a planning scan, perform manual 3-D delineation and calculate the prescribed radiation dose. Manual 3-D delineation and dosimetry takes hours. The InnerEye process takes about five minutes. ‘We want to eliminate critical, laborious and tedious tasks for radiologists and dosimetrists,’ Criminisi explained.
Another application is to monitor disease progression during treatment and perform quantitative radiology automatically. The InnerEye model creates easier visualisation of a tumour from images acquired over time and can create plot lines showing volumes of an active tumour. Criminisi showed a brain tumour that disappeared during treatment – no tumour could be visualised, non-negligible oedema was detected.
‘AI can make monitoring cancer treatment effectiveness and disease progression much easier and potentially much more accurate,’ he said. ‘This is just one aspect of potentially being able to provide more efficient, quantitative image analysis workflow within a clinic. We want to turn this research into a real technology for clinical use,’ he concluded, inviting medical software providers into partnership. ‘Our expertise is in AI research, not healthcare. InnerEye cloud services are intended to be integrated components in third-party medical imaging software. We invite interested companies to contact us at innereyeinfo@microsoft.com.’
Profile:
Antonio Criminisi gained his PhD at the University of Oxford in 2000, the same year he joined Microsoft, where today he is a principal researcher at Microsoft Research in Cambridge, UK, working on artificial intelligence, machine learning, computer vision and medical image analysis. He has authored numerous scientific papers and won several awards, including the David Marr Best Paper Prize at the International Conference on Computer Vision 2015 in Chile. He now leads Microsoft’s InnerEye Project that uses AI to create medical image analysis tools.
27.02.2018