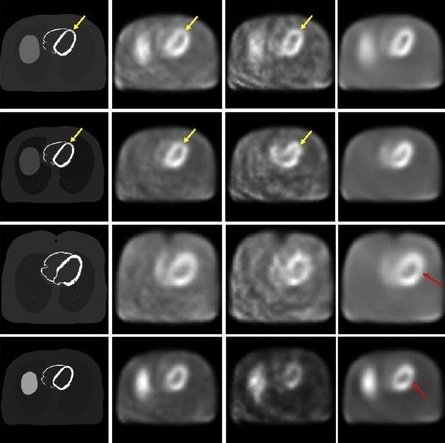
Image source: Washington University in St. Louis; image courtesy of Jha lab
News • Performance-based evaluation
Useful, not pretty: How AI can improve medical imaging
Medical imaging plays an essential role in diagnosis and treatment for an array of conditions. From X-rays to see a broken bone or a tooth cavity to SPECT scans for spotting heart defects, doctors use medical imaging to look inside the body, find disease and treat it appropriately. But what happens when those images aren’t clear?
Recent advances in artificial intelligence have opened the door to using AI-based methods for denoising, or cleaning up, medical images. However, before these tools can be used in clinical settings for real patient care, they need to be rigorously evaluated, said Abhinav Jha, assistant professor of biomedical engineering in the McKelvey School of Engineering and of radiology at Mallinckrodt Institute of Radiology (MIR) in the School of Medicine, both at Washington University in St. Louis.
Rather alarmingly, while the visual-similarity-based metrics suggested that the AI-based denoising technique improved performance, it was actually having no significant impact, and in some cases, it was even degrading performance on clinical tasks
Abhinav Jha
In a study published in Medical Physics, Jha and collaborators at MIR evaluated a commonly used AI-based approach to denoise cardiac SPECT images. The team assessed the performance of the approach in two ways: How visually similar were denoised images to normal images and how well did the denoised image perform in the clinically relevant task of detecting heart defects?
“Rather alarmingly, while the visual-similarity-based metrics suggested that the AI-based denoising technique improved performance, it was actually having no significant impact, and in some cases, it was even degrading performance on clinical tasks,” Jha said. “This emphasizes the important need for performing evaluation of AI algorithms on clinical tasks and not just relying on visual similarity as a measure of performance.”
In the study, first author Zitong Yu, a doctoral student in Jha’s lab, found that the AI denoising technique tended to smooth out cardiac SPECT images, which reduced noise as intended, but also reduced the contrast of the heart defect that doctors need to make accurate diagnoses. “This is precisely what we want to prevent from happening in actual medical practice,” Yu said.
Recommended article
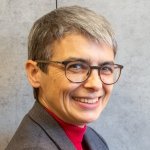
Article • Image augmentation, interpretation and evaluation
AI in multimodality hybrid imaging: a diamond in the rough
AI-based models for multimodality hybrid imaging have the potential to be a potent clinical tool but are currently held back by a lack of transparency and maturity, says Dr Irène Buvat, from the Laboratory of translational Imaging in Oncology, Institute Curie in Paris, France.
The study advocates for task-based evaluation of AI-based denoising methods to assess the usefulness of AI-processed images. “Ensuring AI-based denoising works well for real clinical tasks – not just aesthetically – would mean big benefits for patients by producing high-quality images in less time or with reduced radiation doses,” said collaborator Robert J. Gropler, professor of radiology and senior vice chair and division director of radiological sciences at MIR.
Jha and his team have been developing a new denoising technique along this direction, and their presentation on this topic received an honorable mention at the SPIE Medical Imaging meeting. Jha also led a multi-institutional, multi-agency team tasked with developing a framework for evaluating AI-based medical imaging methods. Their guidelines, Recommendations for Evaluation of AI for Nuclear Medicine (RELAINCE), were released in 2022 and informed this latest research.
Source: Washington University in St. Louis
20.06.2023