Article • Image augmentation, interpretation and evaluation
AI in multimodality hybrid imaging: a diamond in the rough
AI-based models for multimodality hybrid imaging have the potential to be a potent clinical tool but are currently held back by a lack of transparency and maturity, says Dr Irène Buvat, from the Laboratory of translational Imaging in Oncology, Institute Curie in Paris, France. During a webinar of the European Society of Radiology, the expert provided a roundup on the benefits and current limitations of the technology.
Article: Wolfgang Behrends
Image source: Adobe Stock/peterschreiber.media
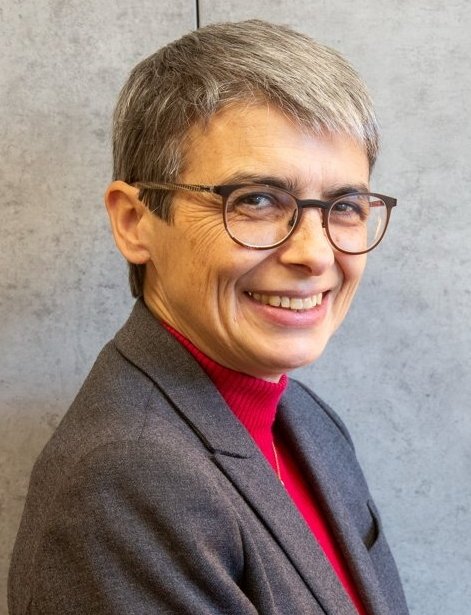
Image source: Institut Curie
‘AI keeps growing‘ – publications in both radiology and nuclear medicine have seen a steep incline in the past years. These mostly focus on three applications: enhancement of image quality, acceleration or automation of image analysis, and discovery of new insights, for example, using predictive biomarkers. The use of AI for image enhancement and analysis has even moved beyond purely academic methods, with the first generation of commercially available products entering the market.
The new technology shows great potential to accelerate research activity and lead to clinically relevant insights. However, Buvat advises to proceed with caution: ‘Having these AI methods available does not mean that they necessarily bring added clinical value.’ Still, the expert believes that the extensive clinical evaluation of these methods can serve as a foundation to build upon.
Image augmentation: better looking, but less accurate?
One field where AI has already produced promising results is the recovery of high-quality images from low-count (“noisy”) data, for example in PDG-PET imaging.1 ‘We can train the algorithm to optimally filter the data, so decent images can be recovered even from low-count acquisitions.’ In studies, nuclear medicine physician rated the resulting images as superior, compared with standard Gaussian filtering. However, it was noted that standard uptake values (SUV) were underestimated in AI-denoised images, especially in smaller lesions. Another study, which focused on low-count SPECT imaging, found that the AI filter even lowered lesion detectability, compared with unaltered images.2 ‘This is somewhat concerning,’ says Buvat, urging her colleagues to keep this effect in mind when measuring SUV during patient follow-up.
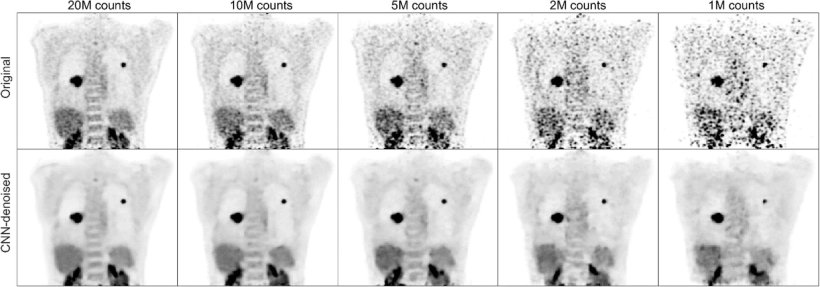
Image source: Schaefferkoetter et al., EJNMMI Research 2020 (CC BY 4.0)
AI is also used to reconstruct attenuation-corrected PET images without CT.3 At first glance, the AI-corrected images look almost identical to those generated with CT. ‘But again, we have to be quite careful when using these algorithms in clinical practice, because they may not always be reliable,’ the expert points out: A closer look reveals that the AI-corrected images are prone to missing smaller lesions, both on baseline and follow-up scans, which are picked up on in CT-based correction.
Despite these shortcomings, Buvat acknowledges the huge potential of AI: ‘If we can make these algorithms work, there would be substantial benefits: It would enable faster scanning and higher patient throughput, with less motion artifacts in the images. A second benefit would be in performing low-dose scanning, which can be extremely useful in paediatrics, or for the follow-up of patients using PET/SPECT scans. There could even be novel applications, a larger use of nuclear medicine procedures in children and also possibly, in pregnant women.’ For example, researchers used FDG-PET/MR imaging to assess fetal radiation dose in the uterus at various stages of a pregnancy.4
Image segmentation: (almost) on par with human experts
AI has also proved capable in the field of image interpretation, for example for automated segmentation. ‘The good news here is that out-of-the-box solutions actually work quite well,’ Buvat points out, citing as an example nnU-Net, a deep learning-based, self-configuring segmentation algorithm.5 The relevancy in the context of hybrid imaging becomes clear when the method is applied to cancer patients, where the AI will automatically segment lesions, for example in head and neck cancer.6 Current algorithms produce tumour delineations that range from satisfactory to almost indistinguishable from those of human experts – a promising application, the expert finds, even if some manual correction of results may still be necessary at this point.
Extending this method to whole-body imaging, recent algorithms have even tackled the challenge of multi-organ segmentation from PET/CT/MR scans – with equally impressive results.7 This could lead to several interesting applications, from automated tumour location and reporting to multi-organ metabolism measurements. ‘We are entering the domain of systems medicine here, so this is extremely promising.’ However, Buvat cautions that the AI-generated evaluation should still be closely scrutinised to avoid misleading results.
Gaining additional insights
Artificial intelligence can also be used to gather additional insights from diagnostic images. The creation of radiomic model for prediction and patient stratification is among the most frequent of these applications, the expert explains. For example, researchers used an AI to predict survival of Diffuse Large B-cell lymphoma (DLBCL) patients based on their PET/CT scan maximum intensity projection (MIP).8 ‘When the scan is segmented by an AI, and the metabolic tumour volume is calculated, the stratification – which is fully automated – is very close to what is obtained by an expert.’
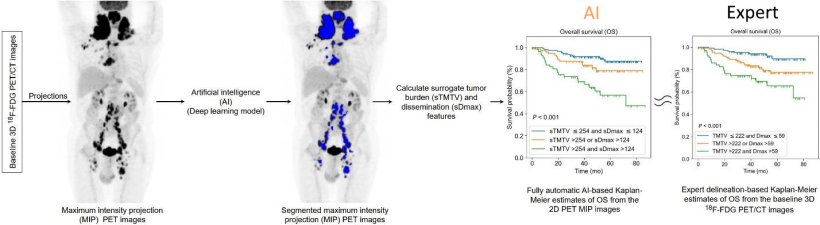
Image source: Girum et al., Journal of Nuclear Medicine 2022 (CC BY 4.0)
Combining radiomic and clinical information can also yield valuable insights into patient survival and recurrence rates from head and neck cancer.6 ‘A nice asset of this model is that you can understand the factors that are related to recurrence-free survival,’ Buvat says: This takes into account both clinical factors, such as tobacco use, and imaging features, for example maximum diameter of lesions and shows their impact, giving transparent results.
A major challenge at this point is the ability of the models to generalise. ‘It has been shown that this is far from obvious,’ the expert points out. Often, the models’ performance was significantly reduced when confronted with new, external data.9 A potential solution for this issue is already in the wings, through harmonising the images and features used by the algorithm. Recent papers show that this approach is quite successful, achieving adequate results even with new data.10,11
An assistant to trust in
when the readers are given the results of the AI and the explanation, the performance in detection is improved compared to when the readers are not assisted by the AI
Irène Buvat
Another roadblock for the adaptation of AI models lies within their lack of transparency. While calculation formula may be highly accurate, their cryptic appearance prevents the imaging experts from gaining an actual understanding about their working principle.12 ‘When dealing with such models, it is impossible to understand what it means, and that means it is difficult to trust it, since we have no idea about what is going on. What we need are models that provide an explanation associated with the results.’ For example, insights into the models’ “black box” may be gained via attention and probability maps that show which region of a given image was used in the decision-making process.13 ‘It has been shown that, when the readers are given the results of the AI and the explanation, the performance in detection is improved compared to when the readers are not assisted by the AI.’
Buvat stresses that even with sophisticated algorithms at work, sometimes an image just does not hold sufficient information to answer a clinical question, for example to differentiate between different forms of neurodegenerative disease.14 Such limitations may be overcome using multimodal data integration. ‘We have to account for more than just the hybrid imaging data,’ the expert points out, citing clinical, pathological and genetic data as viable sources to complement imaging results. AI will play a crucial role in this task, she says: ‘But, at this point, the AI-based methods have not yet reached sufficient maturity.’ Clinical-task-based and multi-centre evaluation are still needed to thoroughly evaluate the models. If these issues are addressed, Buvat predicts a bright future for the technology, concluding: ‘AI will ultimately make high-quality quantitative image interpretation commonplace and will help us discover new radiomic phenotypes associated with outcome.’
Profile:
Dr Irène Buvat is Research Director of the French National Centre for Scientific Research (CNRS) and heads the Inserm Laboratory of Translational Imaging in Oncology (LITO) at Institut Curie, Orsay, France. She is an expert in the development of quantification methods in molecular imaging using Emission Tomography. Her research focuses on developing and validating new biomarkers in Positron Emission Tomography, including using Artificial Intelligence methods, for precision medicine.
References:
- Schaefferkoetter et al.: Convolutional neural networks for improving image quality with noisy PET data; EJNMMI Research 2020
- Yu et al.: Investigating the limited performance of a deep-learning-based SPECT denoising approach: an observer-study-based characterization; Proceedings of SPIE Medical Imaging 2022
- Yang et al.: CT-less Direct Correction of Attenuation and Scatter in the Image Space Using Deep Learning for Whole-Body FDG PET: Potential Benefits and Pitfalls; Radiology: Artificial Intelligence 2020
- Zanotti-Fregonara et al.: Fetal Radiation Dose from 18F-FDG in Pregnant Patients Imaged with PET, PET/CT, and PET/MR; Journal of Nuclear Medicine 2015
- Isensee et al.: nnU-Net: a self-configuring method for deep learning-based biomedical image segmentation; Nature Methods 2021
- Rebaud et al.: Simplicity is All You Need: Out-of-the-Box nnUNet followed by Binary-Weighted Radiomic Model for Segmentation and Outcome Prediction in Head and Neck PET/CT; MICCAI 2022
- Sundar et al.: Fully Automated, Semantic Segmentation of Whole-Body 18F-FDG PET/CT Images Based on Data-Centric Artificial Intelligence; Journal of Nuclear Medicine 2022
- Girum et al.: 18F-FDG PET maximum intensity projections and artificial intelligence: a win-win combination to easily measure prognostic biomarkers in DLBCL patients; Journal of Nuclear Medicine 2022
- Yu et al.: External Validation of Deep Learning Algorithms for Radiologic Diagnosis: A Systematic Review; Radiology: Artificial Intelligence 2022
- Orlhac et al.: A guide to ComBat harmonization of imaging biomarkers in multicenter studies; Journal of Nuclear Medicine 2021
- Leithner et al.: Impact of ComBat Harmonization on PET Radiomics-Based Tissue Classification: A Dual-Center PET/MRI and PET/CT Study; Journal of Nuclear Medicine 2022
- Huang et al.: Development and Validation of a Radiomics Nomogram for Preoperative Prediction of Lymph Node Metastasis in Colorectal Cancer; Journal of Clinical Oncology 2016
- Miller et al.: Explainable Deep Learning Improves Physician Interpretation of Myocardial Perfusion Imaging; Journal of Nuclear Medicine 2022
- Varoquaux G, Cheplygina V: Machine learning for medical imaging: methodological failures and recommendations for the future; NPJ Digital Medicine 2022
19.12.2022