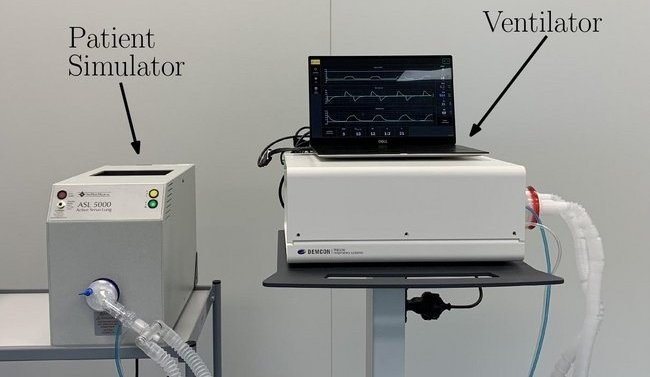
Image source: Eindhoven University of Technology (TU/e)
News • Smart breathing support
Self-learning ventilators could save more COVID-19 patients
As the corona pandemic continues, mechanical ventilators are vital for the survival of COVID-19 patients who cannot breathe on their own. One of the major challenges is tracking and controlling the pressure of the ventilators, to ensure patients get exactly the amount of air they need.
Researchers at the Eindhoven University of Technology (TU/e) have developed a technique based on self-learning algorithms that improves the performance of the controller by a factor ten. The results were presented at IFAC2020, a major international conference on automatic control (available through arXiv.org).
A mechanical ventilator pumps air in and out of a patient’s lungs, when they are no longer able to breathe (sufficiently) on their own. The alternating flow of air allows the lungs to exchange CO2 for O2 in the blood, therefore ensuring the patient’s survival. To make sure that the patients get the amount of air they need, it is crucial that the air pressure exactly follows the instructions of the doctors. Failure to do so, could result in higher mortality. This is not a trivial problem. Not every patient is the same, and the hose and blower systems used to get the air into the patient may vary, leading to unwanted inconsistencies. Much research has therefore been done to rectify this problem, using techniques like adaptive feedback control. However, these techniques rely on accurate patient models, which in practice are not always available, because not every patient is the same.
The researchers at TU/e employ an alternative control technique that associate professor Tom Oomen of the Department of Mechanical Engineering is developing for applications in the high-tech industry, such as printers and wafer scanners. This technique is based on self-learning algorithms and exploits the fact that breathing in sedated patients (which many COVID-19 patients are) tends to be very regular, just like many processes in industry.
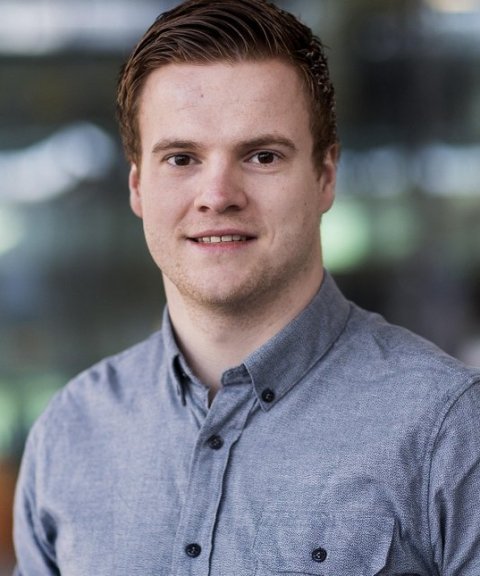
Image source: Eindhoven University of Technology (TU/e)
The technique, called Repetitive Control, can learn from machine errors, and has the ability to correct them within a few iterations, using measured data from sensors in the machine. Doing so for a mechanical ventilator, it can increase the accuracy of pressure and flow provided by the ventilator by a factor ten after a few breaths, even when the lung capacity of the patient is not known.
The technique was tested on artificial lungs in a lab. In all three scenarios (a baby, a child and an adult), the performance of the pressure tracking was superior to existing devices. “Thanks to the self-learning algorithm we apply, we are able to achieve very accurate pressure levels, regardless of the patient connected to the device. This makes the treatment much more constant”, says Joey Reinders, PhD candidate in the Dynamics and Control section at the department of Mechanical Engineering and one of the researchers involved.
Reinders and his colleagues did most of their research in 2019, when for many people the corona pandemic was still a dystopian fantasy. “When we started our research we had no idea that it would become so relevant,” he says. “I am therefore very happy with the results, which one day could prove a lifesaver for corona patients.” He points out that more research needs to be done before the technique can be used in practice. Reinders and his colleagues only tested on sedated patients, for whom Repetitive Control works best because their breathing patterns are so regular. However, ventilators are also used on patients who are still conscious, and who may start to breathe unexpectedly. The ventilator controller needs to be able to cope with such situations as well.
Reinders will continue his PhD research, which is supervised by Oomen and professor Nathan van de Wouw, tackling broader problems in mechanical ventilation systems, such as detection of patient-ventilator asynchrony and identification of the patient’s lung parameters, such as stiffness and airway resistance. He will also be working closely with his employer, DEMCON Macawi Respiratory Systems, to improve ventilation systems in Intensive Care Units, for which he has already been in contact with Erasmus MC in Rotterdam.
Source: Eindhoven University of Technology (TU/e)
03.08.2020