© Anna – stock.adobe.com (AI generated image)
Article • Artificial intelligence meets internal medicine
Medical AI: Enter ‘dea ex machina’
In the world of theatre, the ‘deus ex machina’, the god from the machine, is a dramaturgical trick to resolve seemingly unsolvable conflicts. Can artificial intelligence (AI) also be such a universal problem solver for internal medicine? At the Annual Congress of the German Society of Internal Medicine (DGIM) in Wiesbaden, Dr Isabella Wiest explored the potential – and limitations – of AI helpers.
Article: Wolfgang Behrends
The research associate at the Else Kröner Fresenius Centre (EKFZ) for Digital Health at TU Dresden and resident physician at Mannheim University Hospital explained that the aspect of generative AI in particular shows enormous potential.
Every person generates a large quantity of health-related data on their way through doctors' surgeries and clinics, but also in their private lives, and this data could be analysed by an AI for clinically relevant patterns, the expert pointed out. Examples include medical reports, radiology images, histopathology scans as well as genome and sensor data.
However, the availability of this wealth of data is only half the battle, Wiest pointed out: around 80% of data is stored in unstructured form1, and the free text of findings often contains relevant additional information that goes beyond the structured data.2 In order to use this information quantitatively, much manual rework has been necessary up to now. Traditionally, tasks such as transferring the data from doctors' letters into a table have fallen to the doctoral students.
Locally-run language models help protect sensitive data
This tedious and unrewarding work could be delegated to a generative AI, explained the expert, referring to what is currently probably the best-known application: The large language model (LLM) ChatGPT from OpenAI. This program has been trained on large text corpora and is able to generate new content on this basis. This also includes extracting information from unstructured texts. One recent study has demonstrated useful results in retrieving TNM stages from pathological reports – even if these had not been digitised.3
However, the OpenAI solution cannot be used in these cases, as the sensitive patient data should never be transferred to the US company's servers, Wiest pointed out. A viable alternative would be locally-run language models such as Llama-2, which can be installed on a computer on site. ‘This means that the data stays where it is generated and does not leave the hospital or practice.’ Despite having far less computing power, the local AI model delivered good results in tests with gastroenterological endoscopy findings, the expert reported.
[Retrieval-Augmented Generation] is a promising technology that doctors can use to have up-to-date treatment recommendations available when they are needed
Isabella Wiest
Initial studies suggest that the LLMs also perform well when summarising the content of clinical texts. Although these summaries are by no means flawless, Wiest recognised that the AI-generated texts contain fewer misinterpretations and inaccuracies than those of human experts.4 The expert argued that the use of these tools could ‘make work easier and increase efficiency, leaving more time for the patient and less time for documentation’.5
However, for LLMs to provide medically accurate information without producing the infamous “hallucinations”, the input has to be right, Wiest pointed out: for example, current guidelines would have to be entered into a database in the form of machine-readable vectors so that the corresponding prompt results in well-founded answers. This approach, known as ‘Retrieval Augmented Generation’ (RAG), has already produced some good results in initial trials, according to the expert.6 ‘This is a promising technology that doctors can use to have up-to-date treatment recommendations available when they are needed.’
One algorithm for all – or all for one?
The increasing digitalisation of health data opens up numerous other potential applications for AI, Wiest explained: From predicting MSI to detect genetic changes in histopathology7,8,9, through organ segmentation to support surgical treatment planning10 to assessing radiological images.11,12 However, she emphasised that irrespective of the specialist area, it is fundamentally important to have a solid data basis and to validate the trained models thoroughly. ‘We need to scrutinise generative AI very critically and develop good metrics.’ She concluded that the transparency of the models and explainability of the results must always be ensured.
As an alternative to the current models, which are tailored to narrowly defined tasks, more recent research is moving in the direction of a “generalist” AI. This is designed to integrate any data modalities from the clinic and practice into an application and thus enable a holistic view of medical issues13 – such a model might at least come close to the concept of the deus ex machina.
Recommended article
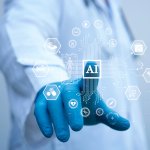
News • GMAI for cancer treatment
Generalist Medical AI: Experts propose legal guardrails
Generalist Medical Artificial Intelligence (GMAI) models pose a significant challenge to current regulatory frameworks. Experts discuss how regulation of these models could work in the future.
‘Whether such a model will exist at all remains to be seen,’ said the expert, pointing out the high level of complexity required. Instead of having a single “jack-of-all-trades” AI, she suggested that individual, specialised models might also be orchestrated with the help of a parent AI – a so-called LLM agent.14 Depending on the clinical task, this supervising algorithm would “consult” the appropriate AI in order to generate a diagnostic or treatment recommendation based on its expertise.
The final hurdle that Wiest addressed was the integration of the models into existing healthcare infrastructures. ‘Interoperability of digital systems is urgently needed for this.’ This means there are still a few challenges to overcome before AI can make the leap from digital assistant to a fully-fledged clinical dea ex machina.
References:
- Kong HJ: Managing Unstructured Big Data in Healthcare System; Healthcare Informatics Research 2019
- Price SJ et al.: Is omission of free text records a possible source of data loss and bias in Clinical Practice Research Datalink studies? A case–control study; BMJ Open 2016
- Truhn D et al.: Extracting structured information from unstructured histopathology reports using generative pre-trained transformer 4 (GPT-4); The Journal of Pathology 2023
- Van Veen D et al.: Adapted large language models can outperform medical experts in clinical text summarization; Nature Medicine 2024
- Sinsky C et al.: Allocation of Physician Time in Ambulatory Practice: A Time and Motion Study in 4 Specialties; Annals of Internal Medicine 2016
- Ferber D et al.: Large Language Models for Information Retrieval and Comparison of Medical Oncology Guidelines; Accepted for publication; NEJM AI
- Coudray N et al.: Classification and mutation prediction from non–small cell lung cancer histopathology images using deep learning; Nature Medicine 2018
- Naik N et al.: Deep learning-enabled breast cancer hormonal receptor status determination from base-level H&E stains; Nature Communications 2020
- Kather JN et al.: Deep learning can predict microsatellite instability directly from histology in gastrointestinal cancer; Nature Medicine 2019
- Wasserthal J et al.: TotalSegmentator: Robust Segmentation of 104 Anatomic Structures in CT Images; Radiology: Artificial Intelligence 2023
- Wanders AJT et al.: Interval Cancer Detection Using a Neural Network and Breast Density in Women with Negative Screening Mammograms; Radiology 2022
- Kim HE et al.: Changes in cancer detection and false-positive recall in mammography using artificial intelligence: a retrospective, multireader study; Lancet Digital Health 2020
- Moor M et al.: Foundation models for generalist medical artificial intelligence; Nature 2023
- Ferber D et al.: Autonomous Artificial Intelligence Agents for Clinical Decision Making in Oncology; arXiv 2024
03.12.2024