Article • Cardiology & AI
Machine learning to predict sudden cardiac death
Could machine learning (ML) help to predict sudden cardiac death (SCD)? According to Dr Sanjiv Narayan, Professor of Medicine at Stanford University, California, many exciting studies are using ML to predict sudden death in ways not previously possible.
Report: Mark Nicholls
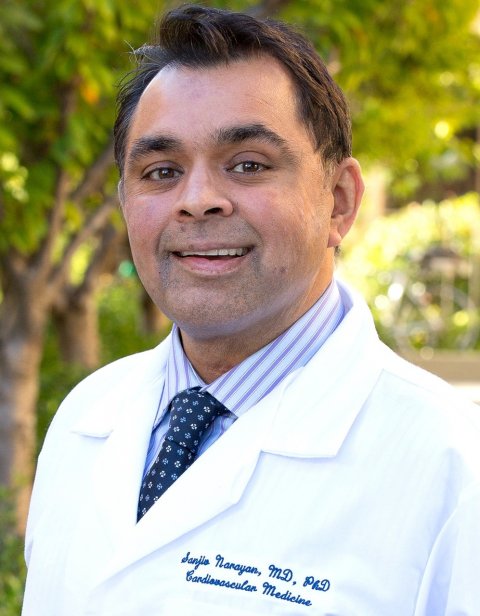
‘Complex data, such as MRI geometry, very large electronic health records or continuous data streams from wearables, are difficult to probe meaningfully with statistics, yet can be studied by ML to make predictions.’ Narayan, who directs the university’s Computational Arrhythmia Laboratory, and co-directs the Stanford Arrhythmia Center, will question ‘Sudden cardiac death: Can we move from prediction to prevention?’ during his ESC 2021 presentation this August.
Speaking to Healthcare in Europe, the professor said ML applications can now use simple measures of heart and breathing rates to detect imminent collapse or hypotension within minutes. This could become the basis for novel wearables to predict SCD a few minutes before it happens and alert emergency services, hopefully to prevent the event. ‘This would be revolutionary,’ he continued. ‘In other applications, machine learning has been used to extract features from the detailed structure of the ventricles and granular distributions of scar imaged by Gadolinium-enhanced MRI to predict SCD better than existing clinical tools.’
Other studies have used ML to predict which drugs could be pro-arrhythmic from their molecular structure alone, or whether ML of the ECG alone could improve on current risk models, while his group has shown that signals from inside the heart that indicate cellular remodelling in heart failure can predict SCD.
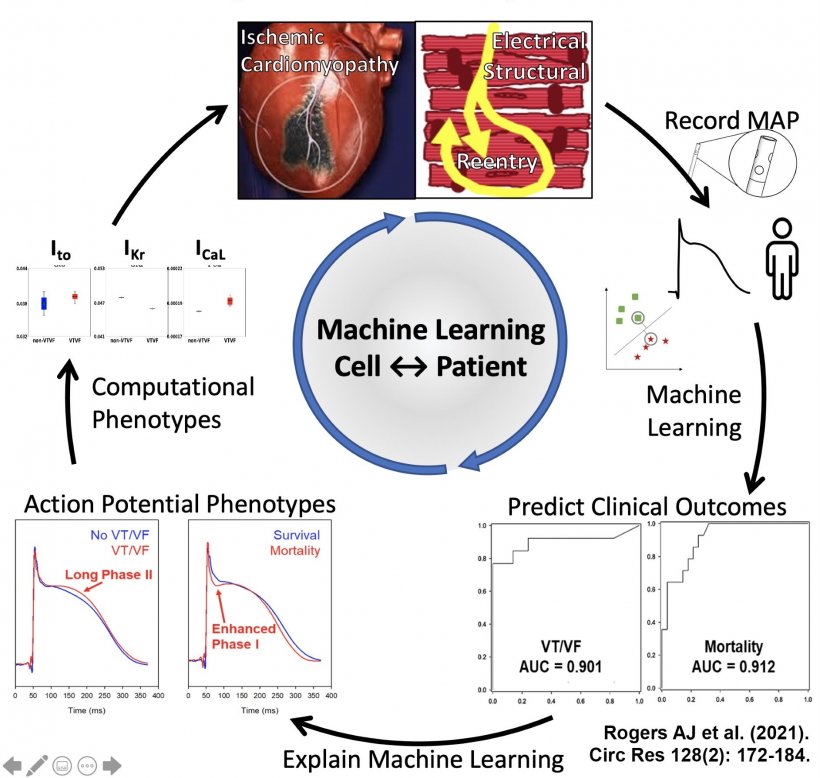
To enable personalised medicine, ML can be trained to learn patterns in an individual, rather than in a population, Narayan explained. Examples: Do LV (left ventricular) geometry, scar on MRI, or electrical remodelling indicated by action potential shape (see figure above), predict sudden death? ‘These questions are difficult to frame for conventional statistical analysis, which is better suited to linear data than complex data (of high dimensionality). ML can also be used for very large data – such as continuous streams from wearables,’ Narayan added.
This can empower the patient, reduce delays in the healthcare system, and potentially off-load some of the health system burden
Sanjiv Narayan
Whilst the professor points out that ML will never replace the clinician, it will provide better tools for personalised medicine – an evolving trend for over a decade. ‘MRI and CT scans have ML tools to help the clinician interpret them, or that flag potential abnormalities,’ he pointed out. ‘Even ECG machines have forms of ML to provide the automated diagnoses. This will enable the clinician to make better management decisions and be more efficient.’
Patients also win where ML automates tools, e.g. focused analysis of continuous large data from an Apple watch, activity monitors or smart devices in the bathroom, elsewhere at home or even in a care home. ‘This can empower the patient, reduce delays in the healthcare system, and potentially off-load some of the health system burden,’ Narayan pointed out. ML can provide simple automated tips; patients can benefit from better and safer drug prescribing and, he believes, many other benefits have yet to be realised.
Other presentations in the session will examine digital heart rate analysis and question whether we can predict sudden cardiac death as well as prevent sudden cardiac death in heart failure.
*ESC 2021, Sudden cardiac death: can we move from prediction to prevention? August 27, 8.30am (CEST).
Profile:
Sanjiv Narayan is Professor of Medicine at Stanford University, Director of the Computational Arrhythmia Laboratory, and Co-Director of the Stanford Arrhythmia Center. He oversees and directs several NIH-funded studies to develop machine learning and computer models for arrhythmias, to bring them directly to the care of patients.
27.08.2021