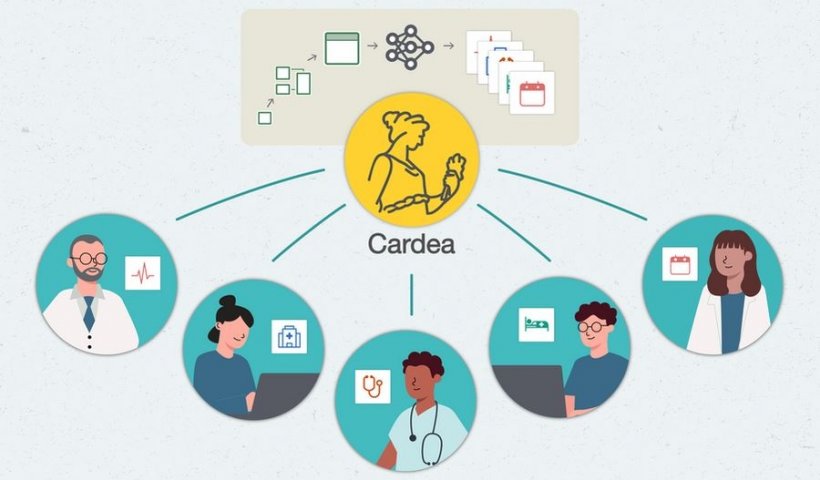
Image: Arash Akhgari/MIT
News • Shepherding medical data
Machine learning platform turns healthcare data into insights
Over the past decade, hospitals and other healthcare providers have put massive amounts of time and energy into adopting electronic healthcare records, turning hastily scribbled doctors' notes into durable sources of information. But collecting these data is less than half the battle.
It can take even more time and effort to turn these records into actual insights — ones that use the learnings of the past to inform future decisions. Cardea, a software system built by researchers and software engineers at the Data to AI Lab (DAI Lab) at the Massachusetts Institute of Technology (MIT), is built to help with that. By shepherding hospital data through an ever-increasing set of machine learning models, the system could assist hospitals in planning for events as large as global pandemics and as small as no-show appointments.
With Cardea, hospitals may eventually be able to solve "hundreds of different types of machine learning problems," says Kalyan Veeramanchaneni, principal investigator of the DAI Lab and a principal research scientist in MIT's Laboratory for Information and Decision Systems (LIDS). Because the framework is open-source, and uses generalizable techniques, they can also share these solutions with each other, increasing transparency and enabling teamwork.
Cardea belongs to a field called automated machine learning, or AutoML. Machine learning is increasingly common, used for everything from drug development to credit card fraud detection. The goal of AutoML is to democratize these predictive tools, making it easier for people — including, eventually, non-experts — to build, use, and understand them, says Veeramachaneni. Instead of requiring people to design and code an entire machine learning model, AutoML systems like Cardea surface existing ones, along with explanations of what they do and how they work. Users can then mix and match modules to accomplish their goals, like going to a buffet rather than cooking a meal from scratch. For instance, data scientists have built a number of machine learning tools for health care, but most of them aren't very accessible — even to experts. "They're written up in papers and hidden away," says Sarah Alnegheimish, a graduate student in LIDS. To build Cardea, she and her colleagues have been unearthing these tools and bringing them together, aiming to form "a powerful reference" for hospital problem-solvers, she says.
Recommended article
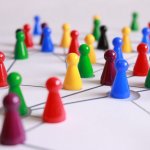
News • Information distribution
Experts urge solution to health data transfer barriers
Legal challenges hamper the sharing of health data with researchers outside the EU/European Economic Area (EEA), a new report by European academy networks concludes. The authors call for solutions to overcome these barriers to ensure timely and straightforward research collaboration in the public sector and thereby maximize health benefits for European citizens.
To turn reams of data into useful predictions, Cardea walks users through a pipeline, with choices and safeguards at each step. They are first greeted by a data assembler, which ingests the information they provide. Cardea is built to work with Fast Healthcare Interoperability Resources (FHIR), the current industry standard for electronic health care records. Hospitals vary in exactly how they use FHIR, so Cardea has been built to "adapt to different conditions and different datasets seamlessly," says Veeramachaneni. If there are discrepancies within the data, Cardea's data auditor points them out, so that they can be fixed or dismissed.
Next, Cardea asks the user what they want to find out. Perhaps they would like to estimate how long a patient might stay in the hospital. Even seemingly small questions like this one are crucial when it comes to day-to-day hospital operations — especially now, as health care facilities manage their resources during the Covid-19 pandemic, says Alnegheimish. Users can choose between different models, and the software system then uses the dataset and models to learn patterns from previous patients, and to predict what could happen in this case, helping stakeholders plan ahead.
Currently, Cardea is set up to help with four types of resource-allocation questions. But because the pipeline incorporates so many different models, it can be easily adapted to other scenarios that might arise. As Cardea grows, the goal is for stakeholders to eventually be able to use it to "solve any prediction problem within the health care domain," Alnegheimish says.
The team presented their paper describing the system at the IEEE International Conference on Data Science and Advanced Analytics in October 2020. The researchers tested the accuracy of the system against users of a popular data science platform, and found that it out-competed 90 percent of them. They also tested its efficacy, asking data analysts to use Cardea to make predictions on a demo health care dataset. They found that Cardea significantly improved their efficiency — for example, feature engineering, which the analysts said usually takes them an average of two hours, took them five minutes instead.
The hope is for people to adopt it, and start contributing to it. With the help of the community, we can make it something much more powerful
Sarah Alnegheimish
Hospital workers are often tasked with making high-stakes, critical decisions. It's vital that they trust any tools they use along the way, including Cardea. It's not enough for users to plug in some numbers, press a button, and get an answer: "They should get some sense of the model, and they should know what is going on," says Dongyu Liu, a postdoc in LIDS. To build in even more transparency, Cardea's next step is a model audit. Like all predictive apparatuses, machine learning models have strengths and weaknesses. By laying these out, Cardea gives the user the ability to decide whether to accept this model's results, or to start again with a new one.
Cardea was released to the public earlier this year. Because it's open source, users are welcome to integrate their own tools. The team also took pains to ensure that the software system is not only available, but understandable and easy to use. This will also help with reproducibility, Veeramachaneni says, so that predictions made on models built with the software can be understood and checked by others. The team also plans to build in more data visualizers and explanations, to provide an even deeper view, and make the software system more accessible to non-experts, Liu says. "The hope is for people to adopt it, and start contributing to it," Alnegheimish says. "With the help of the community, we can make it something much more powerful."
Source: Massachusetts Institute of Technology (MIT)
13.04.2021